A Velocity Based HMM Framework for Indoor Localization.
APCC(2018)
摘要
Indoor fingerprint location is widely used in passenger flow analysis, location based service, and security monitoring. RSS is usually used as signal fingerprint. However, in these applications the acquisition process relies on the uplink data transmission of the target device. These signals are often non-normally distributed and fluctuate greatly when the target moves. In order to cope with the above difficulties, this paper proposes a hidden markov model (HMM) framework based on targets maximum speed. It combines the speed parameters with path constraints to improve the calculation of state transition matrix. The kernel density estimation method based on the Wiener process is also used to solve the confusion matrix of HMM, which improves the accuracy of estimating the RSS distribution of the uplink signal. The performance of the algorithm are compared with the traditional HMM and NB methods in an actual indoor scenario. The influence of the target moving speed and the length of the observation sequence on the positioning accuracy is also analyzed.
更多查看译文
关键词
Hidden Markov models,Kernel,Estimation,Training,Probability density function,Monitoring,Uplink
AI 理解论文
溯源树
样例
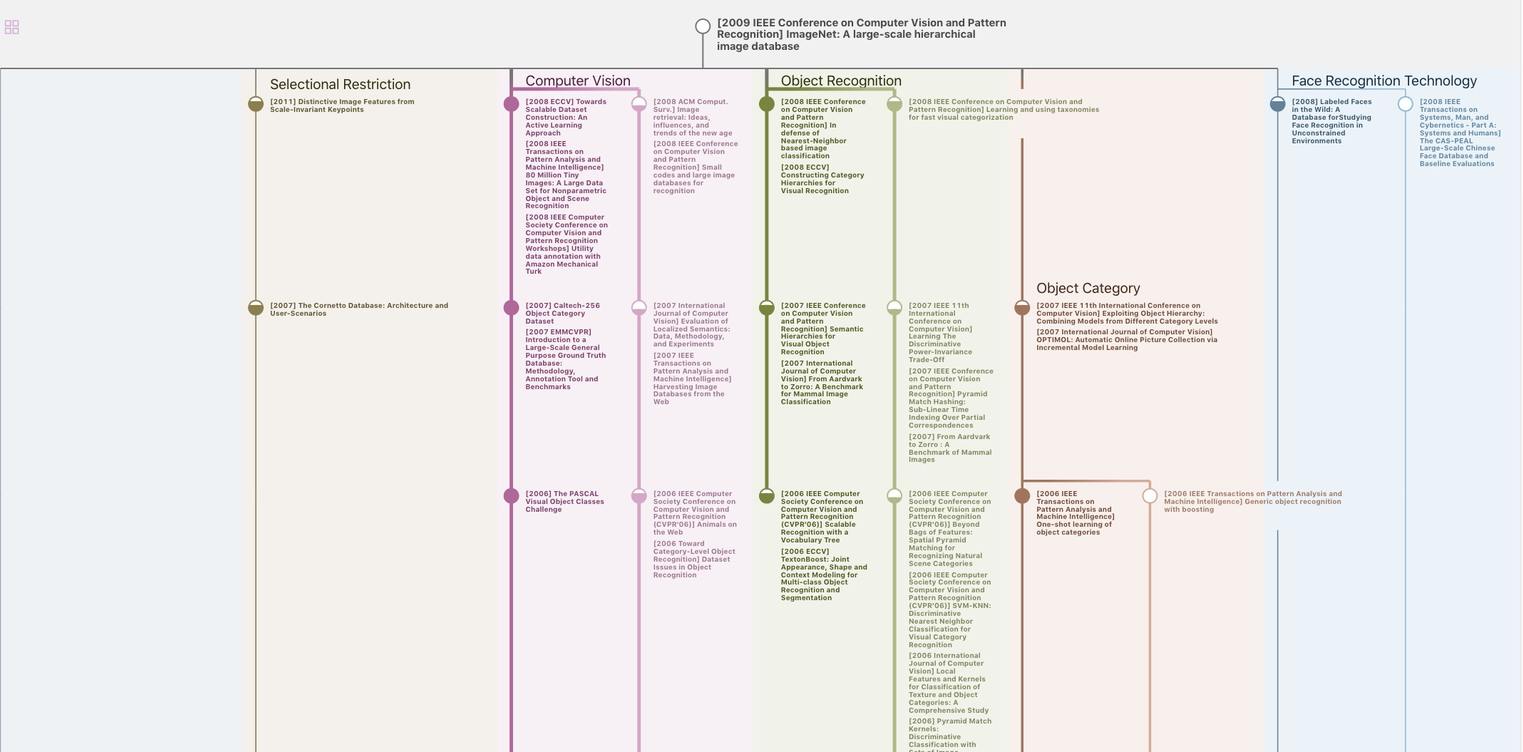
生成溯源树,研究论文发展脉络
Chat Paper
正在生成论文摘要