Adversarial Pseudo Healthy Synthesis Needs Pathology Factorization.
MIDL(2019)
摘要
Pseudo healthy synthesis, i.e. the creation of a subject-specific `healthyu0027 image from a pathological one, could be helpful in tasks such as anomaly detection, understanding changes induced by pathology and disease or even as data augmentation. We treat this task as a factor decomposition problem: we aim to separate what appears to be healthy and where disease is (as a map). The two factors are then recombined (by a network) to reconstruct the input disease image. We train our models in an adversarial way using either paired or unpaired settings, where we pair disease images and maps (as segmentation masks) when available. We quantitatively evaluate the quality of pseudo healthy images. We show in a series of experiments, performed in ISLES and BraTS datasets, that our method is better than conditional GAN and CycleGAN, highlighting challenges in using adversarial methods in the image translation task of pseudo healthy image generation.
更多查看译文
AI 理解论文
溯源树
样例
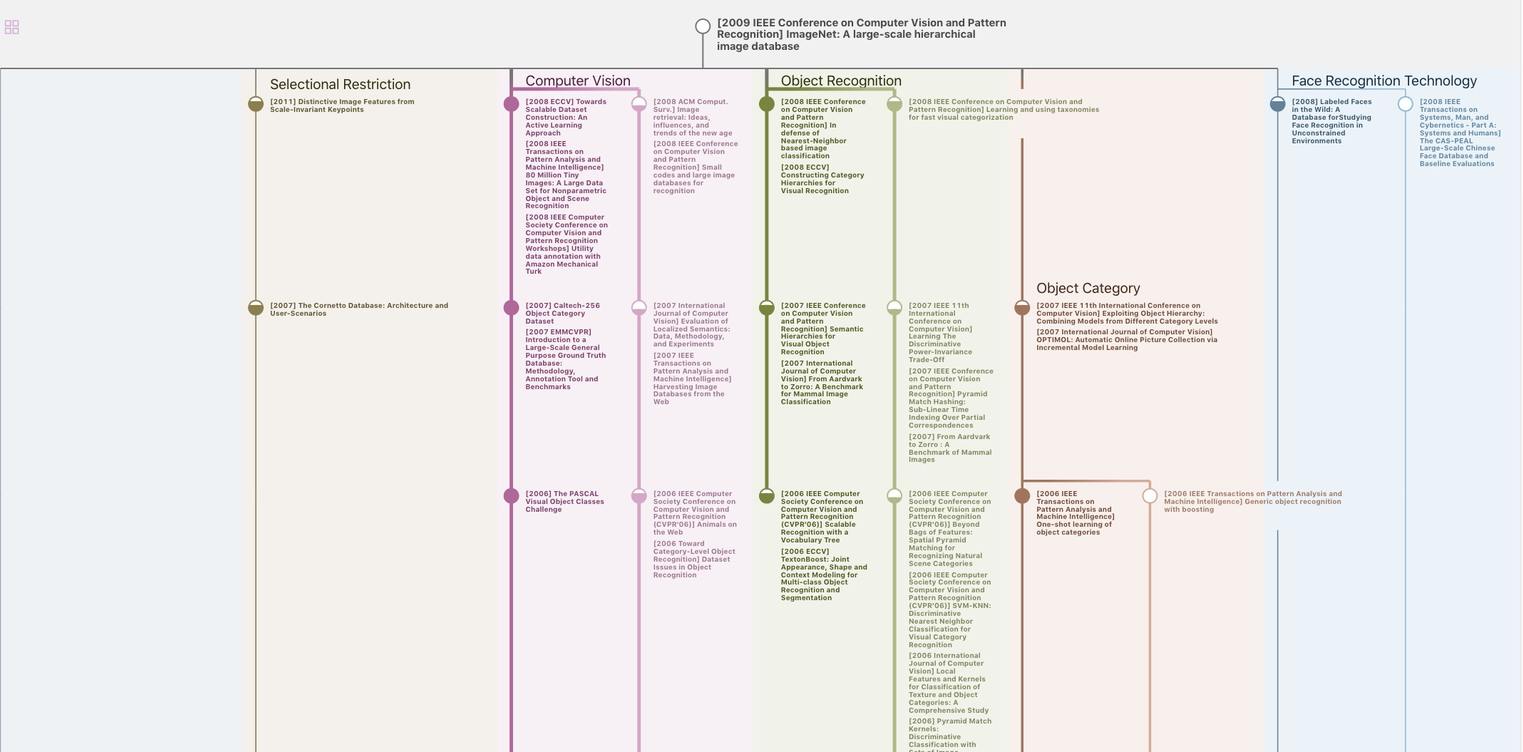
生成溯源树,研究论文发展脉络
Chat Paper
正在生成论文摘要