Angiography-Based Machine Learning For Predicting Fractional Flow Reserve In Intermediate Coronary Artery Lesions
JOURNAL OF THE AMERICAN HEART ASSOCIATION(2019)
摘要
Background-An angiography-based supervised machine learning (ML) algorithm was developed to classify lesions as having fractional flow reserve <= 0.80 versus >0.80.Methods and Results-With a 4:1 ratio, 1501 patients with 1501 intermediate lesions were randomized into training versus test sets. Between the ostium and 10mmdistal to the target lesion, a series of angiographic lumen diameter measurements along the centerline was plotted. The 24 computed angiographic features based on the diameter plot and 4 clinical features (age, sex, body surface area, and involve segment) were used for ML by XGBoost. The model was independently trained and tested by 2000 bootstrap iterations. External validation with 79 patients was conducted. Including all 28 features, the ML model with 5-fold cross-validation in the 1204 training samples predicted fractional flow reserve <= 0.80 with overall diagnostic accuracy of 78 +/- 4% (averaged area under the curve: 0.84 +/- 0.03). The 12 high-ranking features selected by scatter search were involved segment; body surface area; distal lumen diameter; minimal lumen diameter; length of a lumen diameter <2.0 mm, <1.5 mm, and <1.25 mm; mean lumen diameter within the worst segment; sex; diameter stenosis; distal 5-mm reference lumen diameter; and length of diameter stenosis >70%. Using those 12 features, theML predicted fractional flow reserve <= 0.80 in the test set with sensitivity of 84%, specificity of 80%, and overall accuracy of 82% (area under the curve: 0.87). The averaged diagnostic accuracy in bootstrap replicates was 81 +/- 1% (averaged area under the curve: 0.87 +/- 0.01). External validation showed accuracy of 85% (area under the curve: 0.87).Conclusions-Angiography-based ML showed good diagnostic performance in identifying ischemia-producing lesions and reduced the need for pressure wires.
更多查看译文
关键词
artificial intelligence,coronary angiography,fractional flow reserve,machine learning
AI 理解论文
溯源树
样例
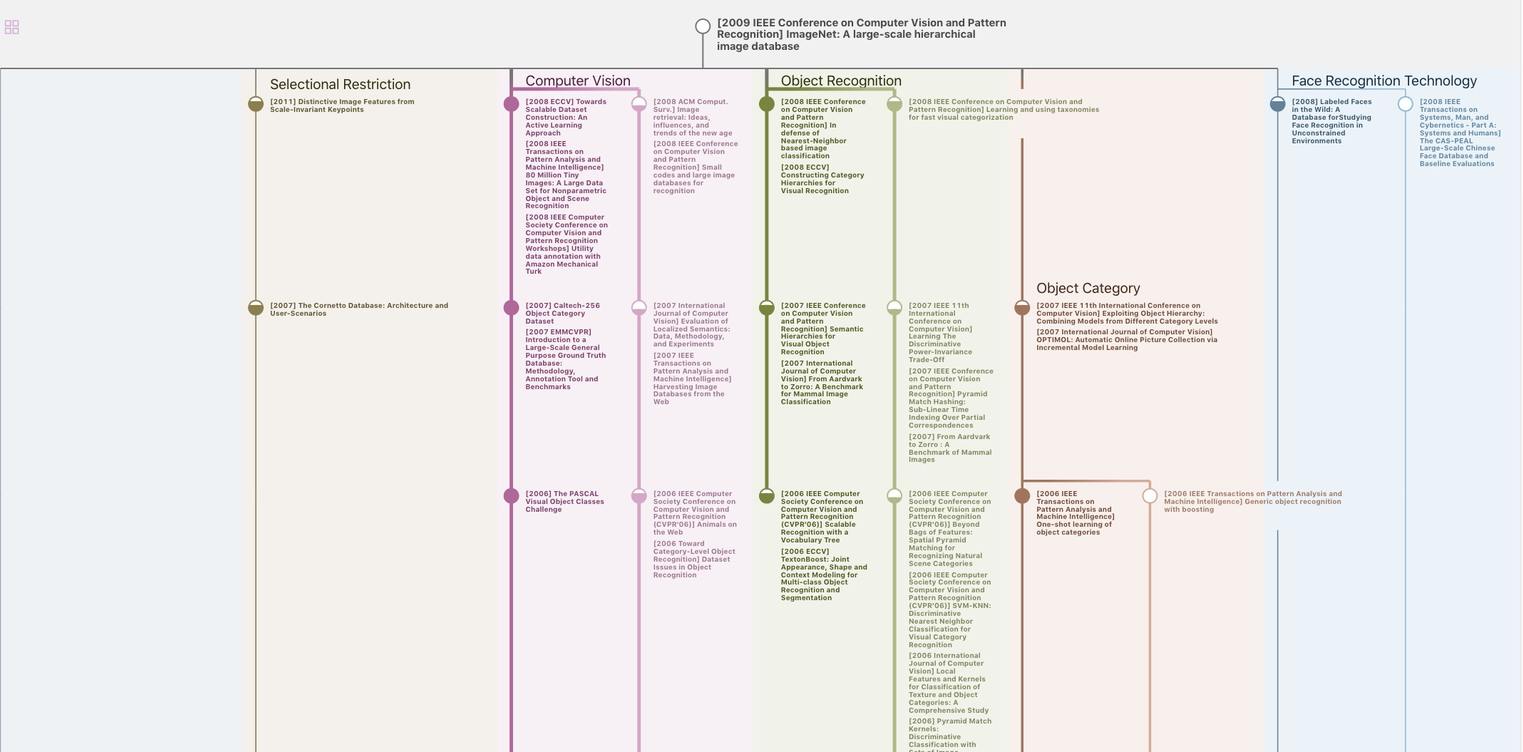
生成溯源树,研究论文发展脉络
Chat Paper
正在生成论文摘要