Diffusion Variational Autoencoders
PROCEEDINGS OF THE TWENTY-NINTH INTERNATIONAL JOINT CONFERENCE ON ARTIFICIAL INTELLIGENCE(2020)
摘要
A standard Variational Autoencoder, with a Euclidean latent space, is structurally incapable of capturing topological properties of certain datasets. To remove topological obstructions, we introduce Diffusion Variational Autoencoders (Delta VAE) with arbitrary (closed) manifolds as a latent space. A Diffusion Variational Autoencoder uses transition kernels of Brownian motion on the manifold. In particular, it uses properties of the Brownian motion to implement the reparametrization trick and fast approximations to the KL divergence. We show that the Delta VAE is indeed capable of capturing topological properties for datasets with a known underlying latent structure derived from generative processes such as rotations and translations.
更多查看译文
AI 理解论文
溯源树
样例
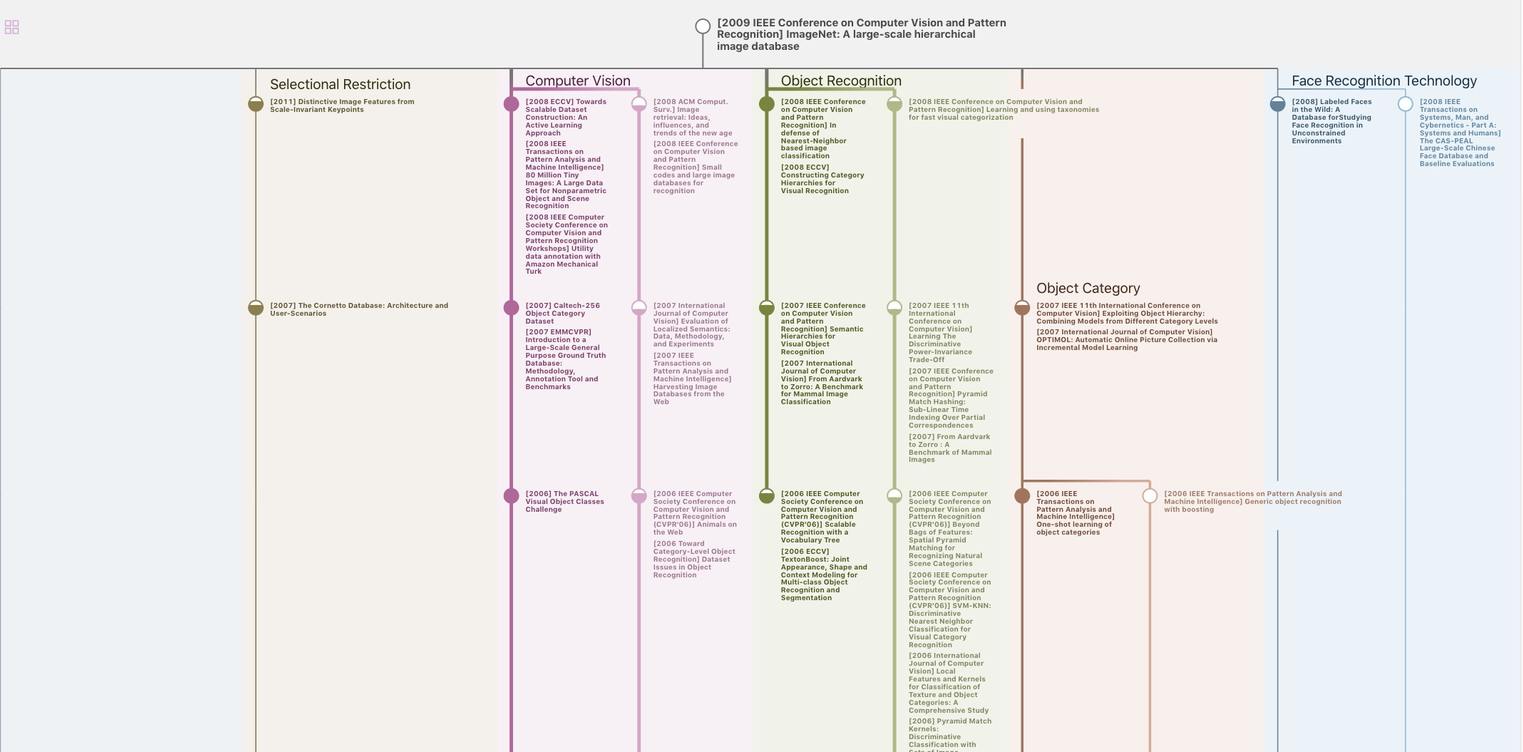
生成溯源树,研究论文发展脉络
Chat Paper
正在生成论文摘要