SHNE - Representation Learning for Semantic-Associated Heterogeneous Networks.
WSDM(2019)
摘要
Representation learning in heterogeneous networks faces challenges due to heterogeneous structural information of multiple types of nodes and relations, and also due to the unstructured attribute or content (e.g., text) associated with some types of nodes. While many recent works have studied homogeneous, heterogeneous, and attributed networks embedding, there are few works that have collectively solved these challenges in heterogeneous networks. In this paper, we address them by developing a Semantic-aware Heterogeneous Network Embedding model (SHNE). SHNE performs joint optimization of heterogeneous SkipGram and deep semantic encoding for capturing both heterogeneous structural closeness and unstructured semantic relations among all nodes, as function of node content, that exist in the network. Extensive experiments demonstrate that SHNE outperforms state-of-the-art baselines in various heterogeneous network mining tasks, such as link prediction, document retrieval, node recommendation, relevance search, and class visualization.
更多查看译文
关键词
deep learning, network embedding, representation learning, semantic-associated heterogeneous networks
AI 理解论文
溯源树
样例
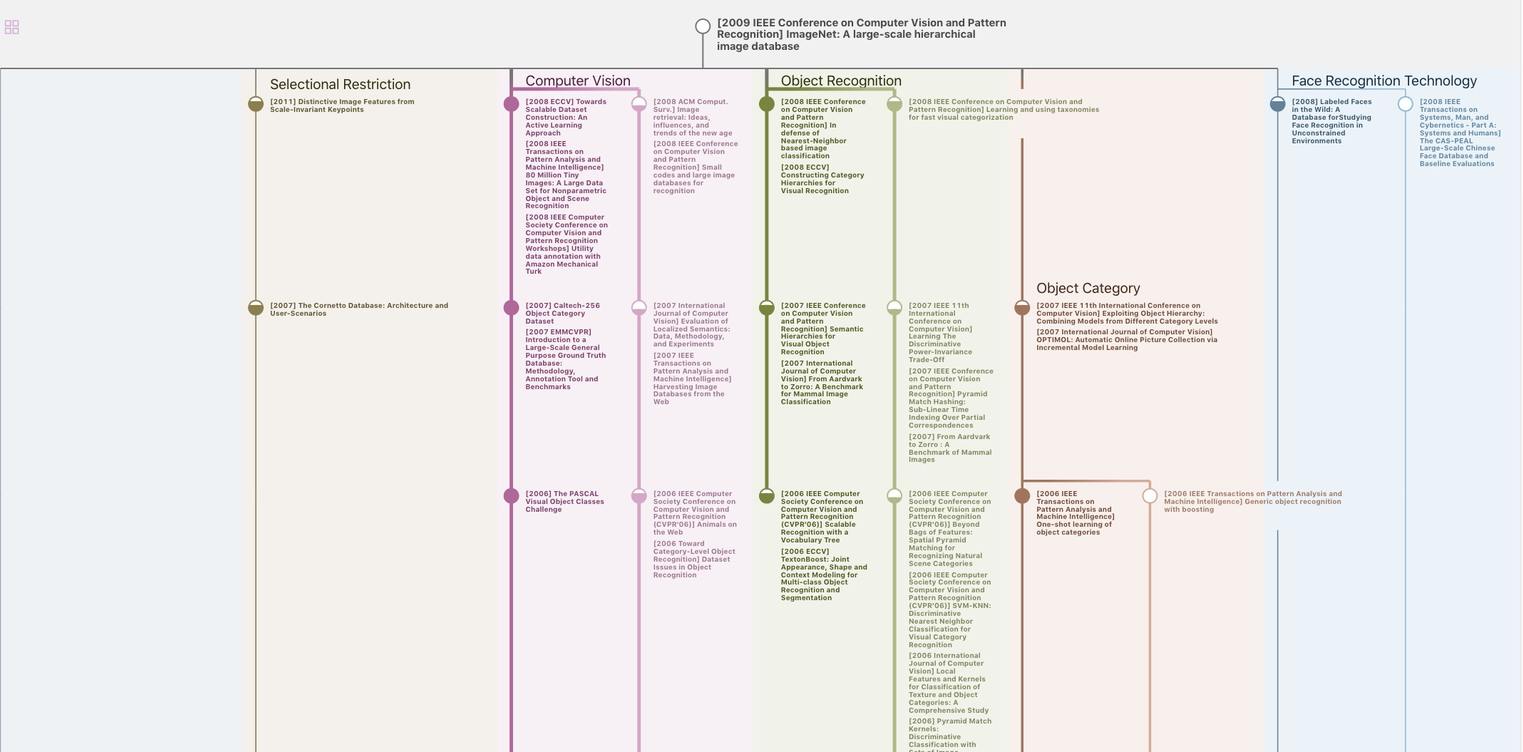
生成溯源树,研究论文发展脉络
Chat Paper
正在生成论文摘要