Derandomizing compressed sensing with combinatorial design
Frontiers in Applied Mathematics and Statistics(2018)
摘要
Compressed sensing is the art of reconstructing structured n-dimensional vectors from substantially fewer measurements than naively anticipated. A plethora of analytic reconstruction guarantees support this credo. The strongest among them are based on deep results from large-dimensional probability theory that require a considerable amount of randomness in the measurement design. Here, we demonstrate that derandomization techniques allow for considerably reducing the amount of randomness that is required for such proof strategies. More, precisely we establish uniform s-sparse reconstruction guarantees for C s log (n) measurements that are chosen independently from strength-four orthogonal arrays and maximal sets of mutually unbiased bases, respectively. These are highly structured families of C̃ n^2 vectors that imitate signed Bernoulli and standard Gaussian vectors in a (partially) derandomized fashion.
更多查看译文
关键词
compressed sensing,k-wise independence,orthogonal arrays,spherical design,derandomization
AI 理解论文
溯源树
样例
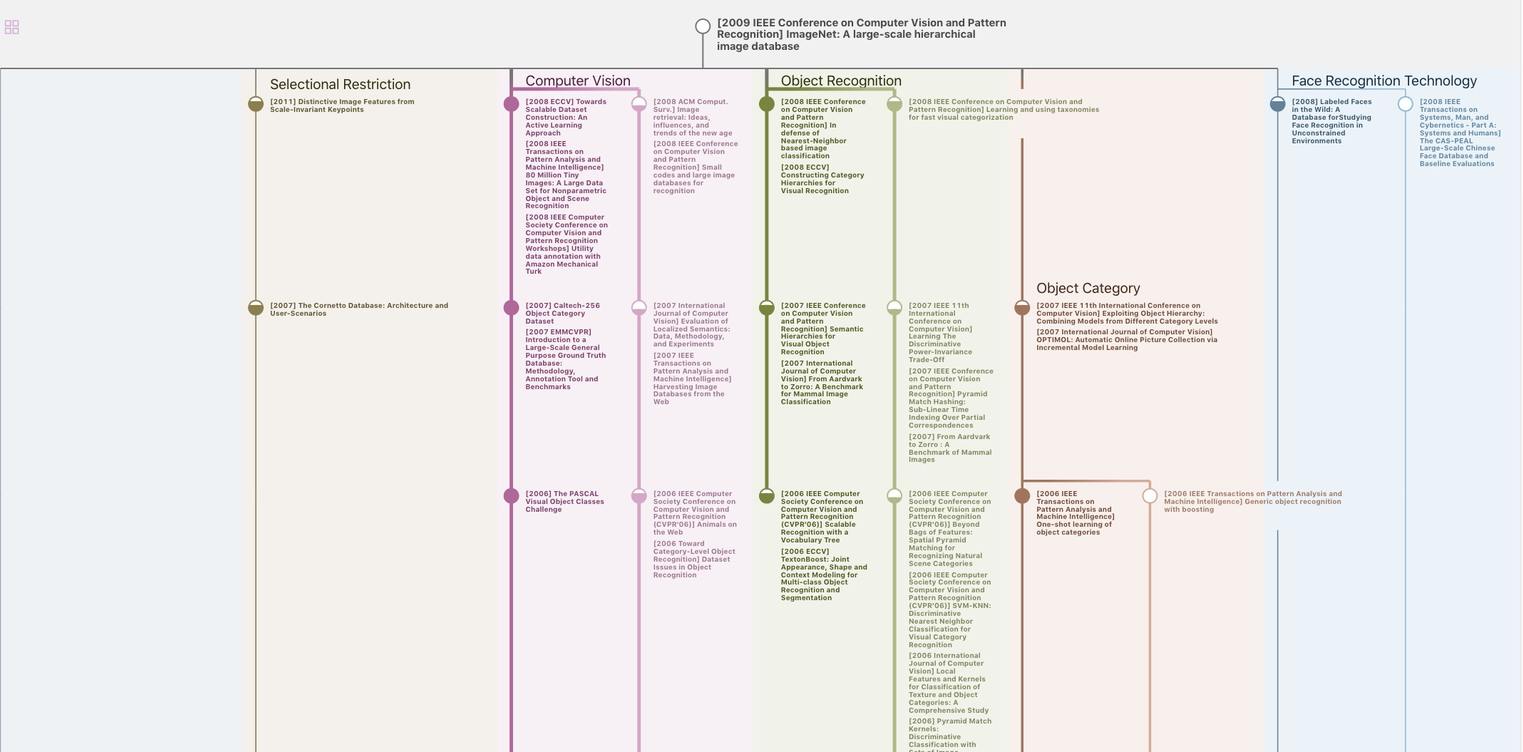
生成溯源树,研究论文发展脉络
Chat Paper
正在生成论文摘要