ST-DenNetFus: A New Deep Learning Approach for Network Demand Prediction
MACHINE LEARNING AND KNOWLEDGE DISCOVERY IN DATABASES, ECML PKDD 2018, PT III(2019)
摘要
Network Demand Prediction is of great importance to network planning and dynamically allocating network resources based on the predicted demand, this can be very challenging as it is affected by many complex factors, including spatial dependencies, temporal dependencies, and external factors (such as regions' functionality and crowd patterns as it will be shown in this paper). We propose a deep learning based approach called, ST-DenNetFus, to predict network demand (i.e. uplink and downlink throughput) in every region of a city. ST-DenNetFus is an end to end architecture for capturing unique properties from spatiotemporal data. ST-DenNetFus employs various branches of dense neural networks for capturing temporal closeness, period, and trend properties. For each of these properties, dense convolutional neural units are used for capturing the spatial properties of the network demand across various regions in a city. Furthermore, ST-DenNetFus introduces extra branches for fusing external data sources that have not been considered before in the network demand prediction problem of various dimensionalities. In our case, these external factors are the crowd mobility patterns, temporal functional regions, and the day of the week. We present an extensive experimental evaluation for the proposed approach using two types of network throughput (uplink and downlink) in New York City (NYC), where ST-DenNetFus outperforms four well-known baselines.
更多查看译文
关键词
Spatio-temporal data,Deep learning,Convolutional neural networks,Dense networks,Network demand prediction
AI 理解论文
溯源树
样例
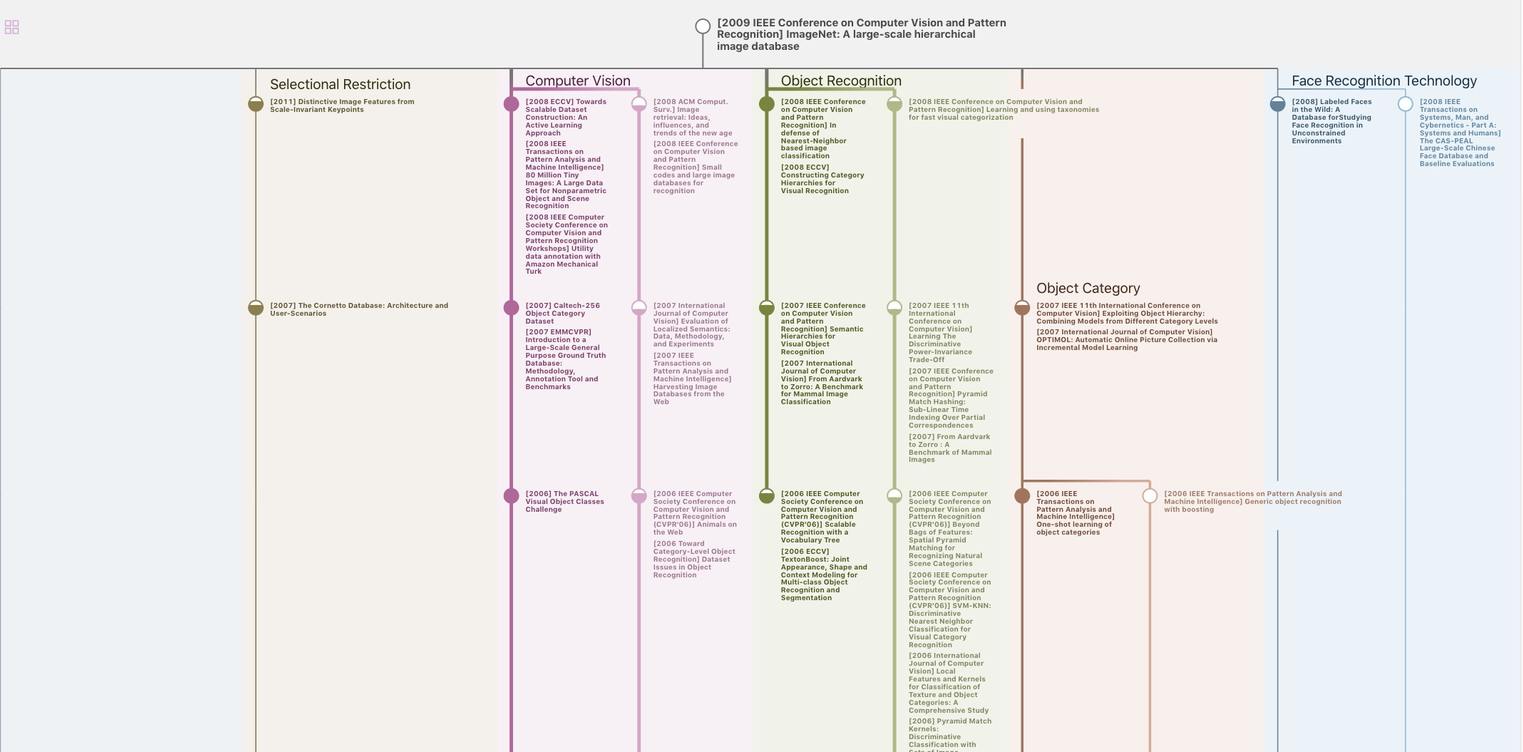
生成溯源树,研究论文发展脉络
Chat Paper
正在生成论文摘要