CREAM: CNN-REgularized ADMM Framework for Compressive-Sensed Image Reconstruction
IEEE ACCESS(2018)
摘要
Compressive sensing (CS) has drawn an enormous amount of attention in recent years due to its sub-Nyquist sampling rate and low-complexity requirement at the encoder. However, it turns out that the decoder in lieu of the encoder suffers from heavy computation in order to decently recover the signal from its CS measurements. With the aim of developing a fast yet accurate algorithm, in this paper, we propose to leverage a deep convolutional neural network (CNN) prior model to the constrained CS reconstruction formulation and solve it via the alternating direction method of multipliers (ADMM). The proposed CNN-REgularized ADMM framework, dubbed CREAM, is able to recover image signals from CS measurements effectively and efficiently. On the one hand, the developed constrained CS formulation by CREAM enables fewer regularization parameters and less computational complexity compared with traditional unconstrained CS formulation by ADMM. On the other hand, rather than training a neural network from scratch, an off-the-shelf CNN model is directly incorporated into CREAM even without the effort of fine tuning, in which CNN has exhibited its desirable reconstruction performance and low computational complexity. Hereby, powerful GPU can be utilized to speed up the reconstruction. Experiments demonstrate that our proposed method for CS reconstruction of natural images surpasses state-of-the-art CS models by a significant margin in speed and performance.
更多查看译文
关键词
Compressive sensing,deep learning,alternate direction method of multipliers (ADMM),convolutional neural network (CNN),image reconstruction
AI 理解论文
溯源树
样例
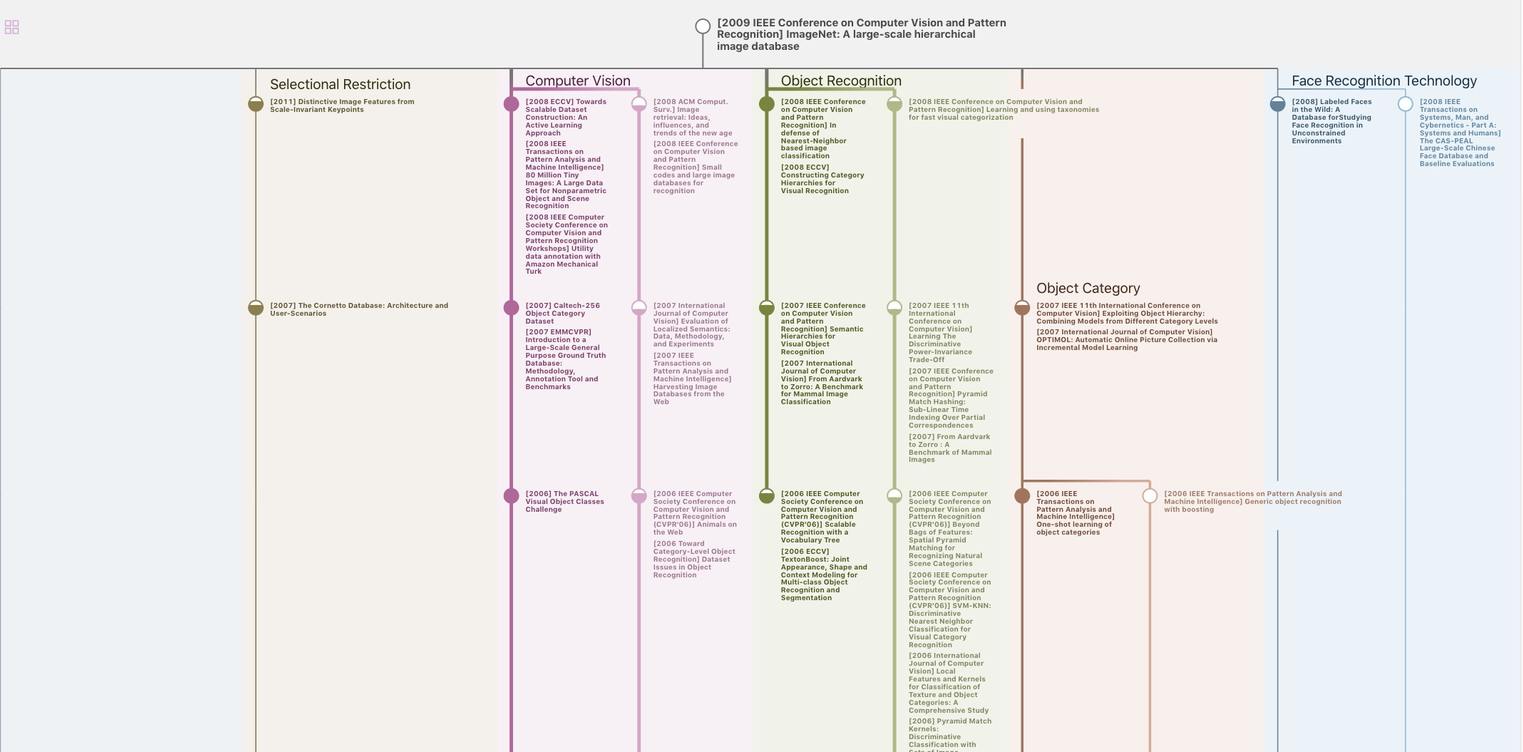
生成溯源树,研究论文发展脉络
Chat Paper
正在生成论文摘要