Deep Multi-Feature Learning for Water Body Extraction from Landsat Imagery
Automatic Control and Computer Sciences(2019)
摘要
Water body extraction from remote sensing image data has been becoming a really hot topic. Recently, researchers put forward numerous methods for water body extraction, while most of them rely on elaborative feature selection and enough number of training samples. Convolution Neural Network (CNN), one of the implementation models of deep learning, has strong capability for two-dimension images’ classification. A new water body extraction model based on CNNs is established for deep multi-feature learning. Before experiment, image enhancement will be done by Dark Channel Prior. Then we concatenate three kinds of features: spectral information, spatial information that is extracted by Extended Multi-attribute Profile (EMAP) and various water indexes firstly. Next, feature matrixes are acted as the input of CNN-based model for training and classifying. The experimental results showed that the proposed model has better classification performance than Support Vector Machine (SVM) and artificial neural network (ANN). On very limited training set, our model could learn unique and representative features for better water body extraction.
更多查看译文
关键词
deep learning,convolution neural network,water information extraction,EMAPs,feature extraction,deep multi-feature learning
AI 理解论文
溯源树
样例
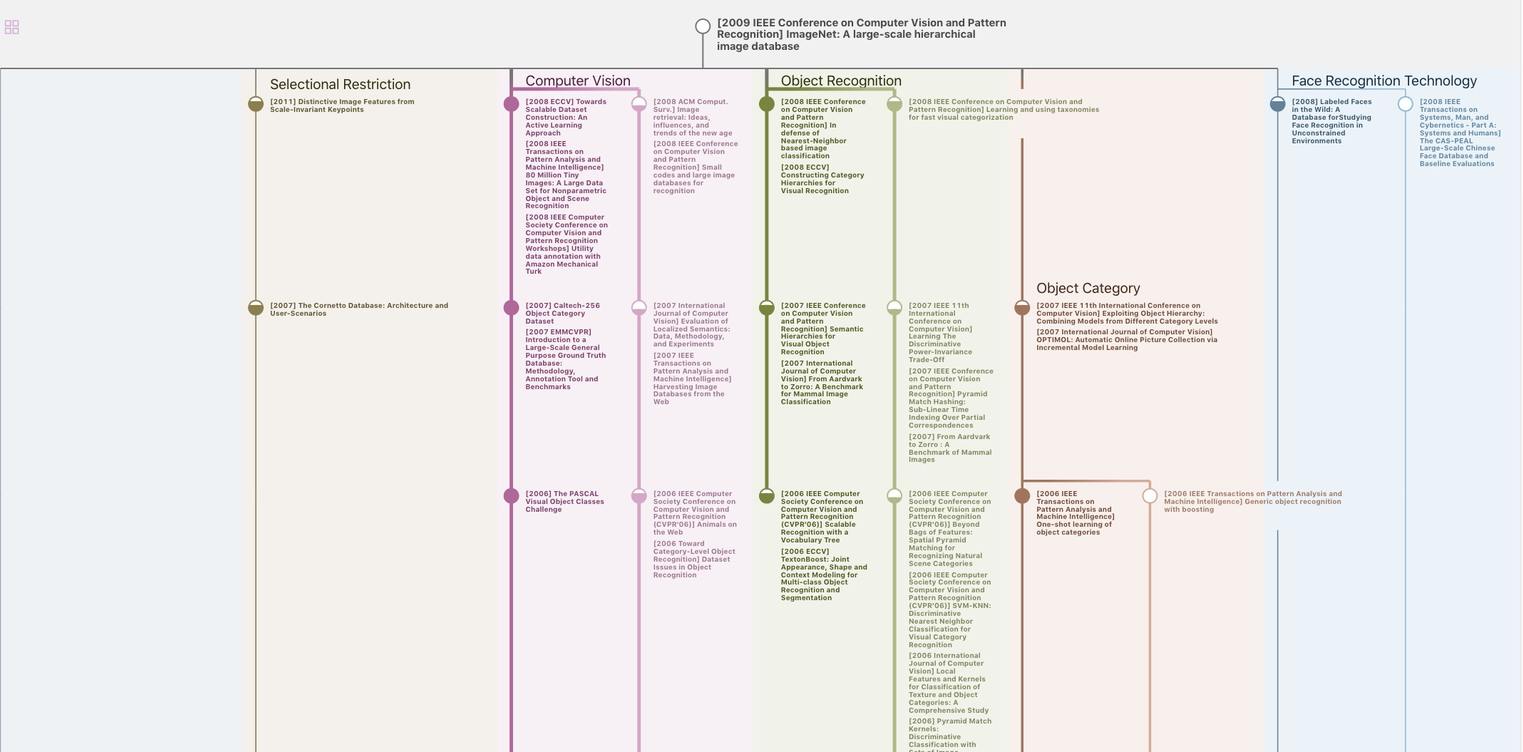
生成溯源树,研究论文发展脉络
Chat Paper
正在生成论文摘要