Fast cloud image segmentation with superpixel analysis based convolutional networks
2017 International Conference on Systems, Signals and Image Processing (IWSSIP)(2017)
摘要
Due to the various noises, the cloud image segmentation becomes a big challenge for atmosphere prediction. CNN is capable of learning discriminative features from complex data, but this may be quite time-consuming in pixel-level segmentation problems. In this paper we propose superpixel analysis based CNN (SP-CNN) for high efficient cloud image segmentation. SP-CNN employs image over-segmentation of superpixels as basic entities to preserve local consistency. SP-CNN takes the image patches centered at representative pixels in every superpixel as input, and all superpixels are classified as cloud or non-cloud part by voting of the representative pixels. It greatly reduces the computational burden on CNN learning. In order to avoid the ambiguity from superpixel boundaries, SP-CNN selects the representative pixels uniformly from the eroded superpixels. Experimental analysis demonstrates that SP-CNN guarantees both the effectiveness and efficiency in cloud segmentation.
更多查看译文
关键词
Cloud image,Superpixel analysis,Convolutional neural networks,Cloud segmentation
AI 理解论文
溯源树
样例
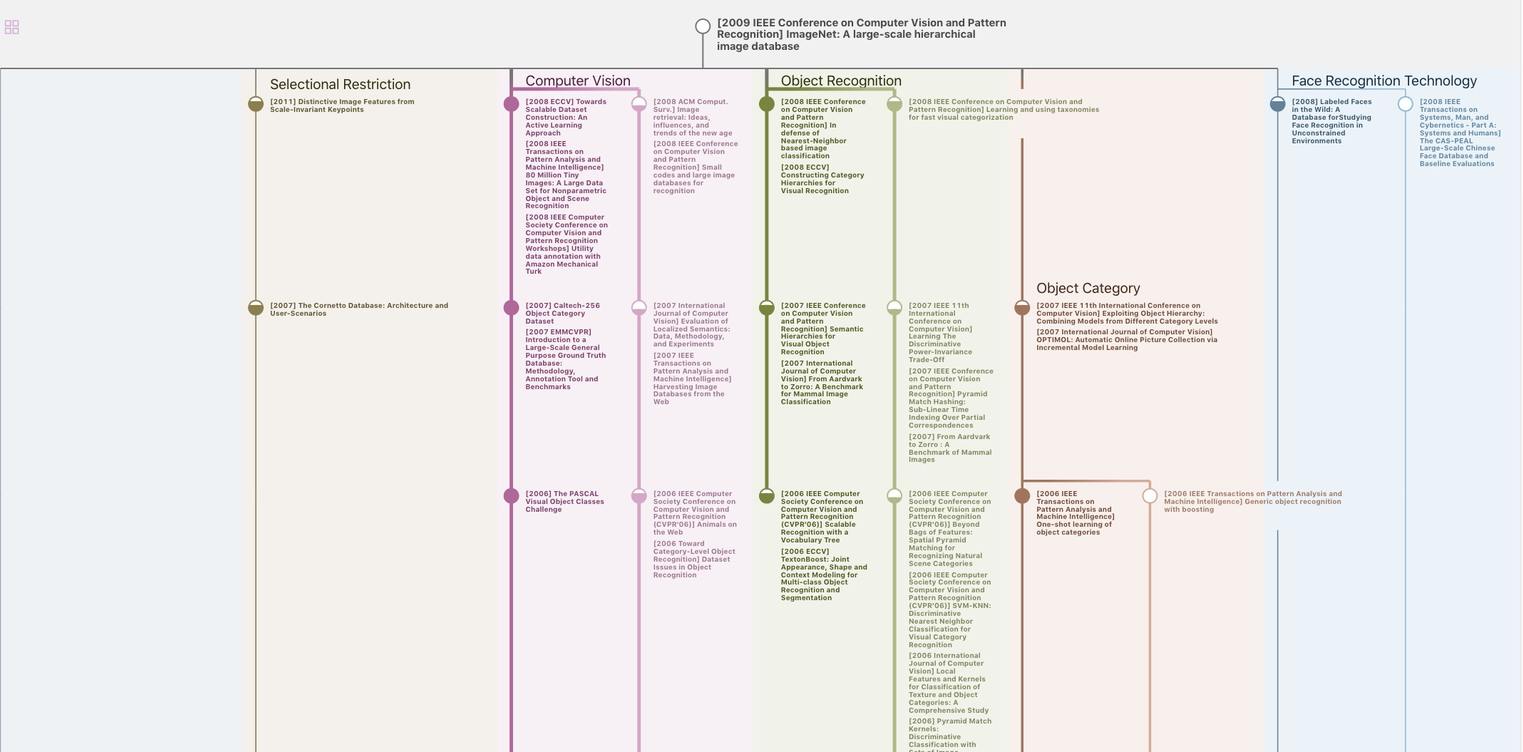
生成溯源树,研究论文发展脉络
Chat Paper
正在生成论文摘要