The Phylogenetic Tree based Deep Forest for Metagenomic Data Classification
2018 IEEE International Conference on Bioinformatics and Biomedicine (BIBM)(2018)
摘要
Microorganisms are closely related to human health and have an impact on the development of various diseases. It is extremely significant to identify the relationships between microorganisms and the phenotypes (such as healthy or disease status) by analyzing microbial abundance in personalized medicine. Deep learning allows computational models that composed of multiple processing layers to learn representation of data with multiple levels of abstraction. These methods have improved the state-of-the-art in speech recognition, visual object recognition and object detection. However, current deep models are typically neural networks which are actually multiple layers of parameterized differentiable nonlinear models that can be trained by backpropagation. It is interesting to explore other deep learning models to handle tasks with small sample size and high dimensional data. While a unique feature of microbial data is that it has phylogenetic tree structure information which can be embedded to improve the classification performance. In this work, in order to further improve the metagenomic classification, we propose a deep model named Cascade Deep Forest which keeps the spatial structure between nodes through embedding phylogenetic tree information. Our results demonstrate: 1) the modified cascade structure can enhance the classification performance of Deep Forest; 2) embedding phylogenetic tree information can also improve the classification of the models; 3) Deep Forest achieves highly competitive performance to deep neural networks.
更多查看译文
关键词
phylogenetic tree based deep forest,cascade deep forest,backpropagation,human health,metagenomic data classification,deep neural networks,modified cascade structure,phylogenetic tree structure information,microbial data,deep learning models,parameterized differentiable nonlinear models,object detection,visual object recognition,speech recognition,computational models,personalized medicine,microbial abundance,microorganisms
AI 理解论文
溯源树
样例
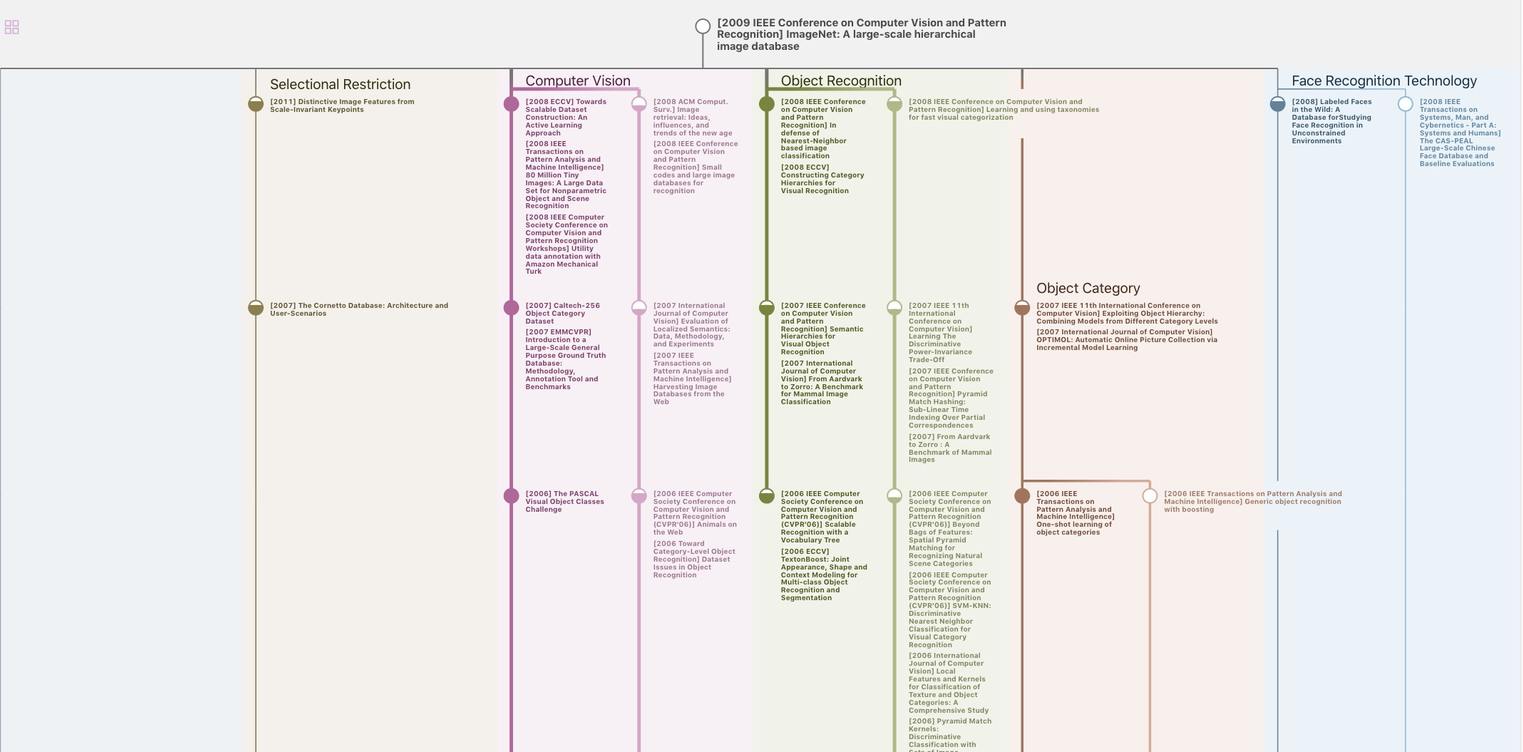
生成溯源树,研究论文发展脉络
Chat Paper
正在生成论文摘要