An Efficient Survival Multifactor Dimensionality Reduction Method for Detecting Gene-Gene Interactions of Lung Cancer Onset Age.
BIBM(2018)
摘要
This study addresses the computational burden often encountered when analyzing gene-gene interactions in relation to time-to-event data, such as patient survival time or time-to-cancer relapse. The goal is to develop a method called Efficient Survival MDR (ES-MDR) that handles survival data by using Martingale Residuals to replace the survival outcome and uses the computationally efficient Quantitative MDR (QMDR) to identify significant interaction models. To demonstrate the strength of ES-MDR, two simulations are designed to evaluate the testing score’s null distribution and to study the success rate of the method. Additionally, ES-MDR is applied on real data with 14,935cases and 12,787 controls of European descent from the OncoArray Consortium that examined the relationship between genetic variants and lung cancer susceptibility. Martingale Residuals, which replace onset age of lung cancer, is treated as the survival outcome, cases are considered event at diagnosis age, and controls are considered censored at interview age. Froman exhaustive search over all one-way and two-way interaction models, we identified a strong association with chr17_41196821_INDEL_T_Dfrom BRCA1 gene and exm1568790_Afrom CBR1 gene as the top SNP-SNP interaction with lung cancer susceptibility at age-of-onset.
更多查看译文
关键词
gene-gene interactions, machine learning, data mining, lung cancer
AI 理解论文
溯源树
样例
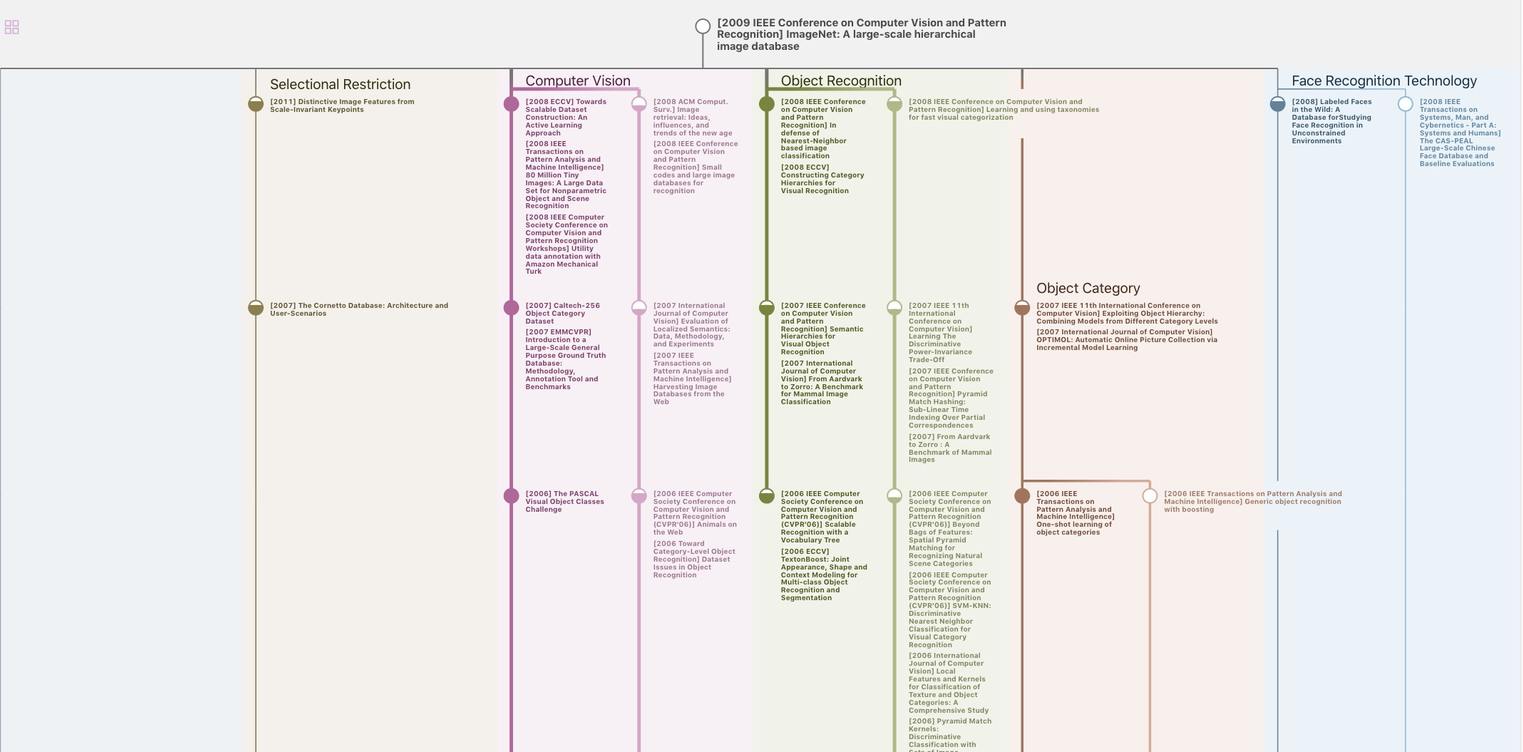
生成溯源树,研究论文发展脉络
Chat Paper
正在生成论文摘要