Joint Sparsity Pattern Recovery With 1-b Compressive Sensing in Distributed Sensor Networks
IEEE Transactions on Signal and Information Processing over Networks(2019)
摘要
In this paper, we study the problem of joint sparse support recovery with 1-b quantized compressive measurements in a distributed sensor network. Multiple nodes in the network are assumed to observe sparse signals having the same but unknown sparse support. Each node quantizes its measurement vector element-wise to 1 b. First, we consider that all the quantized measurements are available at a central fusion center. We derive performance bounds for sparsity pattern recovery using 1-bit quantized measurements from multiple sensors when the maximum likelihood decoder is employed. We further develop two computationally tractable algorithms for joint sparse support recovery in the centralized setting. One algorithm minimizes a cost function defined as the sum of the likelihood function and the
$l_{1,\infty }$
quasi-norm, while the other algorithm extends the binary iterative hard thresholding algorithm to the multiple measurement vector case. Second, we consider a decentralized setting where each node transmits 1-b measurements to its one-hop neighbors. The basic idea behind the algorithms developed in the decentralized setting is to embed collaboration among nodes and fusion strategies. We show that even with noisy 1-b compressed measurements, joint support recovery can be carried out accurately in both centralized and decentralized settings. We further show that the performance of the proposed 1-bit compressive sensing-based algorithms is very close to that of their real-valued counterparts except when the signal-to-noise ratio is very small.
更多查看译文
关键词
Signal to noise ratio,Indexes,Measurement uncertainty,Information processing,Compressed sensing,Decoding,Iterative algorithms
AI 理解论文
溯源树
样例
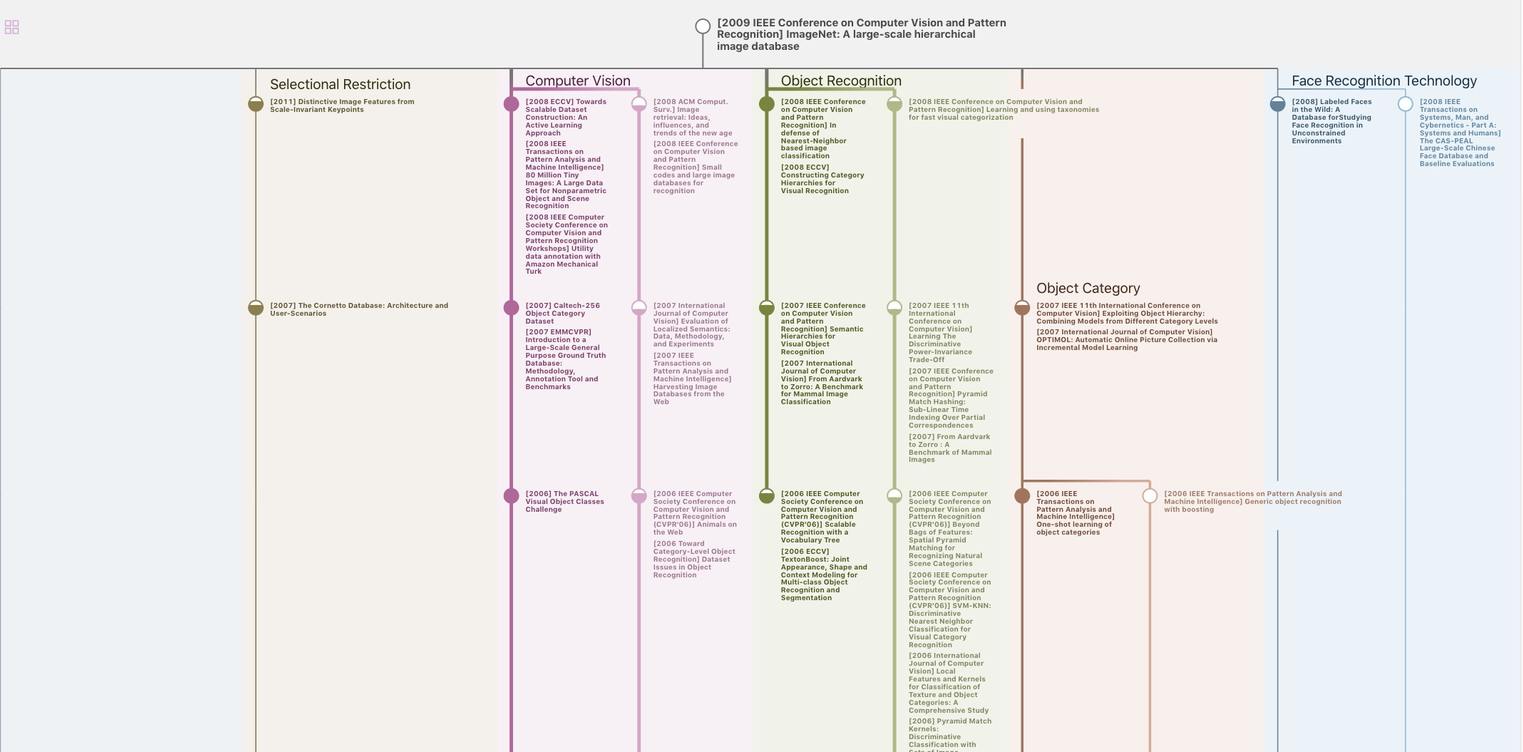
生成溯源树,研究论文发展脉络
Chat Paper
正在生成论文摘要