EAT-NAS: elastic architecture transfer for accelerating large-scale neural architecture search
Science China Information Sciences(2021)
摘要
Neural architecture search (NAS) methods have been proposed to relieve human experts from tedious architecture engineering. However, most current methods are constrained in small-scale search owing to the issue of huge computational resource consumption. Meanwhile, the direct application of architectures searched on small datasets to large datasets often bears no performance guarantee due to the discrepancy between different datasets. This limitation impedes the wide use of NAS on large-scale tasks. To overcome this obstacle, we propose an elastic architecture transfer mechanism for accelerating large-scale NAS (EAT-NAS). In our implementations, the architectures are first searched on a small dataset, e.g., CIFAR-10. The best one is chosen as the basic architecture. The search process on a large dataset, e.g., ImageNet, is initialized with the basic architecture as the seed. The large-scale search process is accelerated with the help of the basic architecture. We propose not only a NAS method but also a mechanism for architecture-level transfer learning. In our experiments, we obtain two final models EATNet-A and EATNet-B, which achieve competitive accuracies of 75.5% and 75.6%, respectively, on ImageNet. Both the models also surpass the models searched from scratch on ImageNet under the same settings. For the computational cost, EAT-NAS takes only fewer than 5 days using 8 TITAN X GPUs, which is significantly less than the computational consumption of the state-of-the-art large-scale NAS methods.
更多查看译文
关键词
architecture transfer,neural architecture search,evolutionary algorithm,large-scale dataset
AI 理解论文
溯源树
样例
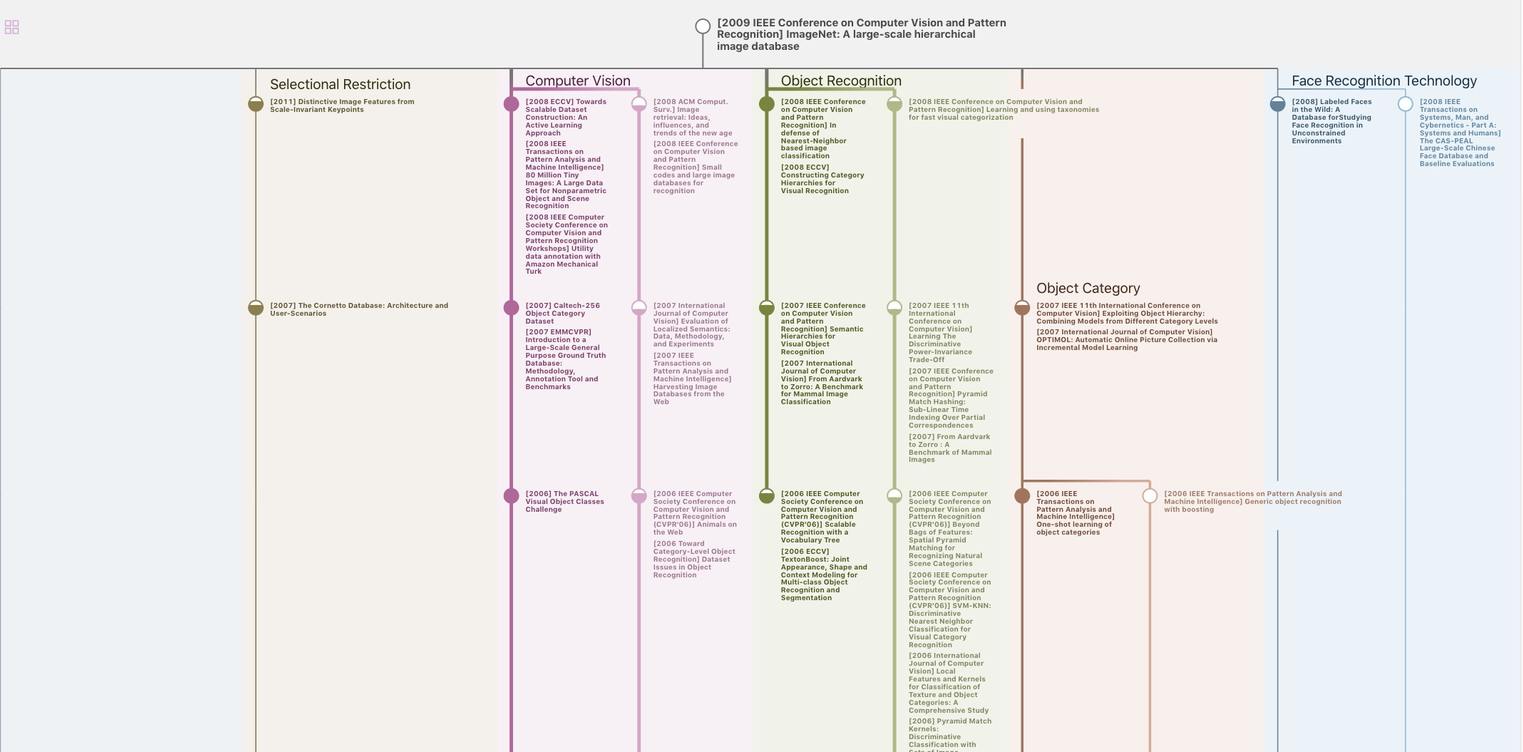
生成溯源树,研究论文发展脉络
Chat Paper
正在生成论文摘要