Scalable Gaussian Processes on Discrete Domains
CoRR(2019)
摘要
Kernel methods on discrete domains have shown great promise for many challenging data types, for instance, biological sequence data and molecular structure data. Scalable kernel methods like Support Vector Machines may offer good predictive performances but do not intrinsically provide uncertainty estimates. In contrast, probabilistic kernel methods like Gaussian Processes offer uncertainty estimates in addition to good predictive performance but fall short in terms of scalability. We present the first sparse Gaussian Process approximation framework on discrete input domains. Our framework achieves good predictive performance as well as uncertainty estimates using discrete optimization techniques. We present competitive results comparing our framework to baseline methods such as Support Vector Machines and full Gaussian Processes on synthetic data as well as on challenging real-world DNA sequence data.
更多查看译文
AI 理解论文
溯源树
样例
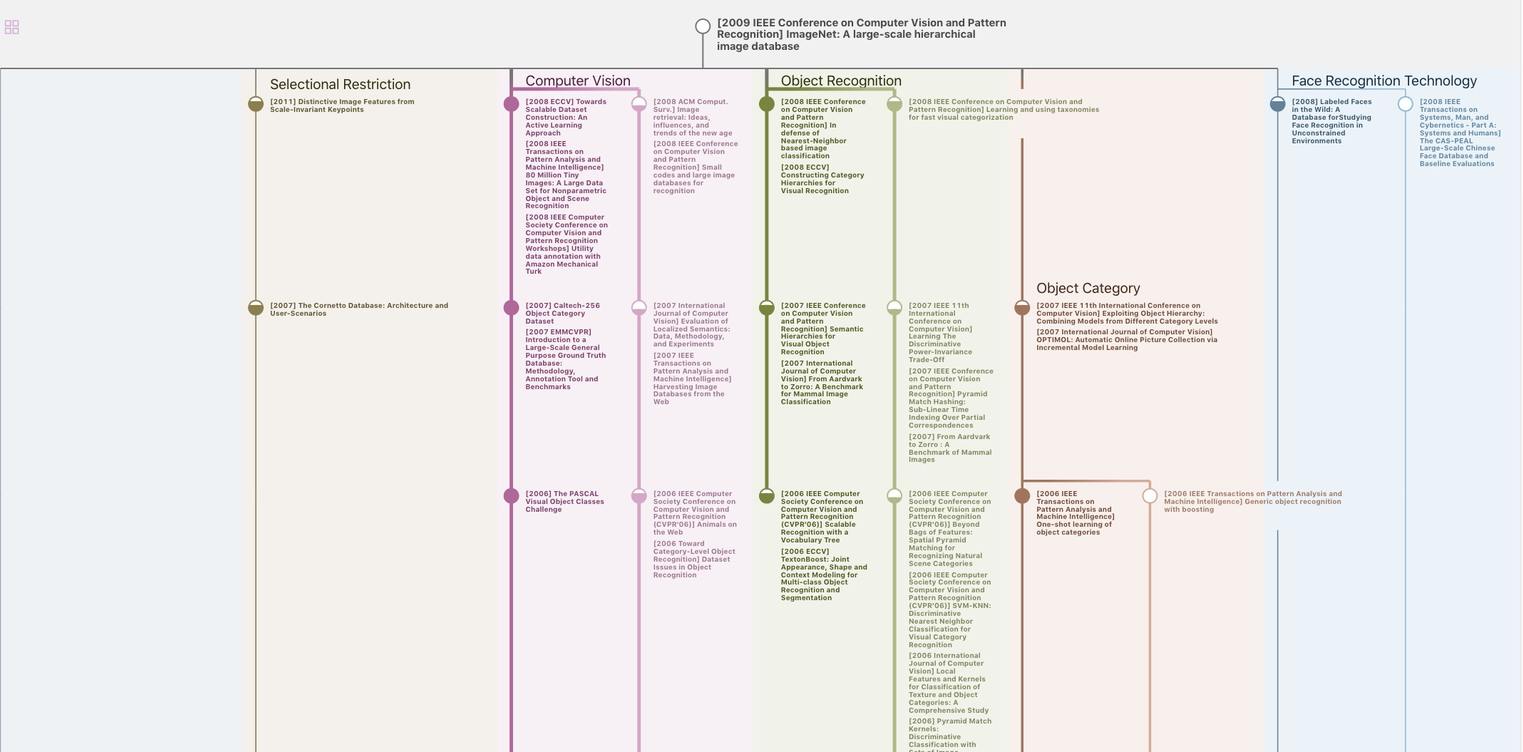
生成溯源树,研究论文发展脉络
Chat Paper
正在生成论文摘要