AuxNet: Auxiliary tasks enhanced Semantic Segmentation for Automated Driving
PROCEEDINGS OF THE 14TH INTERNATIONAL JOINT CONFERENCE ON COMPUTER VISION, IMAGING AND COMPUTER GRAPHICS THEORY AND APPLICATIONS (VISAPP), VOL 5(2019)
摘要
Decision making in automated driving is highly specific to the environment and thus semantic segmentation plays a key role in recognizing the objects in the environment around the car. Pixel level classification once considered a challenging task which is now becoming mature to be productized in a car. However, semantic annotation is time consuming and quite expensive. Synthetic datasets with domain adaptation techniques have been used to alleviate the lack of large annotated datasets. In this work, we explore an alternate approach of leveraging the annotations of other tasks to improve semantic segmentation. Recently, multi-task learning became a popular paradigm in automated driving which demonstrates joint learning of multiple tasks improves overall performance of each tasks. Motivated by this, we use auxiliary tasks like depth estimation to improve the performance of semantic segmentation task. We propose adaptive task loss weighting techniques to address scale issues in multi-task loss functions which become more crucial in auxiliary tasks. We experimented on automotive datasets including SYNTHIA and KITTI and obtained 3% and 5% improvement in accuracy respectively.
更多查看译文
关键词
Semantic Segmentation, Multitask Learning, Auxiliary Tasks, Automated Driving
AI 理解论文
溯源树
样例
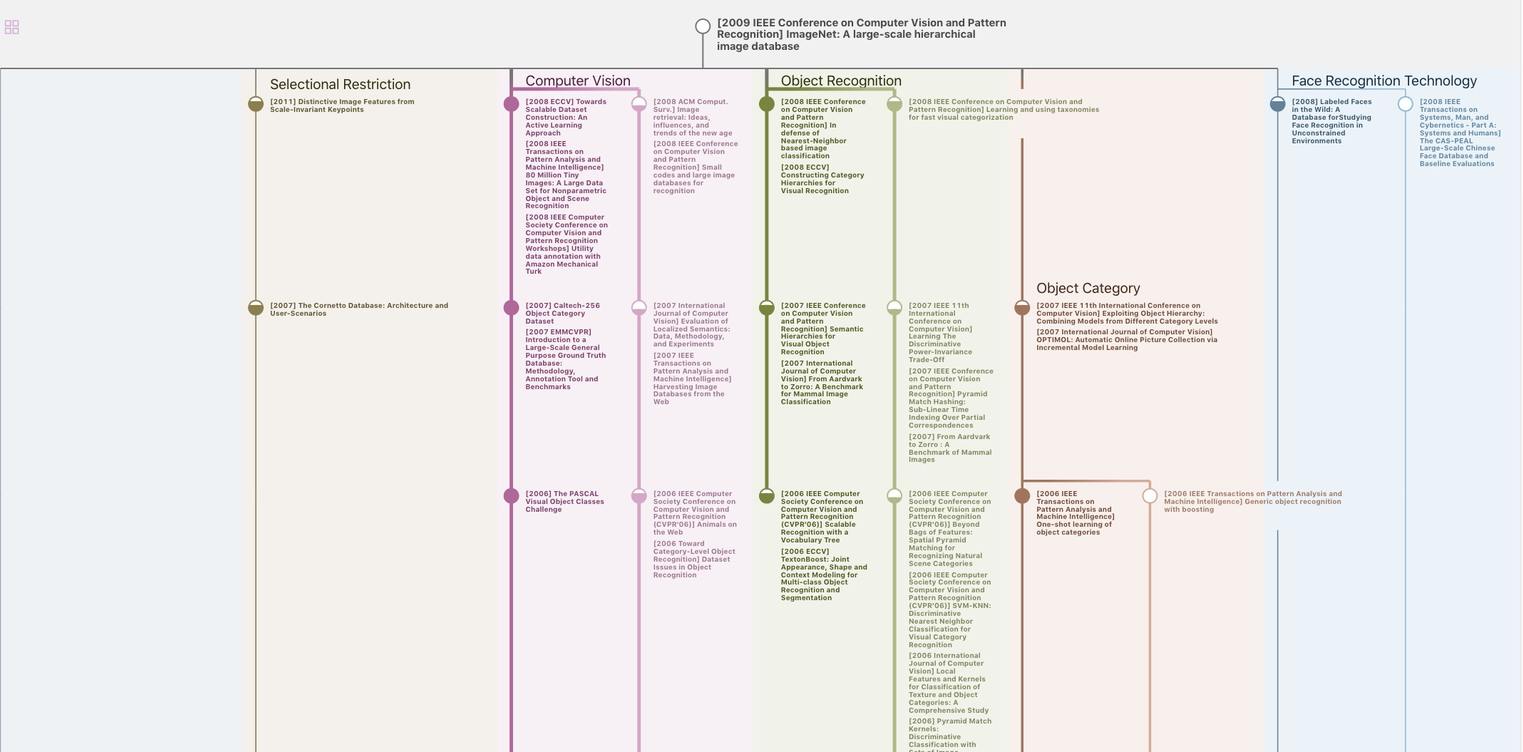
生成溯源树,研究论文发展脉络
Chat Paper
正在生成论文摘要