Recommendation with Hybrid Interest Model
2018 18TH IEEE INTERNATIONAL CONFERENCE ON DATA MINING WORKSHOPS (ICDMW)(2018)
摘要
Considerable efforts have been made to build high quality recommender system in the last two decades. Among them, factor models, which represent the user's preferences by decomposing the sparse user-item interactions matrix into two low-rank latent feature matrices as user profiles and item profiles, are one of the most prevalent recommendation algorithms due to its simplicity and scalability. While the factor models successfully capture the user's static interest, they fail to consider the user's dynamic interest. Recently, recurrent neural network (RNN) based models, which capture temporal dynamics for users, has attracted significant attention and achieved promising results. Considering that a user's choice could due to either the static interest or dynamic interest, we here propose a novel recommender with hybrid interest model (HIM) to simultaneously model users' static interest and dynamic interest, where the dynamic interest is captured with an attention layer based on users' activities in the current session. An adaptive mechanism is introduced to determine the contribution of the two types of user's interest when making recommendation. What's more, to handle large number of users and items in the real world scenario, we design an efficient model with shallow network. We validate the HIM with three real-world datasets and the experimental results have demonstrated the superiority of our model over state-of-the-art methods.
更多查看译文
关键词
recommender systems (RS), static interest, dynamic interest, adaptive mechanism, shallow network
AI 理解论文
溯源树
样例
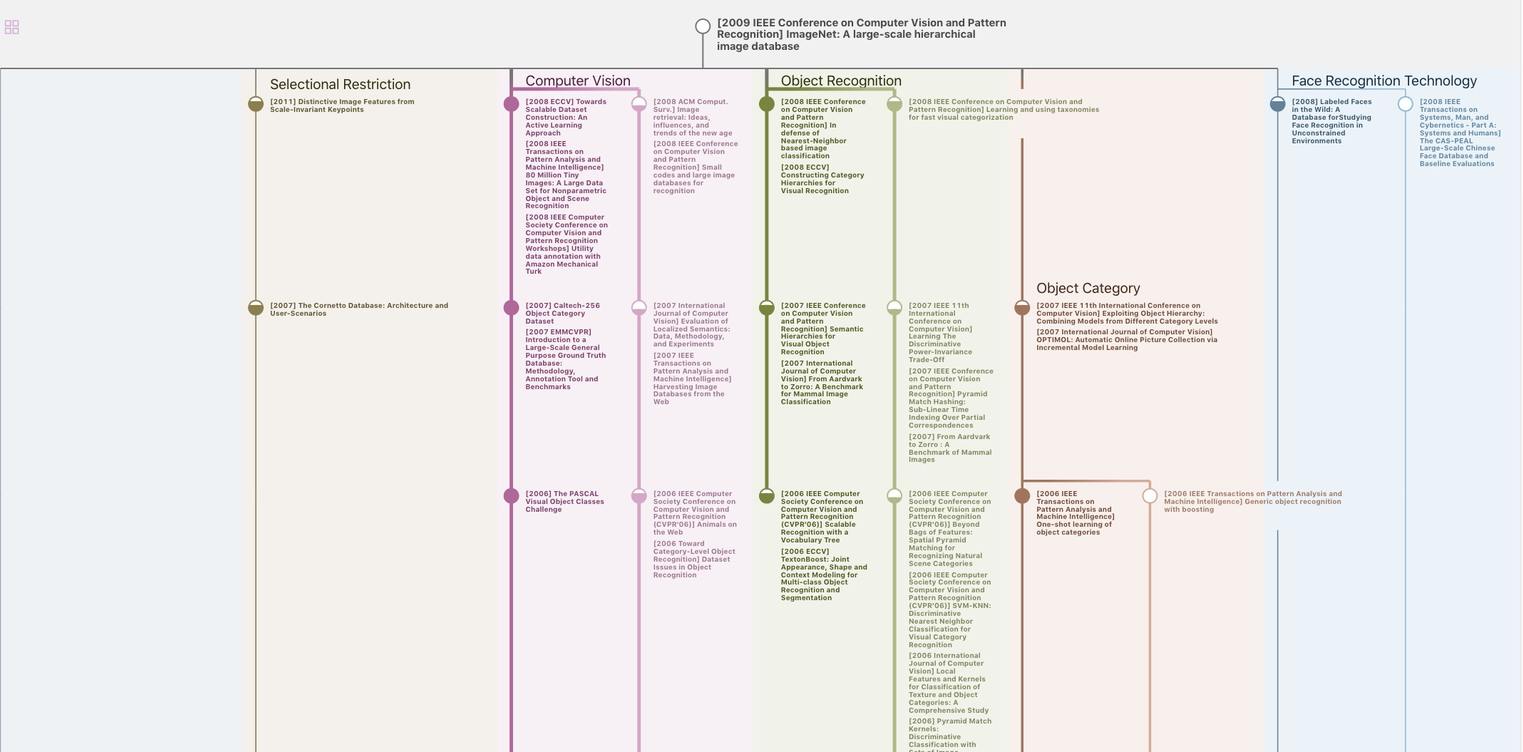
生成溯源树,研究论文发展脉络
Chat Paper
正在生成论文摘要