Mining Sensor Data for Predictive Maintenance in the Automotive Industry.
DSAA(2018)
摘要
Predictive maintenance is an ever-growing area of interest, spanning different fields and approaches. In the automotive industry faulty behaviors of the oxygen sensor are a key challenge to address. This paper presents OxyClog, a data-driven framework that, given a large number of time series collected from a vehicle's ECU (engine control unit), builds a model to predict if the oxygen sensor is currently unclogged, almost clogged (since the clogging of the sensor happens gradually), or clogged. OxyClog is characterized by a tailored preprocessing, which includes a custom and interpretable feature selection algorithm, along with a summarization strategy to transform a time-dependent problem into a time-independent one. Furthermore, a semi-supervised labeling methodology has been devised to use different data sources with different characteristics to define meaningful clogging labels. OxyClog integrates state-of-the-art classification algorithms – both interpretable and non-interpretable – to process real ECU data with good prediction performance.
更多查看译文
关键词
Predictive models,Engines,Monitoring,Prediction algorithms,Predictive maintenance,Feature extraction,Automotive engineering
AI 理解论文
溯源树
样例
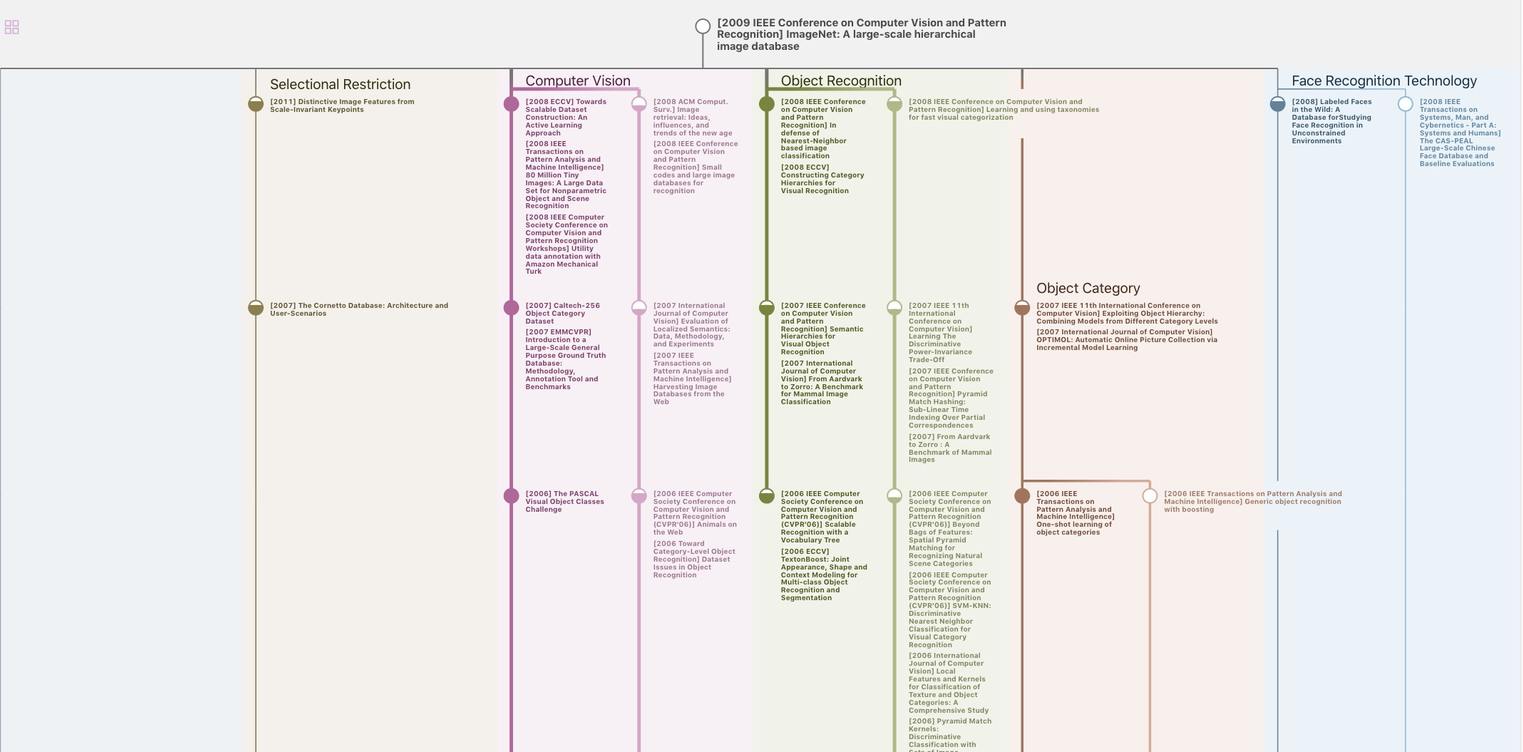
生成溯源树,研究论文发展脉络
Chat Paper
正在生成论文摘要