On the Convergence of (Stochastic) Gradient Descent with Extrapolation for Non-Convex Optimization
arxiv(2019)
摘要
Extrapolation is a well-known technique for solving convex optimization and variational inequalities and recently attracts some attention for non-convex optimization. Several recent works have empirically shown its success in some machine learning tasks. However, it has not been analyzed for non-convex minimization and there still remains a gap between the theory and the practice. In this paper, we analyze gradient descent and stochastic gradient descent with extrapolation for finding an approximate first-order stationary point in smooth non-convex optimization problems. Our convergence upper bounds show that the algorithms with extrapolation can be accelerated than without extrapolation.
更多查看译文
关键词
gradient descent,optimization,extrapolation,stochastic,convergence,non-convex
AI 理解论文
溯源树
样例
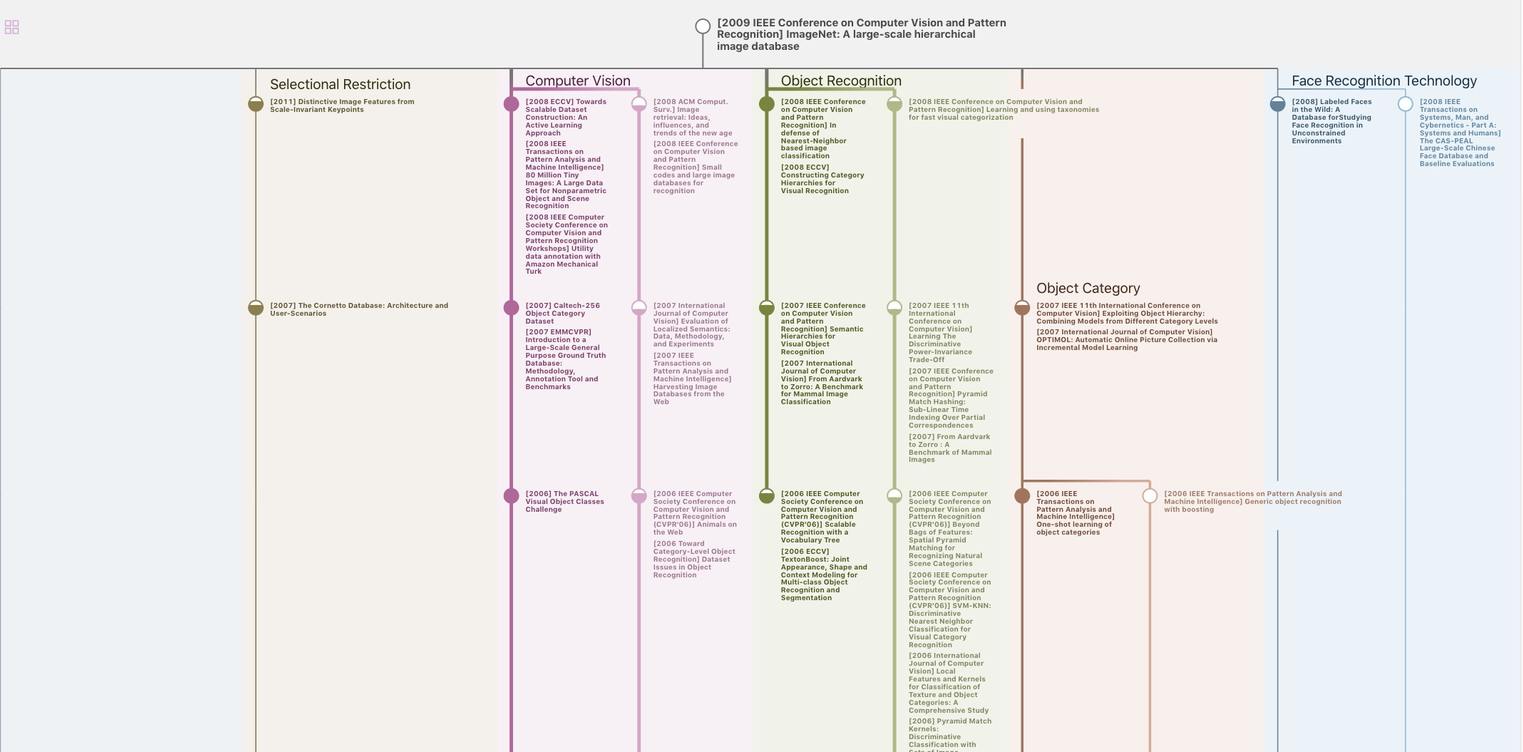
生成溯源树,研究论文发展脉络
Chat Paper
正在生成论文摘要