Sampling of probability measures in the convex order by Wasserstein projection
ANNALES DE L INSTITUT HENRI POINCARE-PROBABILITES ET STATISTIQUES(2020)
摘要
In this paper, for mu, and nu two probability measures on R-d with finite moments of order rho >= 1, we define the respective projections for the W-rho -Wasserstein distance of mu, and nu on the sets of probability measures dominated by nu and of probability measures larger than mu, in the convex order. The W-2-projection of mu, can be easily computed when mu, and nu have finite support by solving a quadratic optimization problem with linear constraints. In dimension d = 1, Gozlan et al. (Ann. Inst. Henri Poincare Probab. Stat. 54 (3) (2018) 1667-1693) have shown that the projection of mu does not depend on rho. We explicit their quantile functions in terms of those of mu and nu. The motivation is the design of sampling techniques preserving the convex order in order to approximate Martingale Optimal Transport problems by using linear programming solvers. We prove convergence of the Wasserstein projection based sampling methods as the sample sizes tend to infinity and illustrate them by numerical experiments.
更多查看译文
关键词
Convex order,Martingale optimal transport,Wasserstein distance,Sampling techniques,Linear programming
AI 理解论文
溯源树
样例
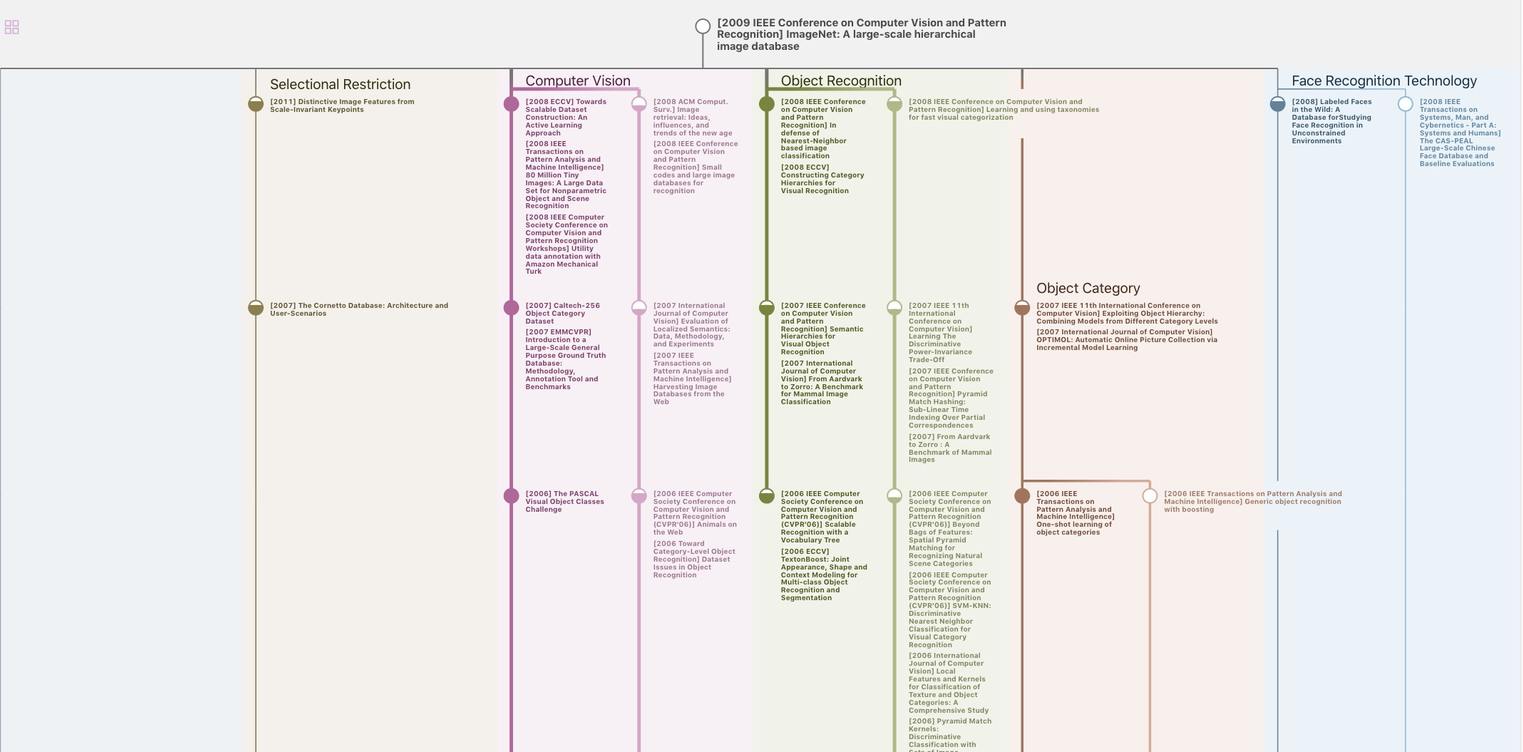
生成溯源树,研究论文发展脉络
Chat Paper
正在生成论文摘要