Elastic Nonnegative Matrix Factorization
2018 18TH IEEE INTERNATIONAL CONFERENCE ON DATA MINING WORKSHOPS (ICDMW)(2019)
摘要
Nonnegative Matrix Factorization factors a large matrix into smaller nonnegative components. Non-negative models are often more amenable to interpretation vis-a-vis standard principal component analysis where negative entries may not correspond to any physical process. Non-negative matrix factorization has been used widely in a variety of data mining settings, in a multitude of disciplines, including the famous Netflix challenge. Finding optimum solution to these problems are intractable. Iterative updates are widely used to find a local optima in most algorithms, but many of these updates are unstable and lack strong convergence guarantees, including several well known ones. We provide a technique that guarantees convergence even under distributed updates (with mild restrictions). Moreover there has been extensive research in many variants of nonnegative matrix factorization in the presence of noise. None of these robust algorithms have guaranteed convergence to even a local optima. We provide an algorithm that adheres to the general analysis technique and addresses noise and contamination. The algorithm is implemented in an elastic distributed system and provides a better quality of factorization than many alternative approaches on a variety of datasets
更多查看译文
关键词
nonnegative matrix factorization, algorithms, robust algorithms
AI 理解论文
溯源树
样例
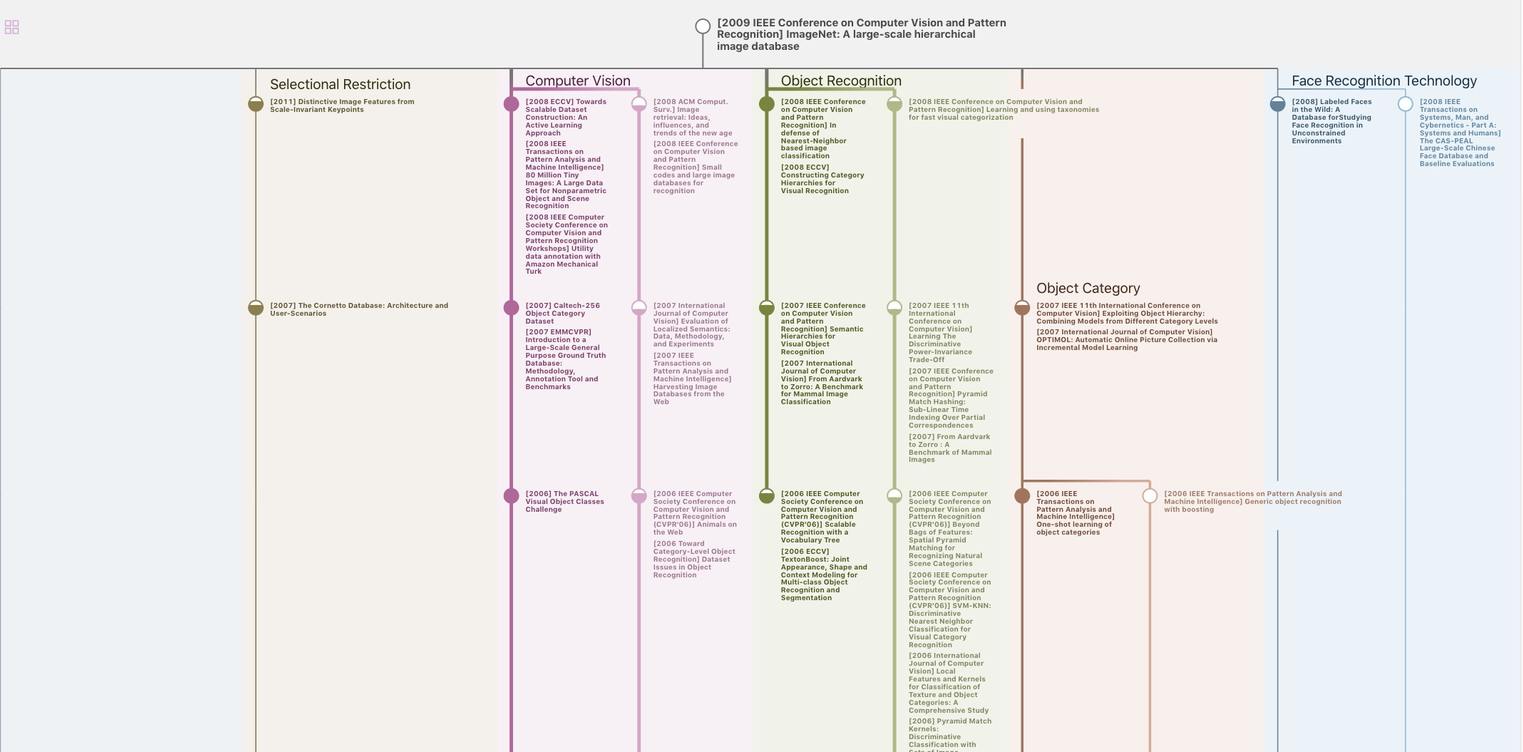
生成溯源树,研究论文发展脉络
Chat Paper
正在生成论文摘要