Improving large-scale hierarchical classification by rewiring: a data-driven filter based approach
J. Intell. Inf. Syst.(2018)
摘要
Hierarchical Classification (HC) is a supervised learning problem where unlabeled instances are classified into a taxonomy of classes. Several methods that utilize the hierarchical structure have been developed to improve the HC performance. However, in most cases apriori defined hierarchical structure by domain experts is inconsistent; as a consequence performance improvement is not noticeable in comparison to flat classification methods. We propose a scalable data-driven filter based rewiring approach to modify an expert-defined hierarchy. Experimental comparisons of top-down hierarchical classification with our modified hierarchy, on a wide range of datasets shows classification performance improvement over the baseline hierarchy ( i . e ., defined by expert), clustered hierarchy and flattening based hierarchy modification approaches. In comparison to existing rewiring approaches, our developed method ( r e w H i e r ) is computationally efficient, enabling it to scale to datasets with large numbers of classes, instances and features. We also show that our modified hierarchy leads to improved classification performance for classes with few training samples in comparison to flat and state-of-the-art hierarchical classification approaches. Source Code: https://cs.gmu.edu/~mlbio/TaxMod/
更多查看译文
关键词
Top-down hierarchical classification, Inconsistency, Error propagation, Flattening, Clustering, Rewiring
AI 理解论文
溯源树
样例
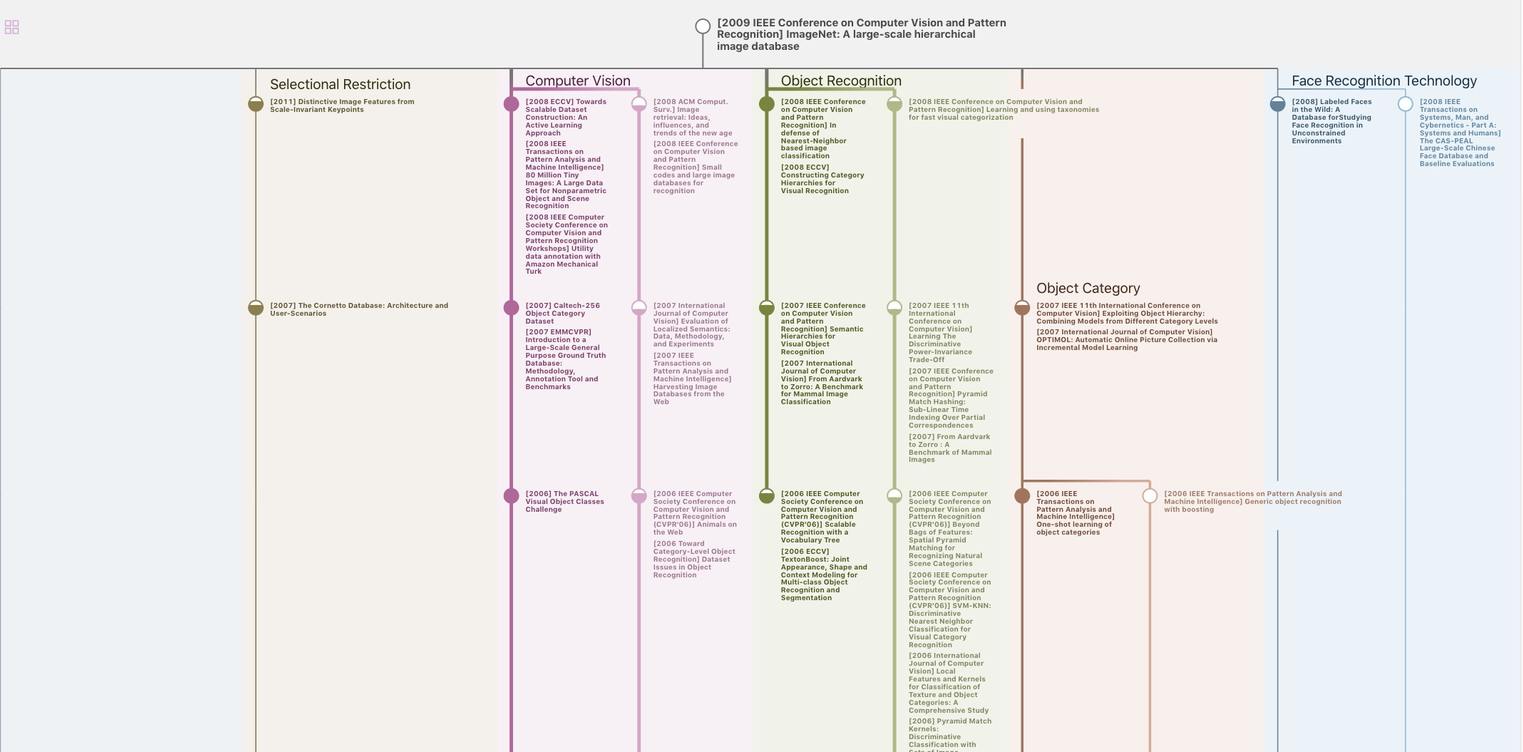
生成溯源树,研究论文发展脉络
Chat Paper
正在生成论文摘要