Machine Learning Models for Multiparametric Glioma Grading With Quantitative Result Interpretations.
FRONTIERS IN NEUROSCIENCE(2019)
摘要
Gliomas are the most common primary malignant brain tumors in adults. Accurate grading is crucial as therapeutic strategies are often disparate for different grades and may influence patient prognosis. This study aims to provide an automated glioma grading platform on the basis of machine learning models. In this paper, we investigate contributions of multi-parameters from multimodal data including imaging parameters or features from the Whole Slide images (WSI) and the proliferation marker Ki-67 for automated brain tumor grading. For each WSI, we extract both visual parameters such as morphology parameters and sub-visual parameters including first-order and second-order features. On the basis of machine learning models, our platform classifies gliomas into grades II, III, and IV. Furthermore, we quantitatively interpret and reveal the important parameters contributing to grading with the Local Interpretable Model-Agnostic Explanations (LIME) algorithm. The quantitative analysis and explanation may assist clinicians to better understand the disease and accordingly to choose optimal treatments for improving clinical outcomes. The performance of our grading model was evaluated with cross-validation, which randomly divided the patients into non-overlapping training and testing sets and repeatedly validated the model on the different testing sets. The primary results indicated that this modular platform approach achieved the highest grading accuracy of 0.90 +/- 0.04 with support vector machine (SVM) algorithm, with grading accuracies of 0.91 +/- 0.08, 0.90 +/- 0.08, and 0.90 +/- 0.07 for grade II, III, and IV gliomas, respectively.
更多查看译文
关键词
glioma grading,machine learning,morphological features,support vector machine,digital pathology images
AI 理解论文
溯源树
样例
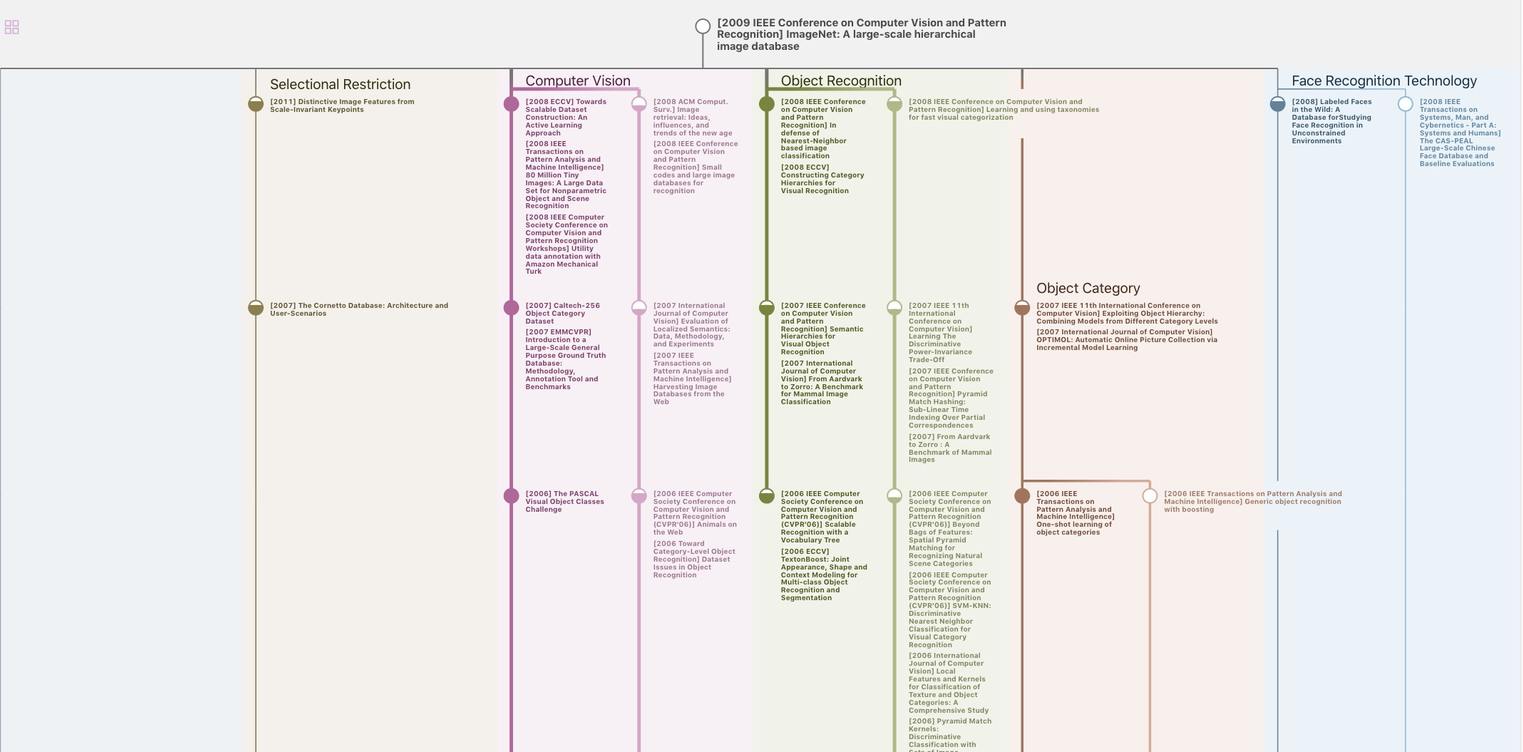
生成溯源树,研究论文发展脉络
Chat Paper
正在生成论文摘要