Robust Commuter Movement Inference from Connected Mobile Devices.
ICDM Workshops(2019)
摘要
The preponderance of connected devices provides unprecedented opportunities for fine-grained monitoring of the public infrastructure. However while classical models expect high quality application-specific data streams, the promise of the Internet of Things (IoT) is that of an abundance of disparate and noisy datasets from connected devices. In this context, we consider the problem of estimation of the level of service of a city-wide public transport network. We first propose a robust unsupervised model for train movement inference from wifi traces, via the application of robust clustering methods to a one dimensional spatio-temporal setting. We then explore the extent to which the demand-supply gap can be estimated from connected devices. We propose a classification model of real-time commuter patterns, including both a batch training phase and an online learning component. We describe our deployment architecture and assess our system accuracy on a large-scale anonymized dataset comprising more than 10 billion records.
更多查看译文
关键词
Sensors,Real-time systems,Probes,Monitoring,Estimation,Biological system modeling,Machine learning
AI 理解论文
溯源树
样例
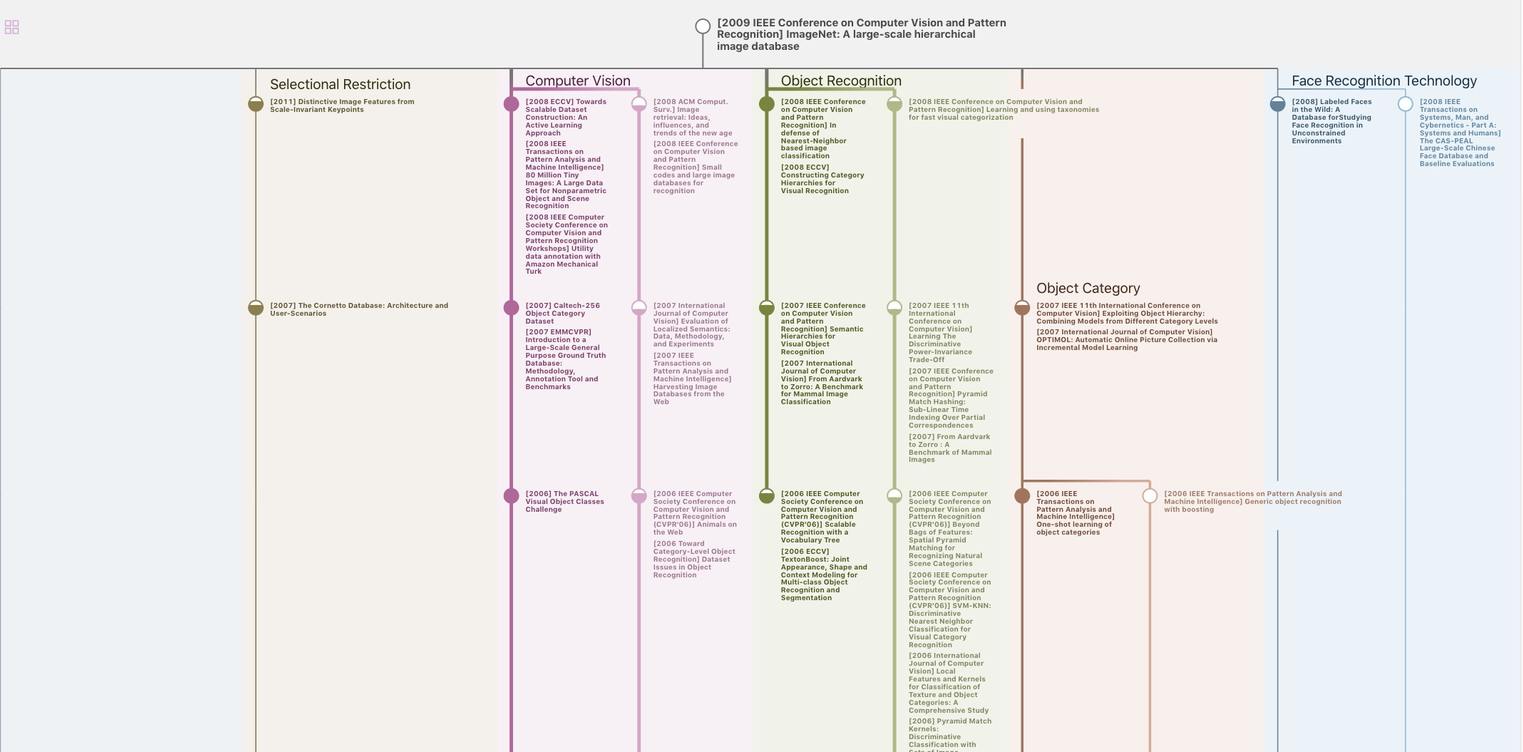
生成溯源树,研究论文发展脉络
Chat Paper
正在生成论文摘要