Document-based and term-based linear methods for pseudo-relevance feedback
ACM SIGAPP Applied Computing Review(2019)
摘要
Query expansion is a successful approach for improving Information Retrieval effectiveness. This work focuses on pseudo-relevance feedback (PRF) which provides an automatic method for expanding queries without explicit user feedback. These techniques perform an initial retrieval with the original query and select expansion terms from the top retrieved documents. We propose two linear methods for pseudo-relevance feedback, one document-based and another term-based, that models the PRF task as a matrix decomposition problem. These factorizations involve the computation of an inter-document or inter-term similarity matrix which is used for expanding the original query. These decompositions can be computed by solving a least squares regression problem with regularization and a non-negativity constraint. We evaluate our proposals on five collections against state-of-the-art baselines. We found that the term-based formulation provides high figures of MAP, nDCG and robustness index whereas the document-based formulation provides very cheap computation at the cost of a slight decrease in effectiveness.
更多查看译文
关键词
information retrieval, linear least squares, linear methods, pseudo-relevance feedback, query expansion
AI 理解论文
溯源树
样例
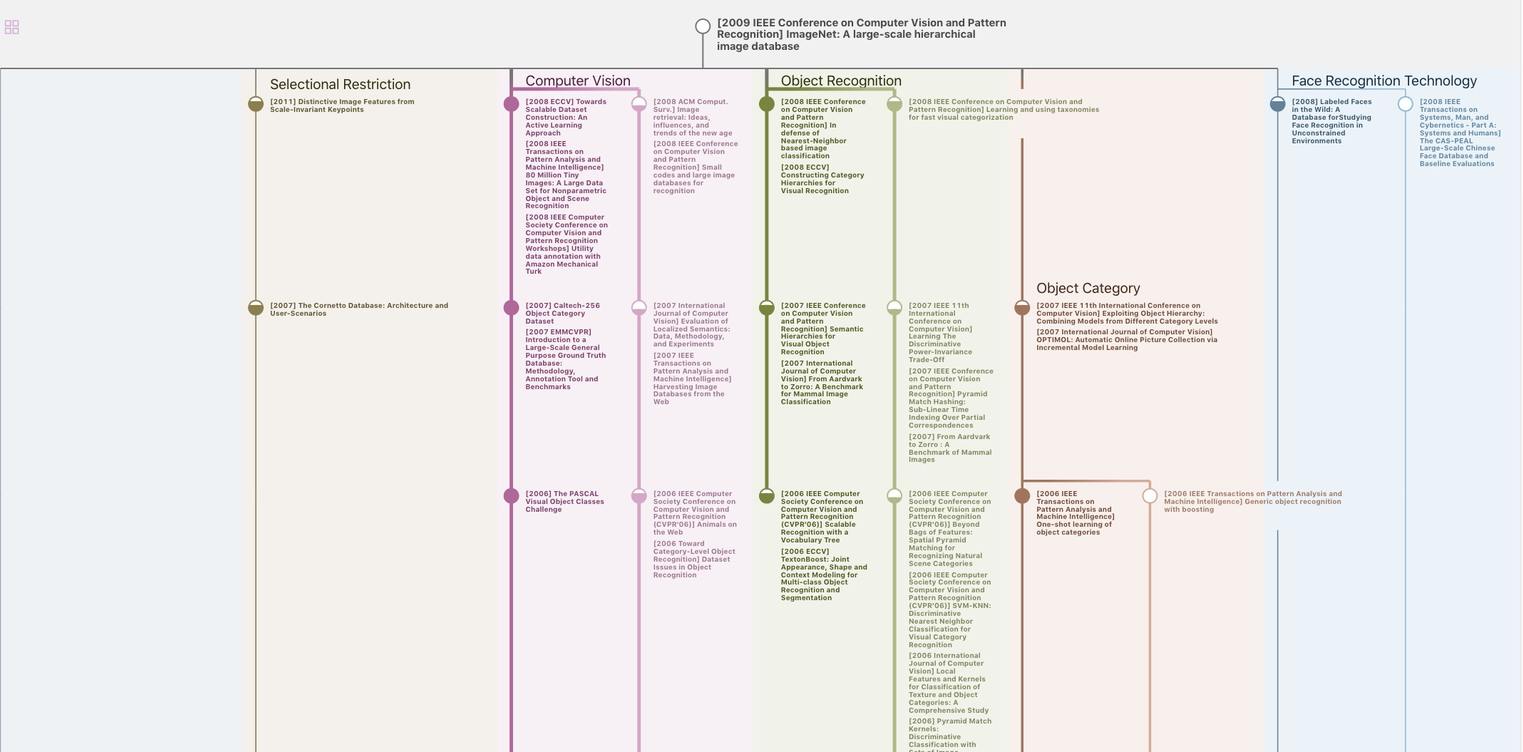
生成溯源树,研究论文发展脉络
Chat Paper
正在生成论文摘要