CAE-ADMM: Implicit Bitrate Optimization via ADMM-based Pruning in Compressive Autoencoders.
arXiv: Computer Vision and Pattern Recognition(2019)
摘要
We introduce CAE-ADMM (ADMM-pruned compressive autoencoder), a lossy image compression model, inspired by researches in neural architecture search (NAS) and is capable of implicitly optimizing the bitrate without the use of an entropy estimator. Our experiments show that by introducing alternating direction method of multipliers (ADMM) to the model pipeline, the pruning paradigm yields more accurate results (SSIM/MS-SSIM-wise) when compared to entropy-based approaches and that of traditional codecs (JPEG, JPEG 2000, etc.) while maintaining acceptable inference speed. We further explore the effectiveness of the pruning method in CAE-ADMM by examining the generated latent codes.
更多查看译文
AI 理解论文
溯源树
样例
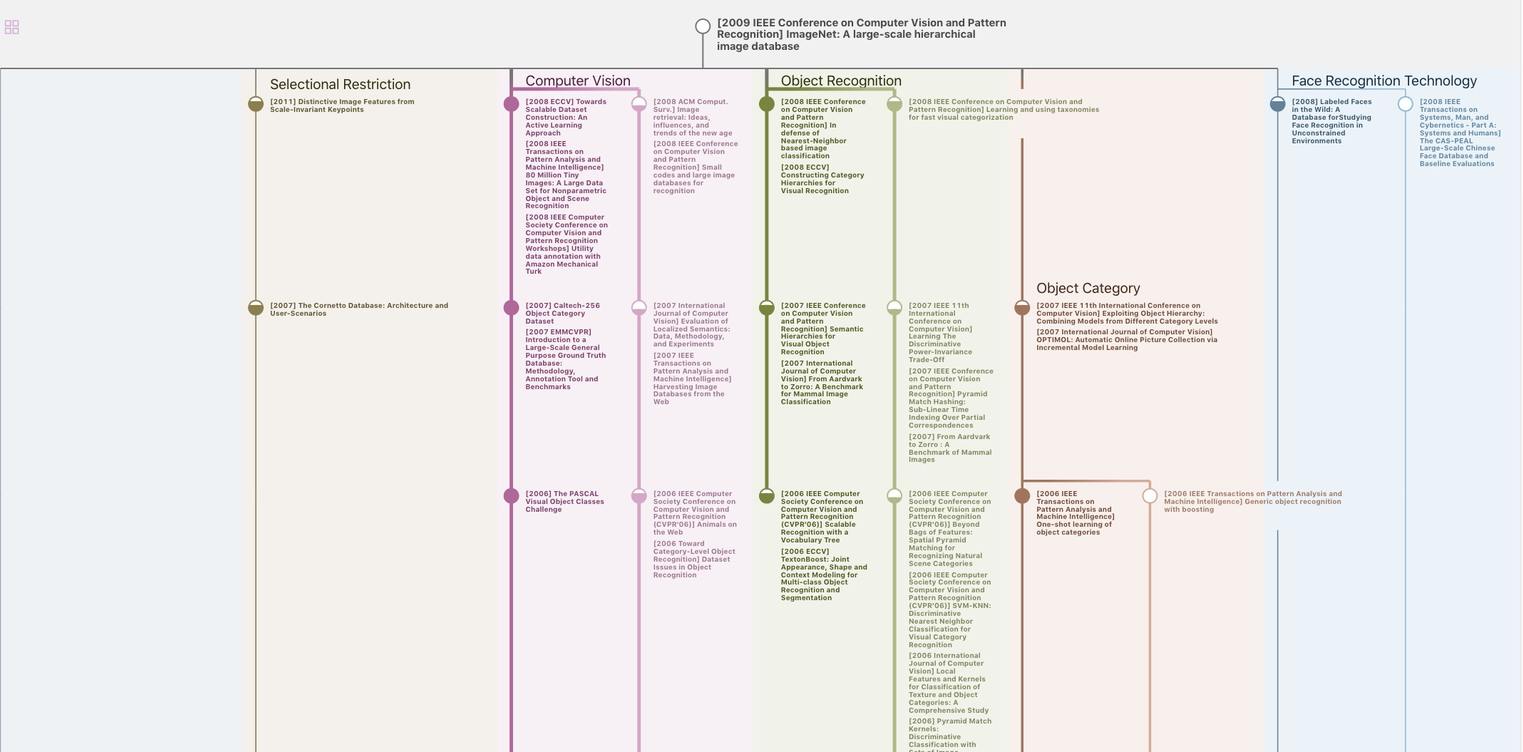
生成溯源树,研究论文发展脉络
Chat Paper
正在生成论文摘要