Exact Markov Chain-based Runtime Analysis of a Discrete Particle Swarm Optimization Algorithm on Sorting and OneMax
arxiv(2019)
摘要
We present a comprehensive analysis of a discrete particle swarm optimization (PSO) algorithm that can be adapted to work on a large class of discrete optimization problems. For this purpose, we model the algorithm's behavior by Markov chains with different choices of transition probabilities and prove, in most cases, matching lower and upper bounds on the expected return times. These new results on Markov chains enable us to derive lower and upper bounds on the expected number of function evaluations performed by the PSO algorithm with a single particle solving the sorting problem and OneMax. Additionally, we are able to prove for the first time upper bounds on the runtime of PSO with multiple particles. Our new techniques in the analysis of Markov chains include a useful indistinguishability property and a novel calculation of bounds by integration. Implicitly, we extend in this work the fitness level method to a non-elitist setting. Additionally, we present a guide that shows how our technical tools may be used in other settings.
更多查看译文
AI 理解论文
溯源树
样例
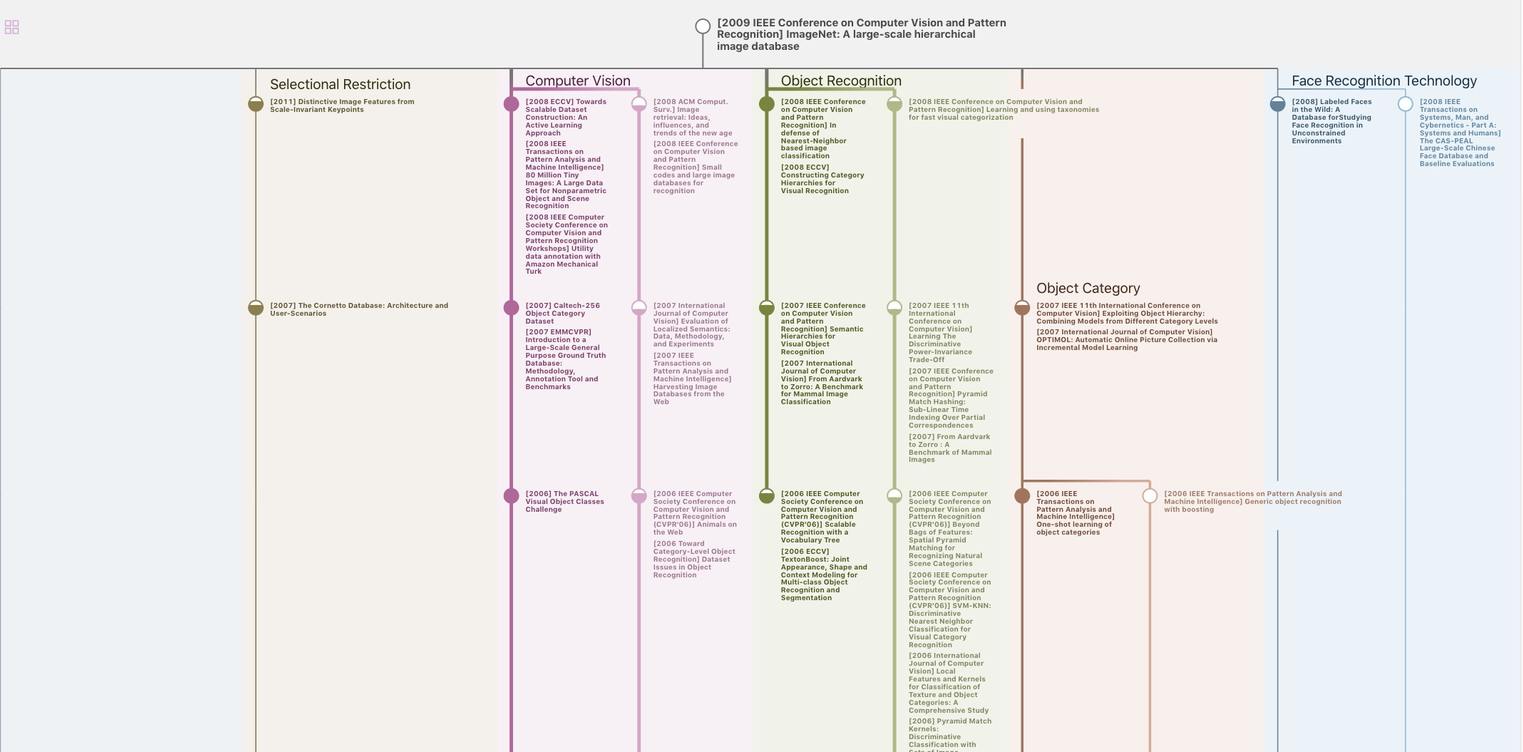
生成溯源树,研究论文发展脉络
Chat Paper
正在生成论文摘要