Automatic Detection Of Respiratory Effort Related Arousals From Polysomnographic Recordings
2018 COMPUTING IN CARDIOLOGY CONFERENCE (CINC)(2018)
摘要
A reliable automatic categorization of respiratory effort is paramount for sleep-disordered breathing characterization from polysomnography. A respiratory effort related arousal (RERA) is a subtle breathing obstruction associated with an arousal. For identification of RERAs we focused on: chest and abdomen EMGs, airflow, and EEG; monitoring changes in ECG and SaO(2). The quality of signals was assessed to overcome sensor associated problems and sporadic individual signal losses. We evaluated an ensemble learning and a deep learning approach using the engineered feature set trained on the 994 available records. The initial ensemble model was officially scored achieving a 0.081 area under the precision-recall curve (AUPRC) on a test set, whereas for the recurrent neural network model the average AUPRC was 0.295, obtained using 10-fold cross-validation.
更多查看译文
关键词
automatic detection,respiratory effort,polysomnographic recordings,reliable automatic categorization,sleep-disordered breathing characterization,breathing obstruction
AI 理解论文
溯源树
样例
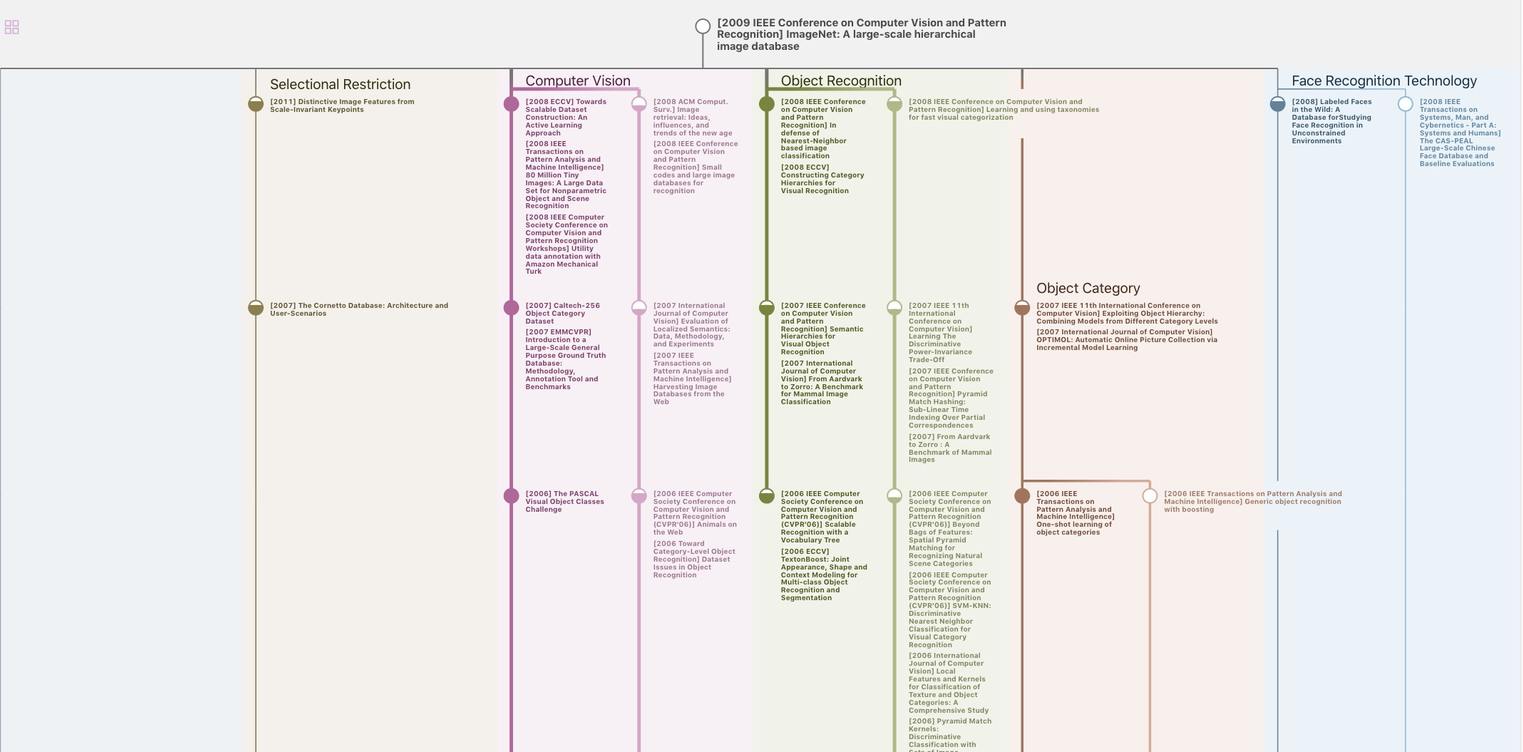
生成溯源树,研究论文发展脉络
Chat Paper
正在生成论文摘要