Multimapper: Data Density Sensitive Topological Visualization
2018 18TH IEEE INTERNATIONAL CONFERENCE ON DATA MINING WORKSHOPS (ICDMW)(2018)
摘要
Mapper is an algorithm that summarizes the topological information contained in a dataset and provides an insightful visualization. It takes as input a point cloud which is possibly high-dimensional, a filter function on it and an open cover on the range of the function. It returns the nerve simplicial complex of the pullback of the cover. Mapper can be considered a discrete approximation of the topological construct called Reeb space, as analysed in the 1-dimensional case by [Carri et al.,]. Despite its success in obtaining insights in various fields such as in [Kamruzzaman et al., 2016], Mapper is an ad hoc technique requiring lots of parameter tuning. There is also no measure to quantify goodness of the resulting visualization, which often deviates from the Reeb space in practice. In this paper, we introduce a new cover selection scheme for data that reduces the obscuration of topological information at both the computation and visualisation steps. To achieve this, we replace global scale selection of cover with a scale selection scheme sensitive to local density of data points. We also propose a method to detect some deviations in Mapper from Reeb space via computation of persistence features on the Mapper graph.
更多查看译文
关键词
Data Visualization, Topology, Mapper
AI 理解论文
溯源树
样例
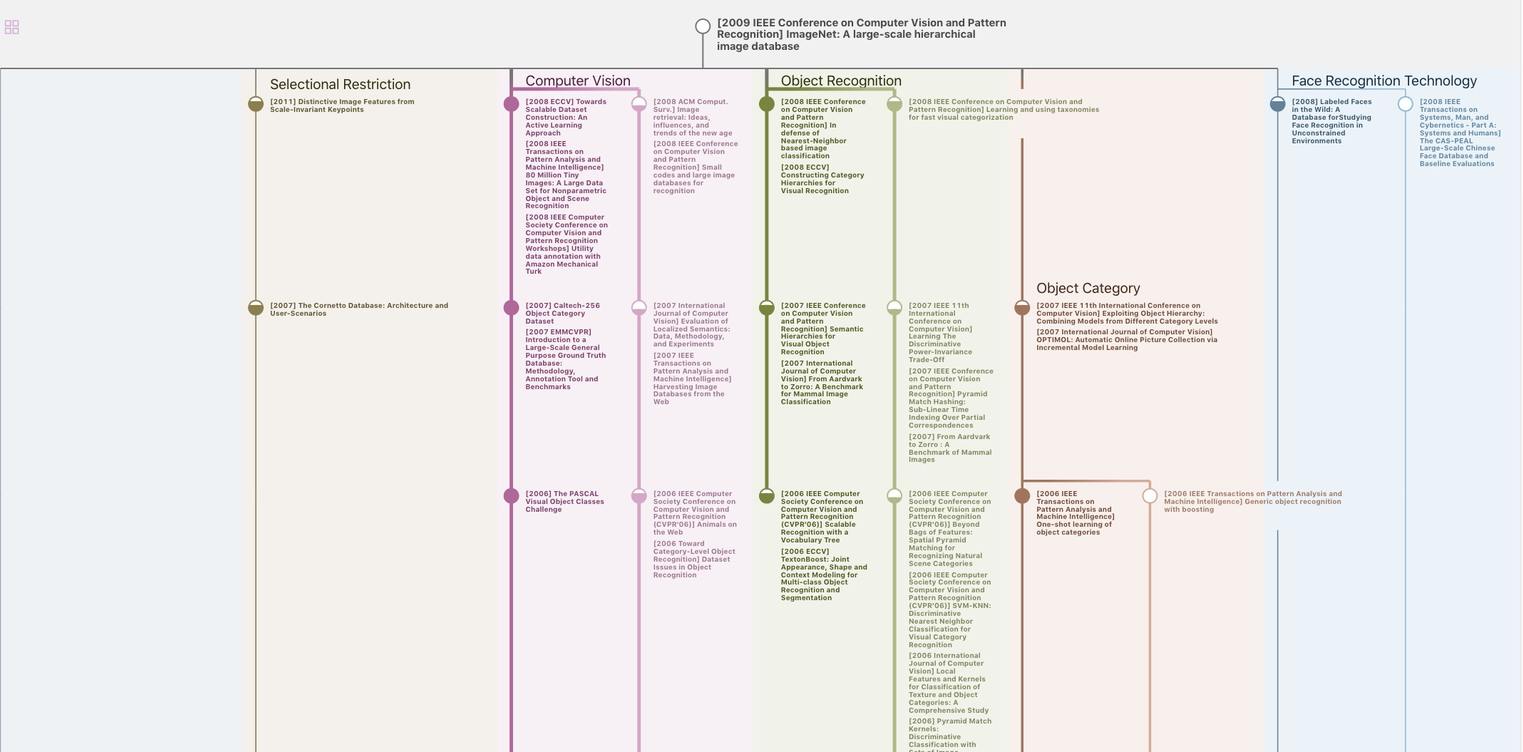
生成溯源树,研究论文发展脉络
Chat Paper
正在生成论文摘要