On Convergence of Heuristics Based on Douglas-Rachford Splitting and ADMM to Minimize Convex Functions over Nonconvex Sets†
2018 56th Annual Allerton Conference on Communication, Control, and Computing (Allerton)(2018)
摘要
Recently, heuristics based on the Douglas-Rachford splitting algorithm and the alternating direction method of multipliers (ADMM) have found empirical success in minimizing convex functions over nonconvex sets, but not much has been done to improve the theoretical understanding of them. In this paper, we investigate convergence of these heuristics. First, we characterize optimal solutions of minimization problems involving convex cost functions over nonconvex constraint sets. We show that these optimal solutions are related to the fixed point set of the underlying nonconvex Douglas-Rachford operator. Next, we establish sufficient conditions under which the Douglas-Rachford splitting heuristic either converges to a point or its cluster points form a nonempty compact connected set. In the case where the heuristic converges to a point, we establish sufficient conditions for that point to be an optimal solution. Then, we discuss how the ADMM heuristic can be constructed from the Douglas-Rachford splitting algorithm. We show that, unlike in the convex case, the algorithms in our nonconvex setup are not equivalent to each other and have a rather involved relationship between them. Finally, we comment on convergence of the ADMM heuristic and compare it with the Douglas-Rachford splitting heuristic.
更多查看译文
关键词
Convex functions,Convergence,Optimized production technology,Heuristic algorithms,Minimization,Cost function
AI 理解论文
溯源树
样例
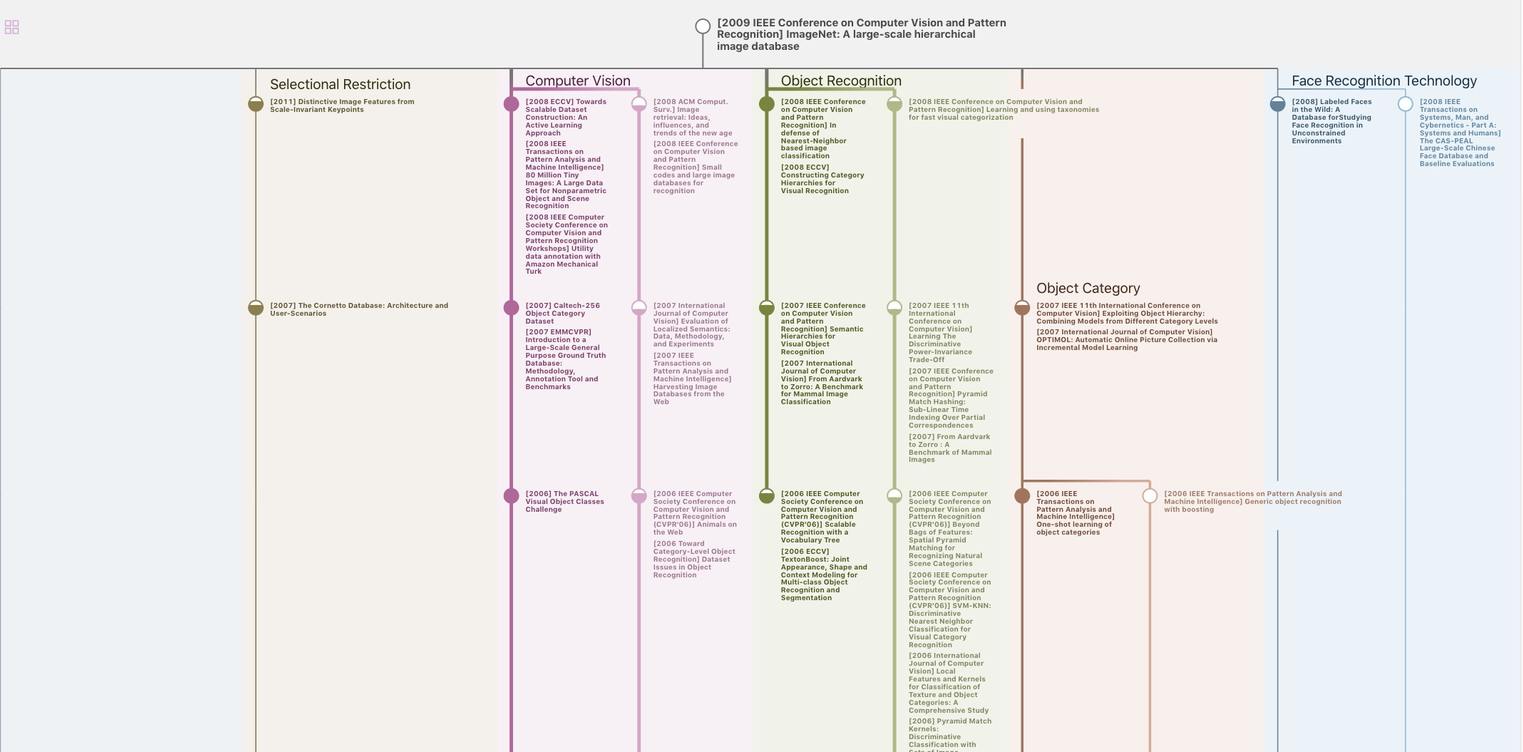
生成溯源树,研究论文发展脉络
Chat Paper
正在生成论文摘要