WatchNet: Efficient and Depth-based Network for People Detection in Video Surveillance Systems
2018 15th IEEE International Conference on Advanced Video and Signal Based Surveillance (AVSS)(2018)
摘要
We propose a deep-learning approach for people detection on depth imagery. The approach is designed to be deployed as an autonomous appliance for identifying people attacks and intrusion in video surveillance scenarios. To this end, we propose a fully-convolutional and sequential network, named WatchNet, that localizes people in depth images by predicting human body landmarks such as head and shoulders. We use a large synthetic dataset to train the network with abundant data and generate automatic annotations. Adaptation to real data is performed via fine tuning with real depth images.The proposed method is validated in a novel and challenging database with about 29k top view images collected from several sequences including different people assaults. A comparative evaluation is given between our approach and other standard methods, showing remarkable detection results and efficiency. The network runs in 10 and 28 FPS using CPU and GPU, respectively.
更多查看译文
关键词
Feature extraction,Cameras,Video surveillance,Head,Training,Databases,Buildings
AI 理解论文
溯源树
样例
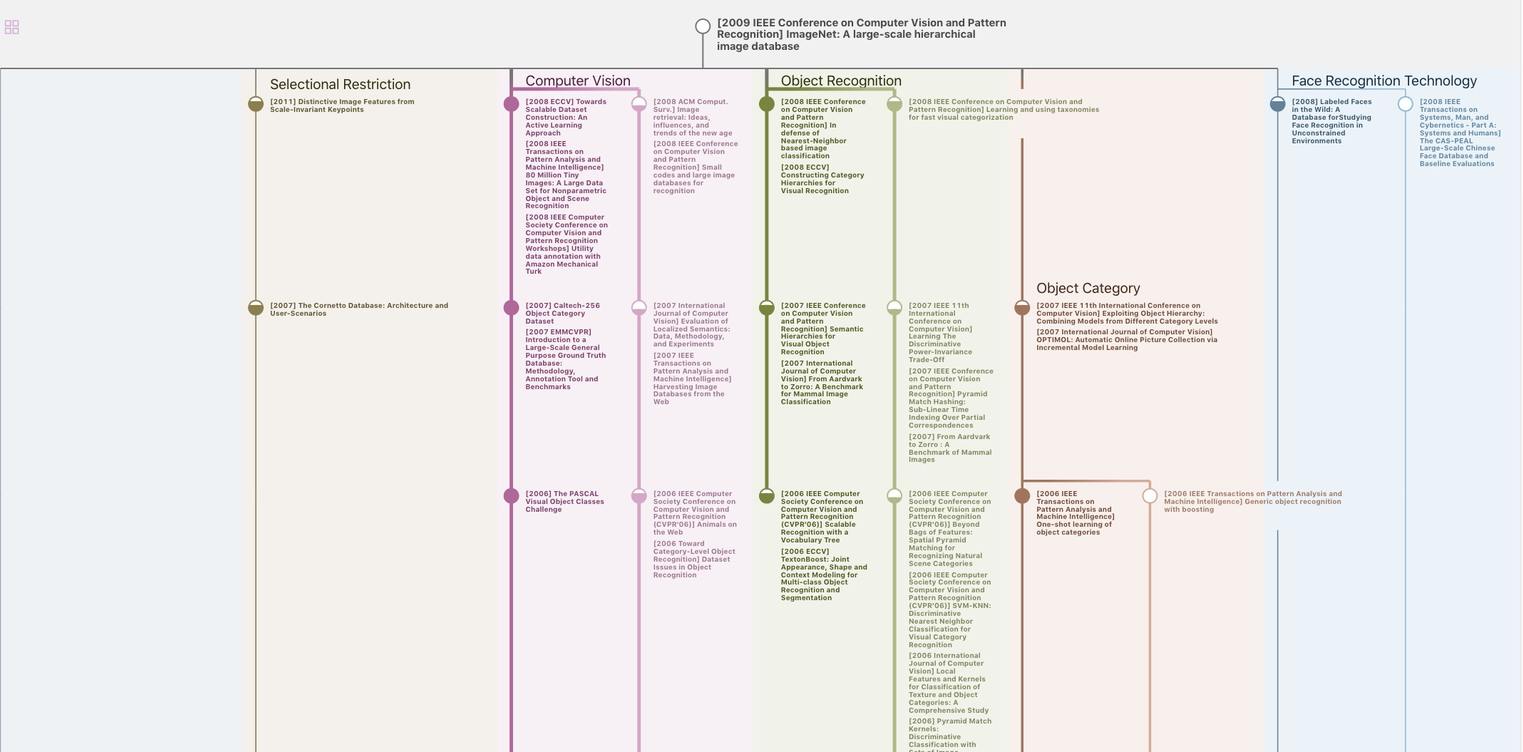
生成溯源树,研究论文发展脉络
Chat Paper
正在生成论文摘要