Evaluating music mastering quality using machine learning.
CASCON(2018)
摘要
Machine learning has been applied in a vast array of applications in the recent years, including several qualitative problems in the arts. However, in the world of music production, including mixing and mastering, most tasks are still performed by music professionals with decades of experience. Aspiring mastering engineers typically have to apprentice with professionals to learn their craft. Access to professionals is a scarce resource though, as they are typically very busy. In this paper, we present a method to evaluate the mastering quality of a piece of music automatically. We delegate the task of determining what we deem to be a subjectively well mastered song to professional mastering engineers. Using professionally mastered music, we derive datasets with varying degrees of deviation from the original music and train models to recognize the changes that have been made. This allows us to provide novice mastering engineers with an automatic rating of their work based on the magnitude of the deviation from the gold standard. We present experiments that demonstrate the accuracy of our approach, as well as a user study that shows how the results of our approach correlate to assessments made by human evaluators.
更多查看译文
AI 理解论文
溯源树
样例
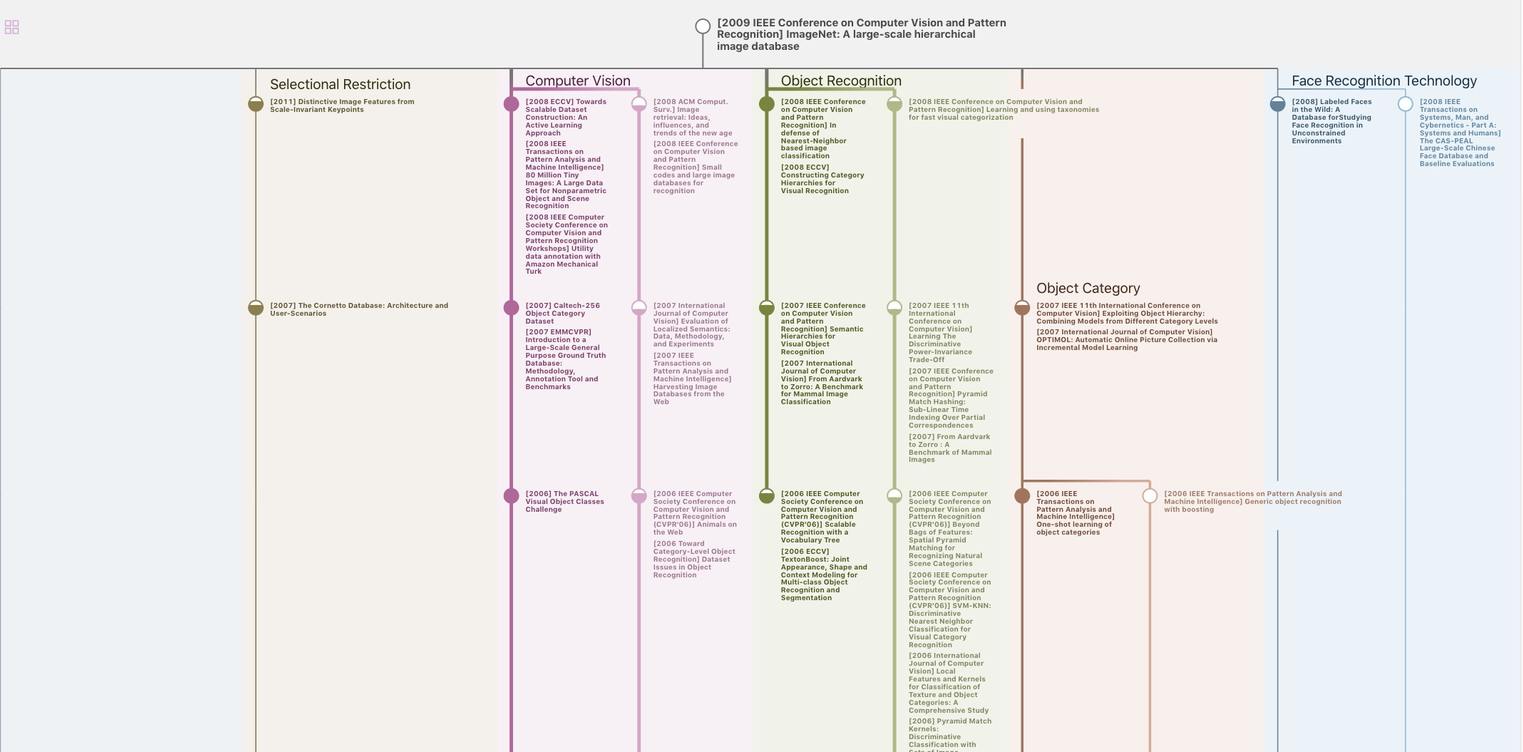
生成溯源树,研究论文发展脉络
Chat Paper
正在生成论文摘要