Multi-Product Dynamic Pricing in High-Dimensions with Heterogeneous Price Sensitivity
2020 IEEE INTERNATIONAL SYMPOSIUM ON INFORMATION THEORY (ISIT)(2020)
摘要
We consider the problem of multi-product dynamic pricing, in a contextual setting, for a seller of differentiated products. In this environment, the customers arrive over time and products are described by high-dimensional feature vectors. Each customer chooses a product according to the widely used Multinomial Logit (MNL) choice model and her utility depends on the product features as well as the prices offered. The seller a-priori does not know the parameters of the choice model but can learn them through interactions with customers. The seller's goal is to design a pricing policy that maximizes her cumulative revenue. This model is motivated by online marketplaces such as Airbnb platform and online advertising. We measure the performance of a pricing policy in terms of regret, which is the expected revenue loss with respect to a clairvoyant policy that knows the parameters of the choice model in advance and always sets the revenue-maximizing prices. We propose a pricing policy, named M3P, that achieves a T-period regret of O(log(Td)(root T+d log(T))) under heterogeneous price sensitivity for products with features of dimension d. We also use tools from information theory to prove that no policy can achieve worst-case T-regret better than Omega(root T). A full version of this paper is accessible at: https://arxiv.org/abs/1901.01030
更多查看译文
关键词
high-dimensional feature vectors,multinomial logit choice model,product features,pricing policy,revenue-maximizing prices,heterogeneous price sensitivity,multiproduct dynamic pricing,high-dimensions,differentiated products,online marketplaces,Airbnb platform,online advertising
AI 理解论文
溯源树
样例
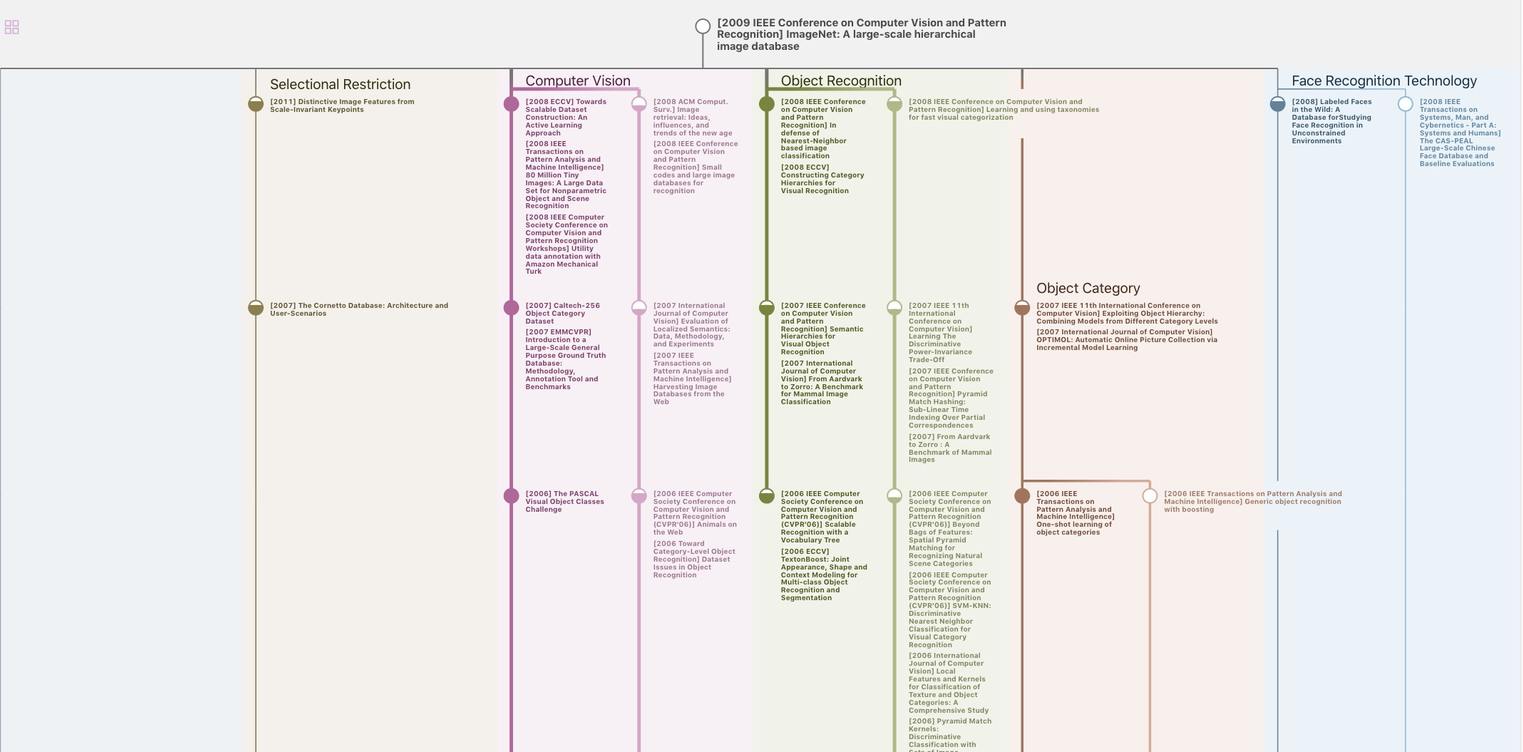
生成溯源树,研究论文发展脉络
Chat Paper
正在生成论文摘要