The information-theoretic value of unlabeled data in semi-supervised learning
INTERNATIONAL CONFERENCE ON MACHINE LEARNING, VOL 97(2019)
摘要
We quantify the separation between the numbers of labeled examples required to learn in two settings: Settings with and without the knowledge of the distribution of the unlabeled data. More specifically, we prove a separation by $\Theta(\log n)$ multiplicative factor for the class of projections over the Boolean hypercube of dimension $n$. We prove that there is no separation for the class of all functions on domain of any size. Learning with the knowledge of the distribution (a.k.a. fixed-distribution learning) can be viewed as an idealized scenario of semi-supervised learning where the number of unlabeled data points is so great that the unlabeled distribution is known exactly. For this reason, we call the separation the value of unlabeled data.
更多查看译文
关键词
unlabeled data,learning,information-theoretic,semi-supervised
AI 理解论文
溯源树
样例
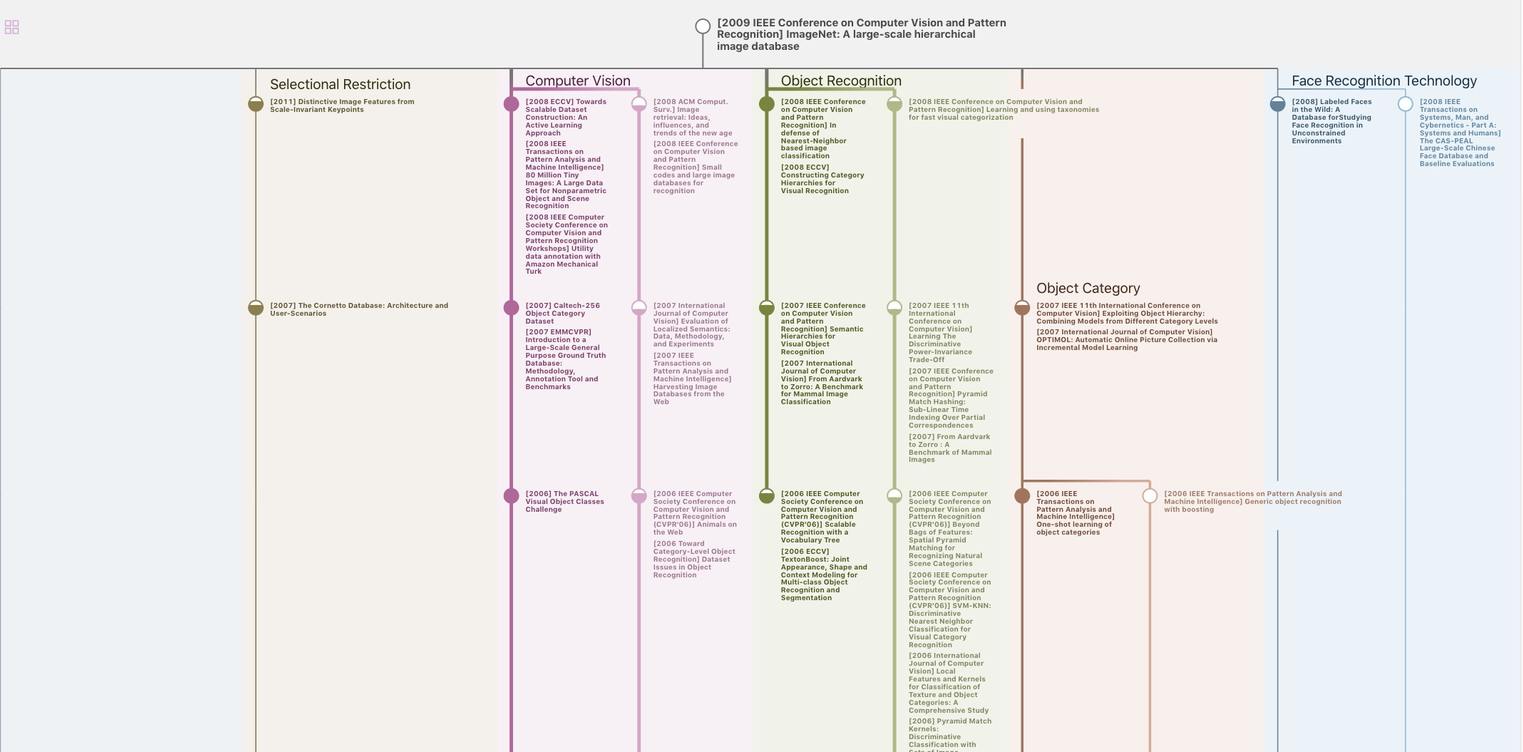
生成溯源树,研究论文发展脉络
Chat Paper
正在生成论文摘要