A Meta-Mdp Approach To Exploration For Lifelong Reinforcement Learning
AAMAS '19: PROCEEDINGS OF THE 18TH INTERNATIONAL CONFERENCE ON AUTONOMOUS AGENTS AND MULTIAGENT SYSTEMS(2019)
摘要
In this paper we consider the problem of how a reinforcement learning agent that is tasked with solving a sequence of reinforcement learning problems (Markov decision processes) can use knowledge acquired early in its lifetime to improve its ability to solve new problems. Specifically, we focus on the question of how the agent should explore when faced with a new environment. We show that the search for an optimal exploration strategy can be formulated as a reinforcement learning problem itself, albeit with a different timescale. We conclude with experiments that show the benefits of optimizing an exploration strategy using our proposed approach.
更多查看译文
关键词
Reinforcement Learning,Hierarchical RL,Exploration
AI 理解论文
溯源树
样例
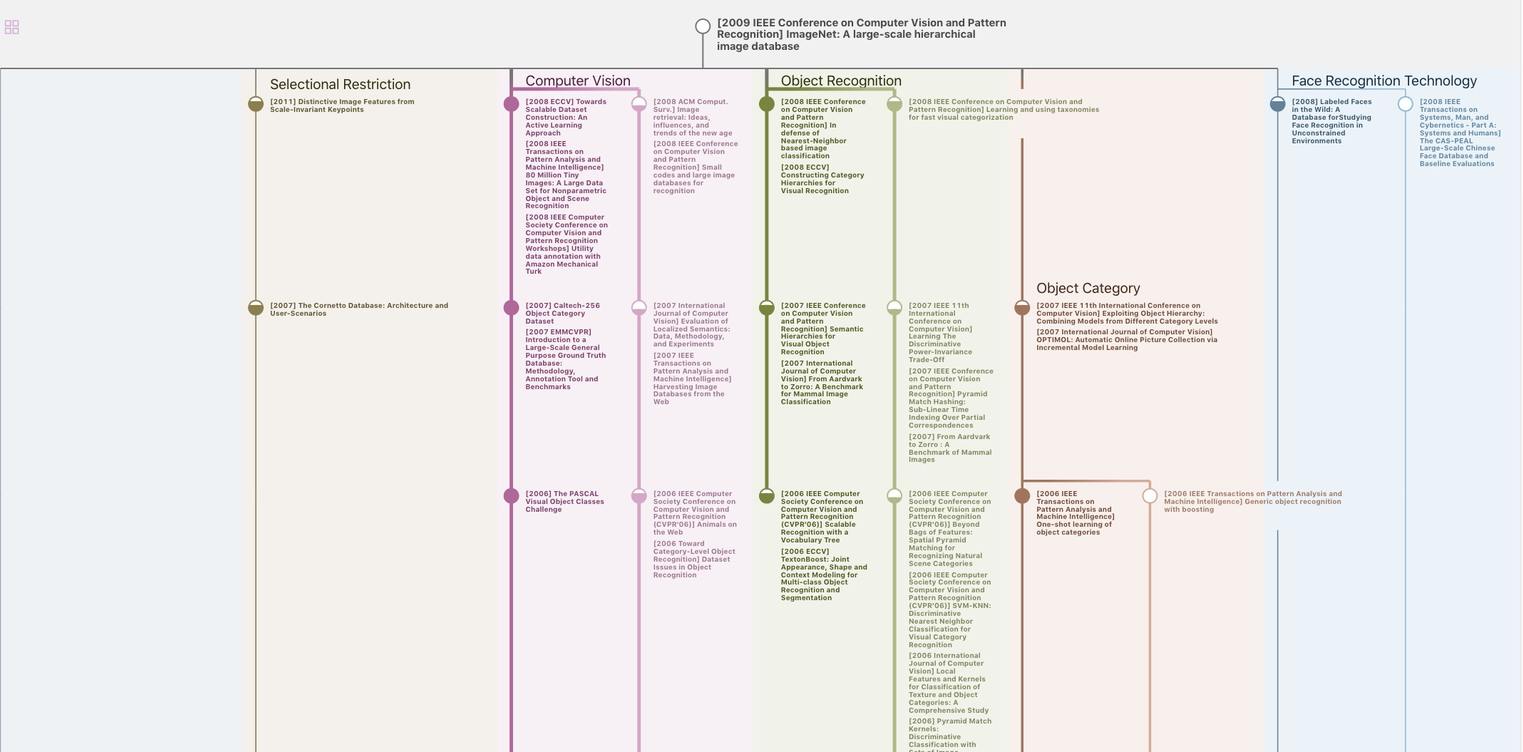
生成溯源树,研究论文发展脉络
Chat Paper
正在生成论文摘要