When People Change their Mind - Off-Policy Evaluation in Non-stationary Recommendation Environments.
WSDM(2019)
摘要
We consider the novel problem of evaluating a recommendation policy offline in environments where the reward signal is non-stationary. Non-stationarity appears in many Information Retrieval (IR) applications such as recommendation and advertising, but its effect on off-policy evaluation has not been studied at all. We are the first to address this issue. First, we analyze standard off-policy estimators in non-stationary environments and show both theoretically and experimentally that their bias grows with time. Then, we propose new off-policy estimators with moving averages and show that their bias is independent of time and can be bounded. Furthermore, we provide a method to trade-off bias and variance in a principled way to get an off-policy estimator that works well in both non-stationary and stationary environments. We experiment on publicly available recommendation datasets and show that our newly proposed moving average estimators accurately capture changes in non-stationary environments, while standard off-policy estimators fail to do so.
更多查看译文
关键词
non-stationary rewards, off-policy evaluation
AI 理解论文
溯源树
样例
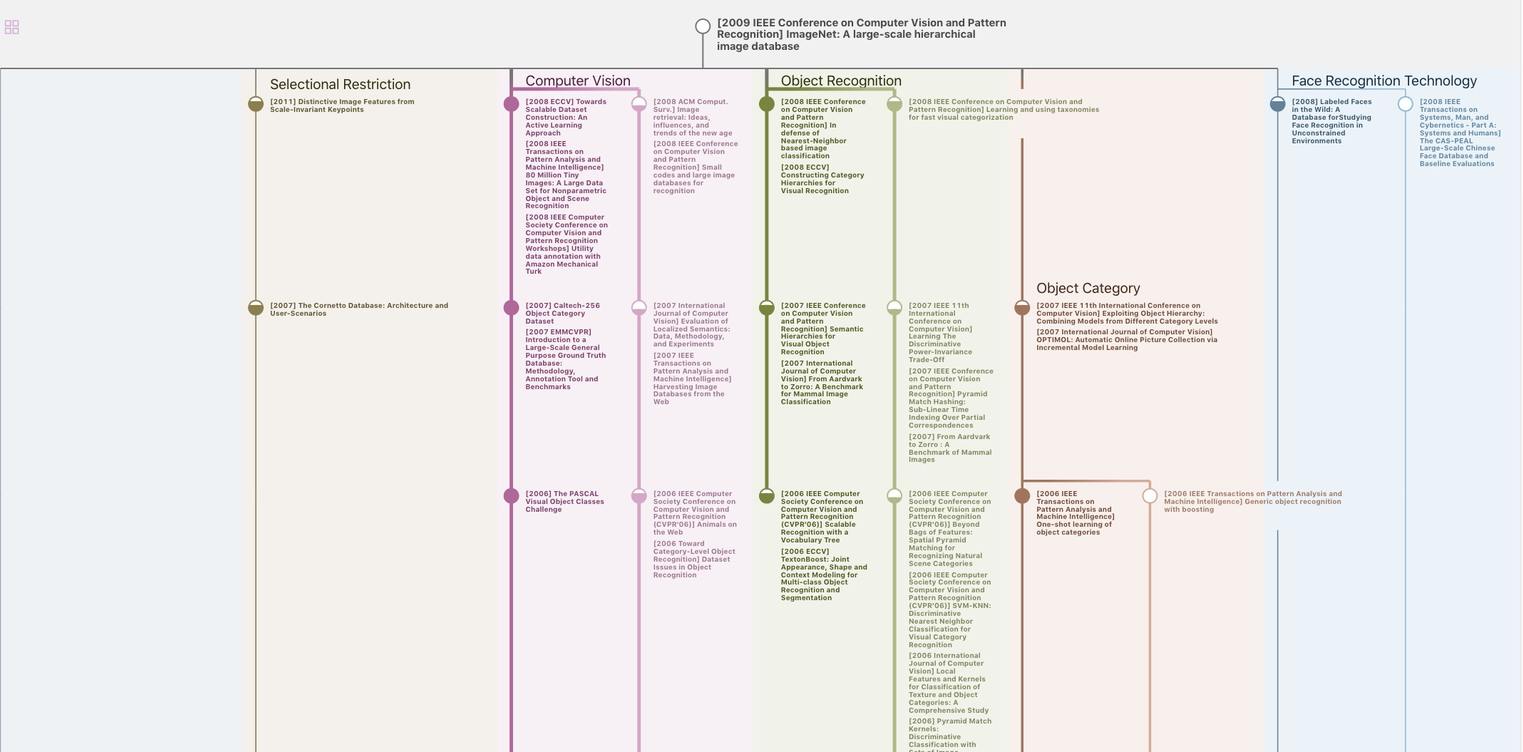
生成溯源树,研究论文发展脉络
Chat Paper
正在生成论文摘要