L21-Norm Based Loss Function and Regularization Extreme Learning Machine.
IEEE ACCESS(2019)
摘要
Extreme learning machine (ELM) has gained increasing interests from various research fields recently. Researchers have proposed various extensions to improve its stability, sparsity, and generalization performance. In this paper, we propose a robust and sparse ELM to exploit L-21-norm minimization of both loss function and regularization (LR21-ELM). Our L-21-norm-based loss function can diminish the undue influence of noises and outliers of data points compared with the L-2-norm based loss function and make the learned ELM model more robust and stable. The powerful structural sparse-inducing L-21-norm regularization is integrated into the ELM objective function to eliminate the potential redundant neurons of ELM adaptively and reduce the complexity of the learning model. We introduce an effective iterative optimization algorithm to solve the L-21-norm minimization problem. Empirical tests on a number of benchmark datasets indicate that our proposed algorithm can generate a more compact, robust, and discriminative model compared with the original ELM algorithm.
更多查看译文
关键词
Extreme learning machine,L-21-norm loss,L-21-norm regularization,robustness,sparsity
AI 理解论文
溯源树
样例
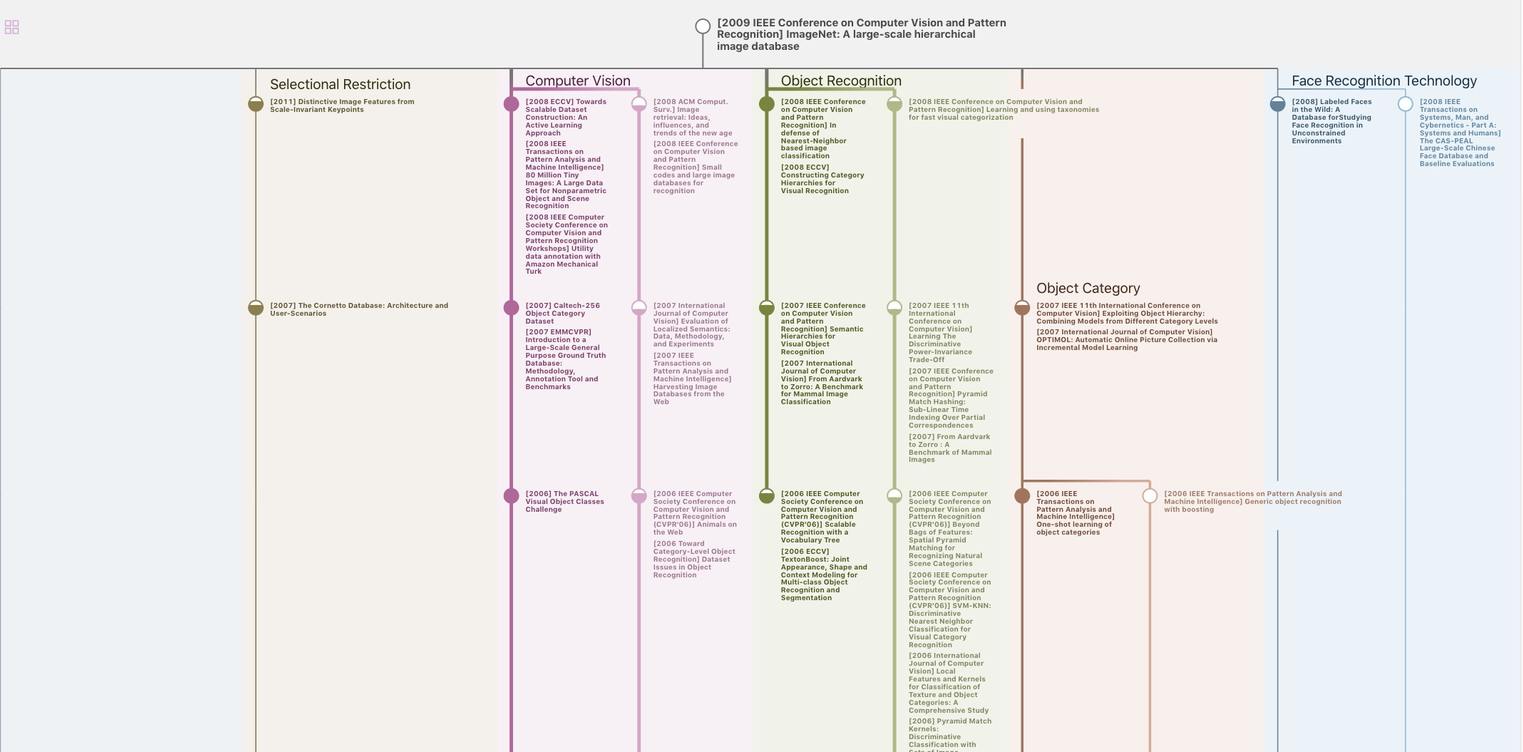
生成溯源树,研究论文发展脉络
Chat Paper
正在生成论文摘要