Sliced inverse regression for integrative multi-omics data analysis.
STATISTICAL APPLICATIONS IN GENETICS AND MOLECULAR BIOLOGY(2019)
摘要
Advancement in next-generation sequencing, transcriptomics, proteomics and other high-throughput technologies has enabled simultaneous measurement of multiple types of genomic data for cancer samples. These data together may reveal new biological insights as compared to analyzing one single genome type data. This study proposes a novel use of supervised dimension reduction method, called sliced inverse regression, to multi-omics data analysis to improve prediction over a single data type analysis. The study further proposes an integrative sliced inverse regression method (integrative SIR) for simultaneous analysis of multiple omics data types of cancer samples, including MiRNA, MRNA and proteomics, to achieve integrative dimension reduction and to further improve prediction performance. Numerical results show that integrative analysis of multi-omics data is beneficial as compared to single data source analysis, and more importantly, that supervised dimension reduction methods possess advantages in integrative data analysis in terms of classification and prediction as compared to unsupervised dimension reduction methods.
更多查看译文
关键词
Integrative genomic analysis,sliced inverse regression,sufficient dimension reduction
AI 理解论文
溯源树
样例
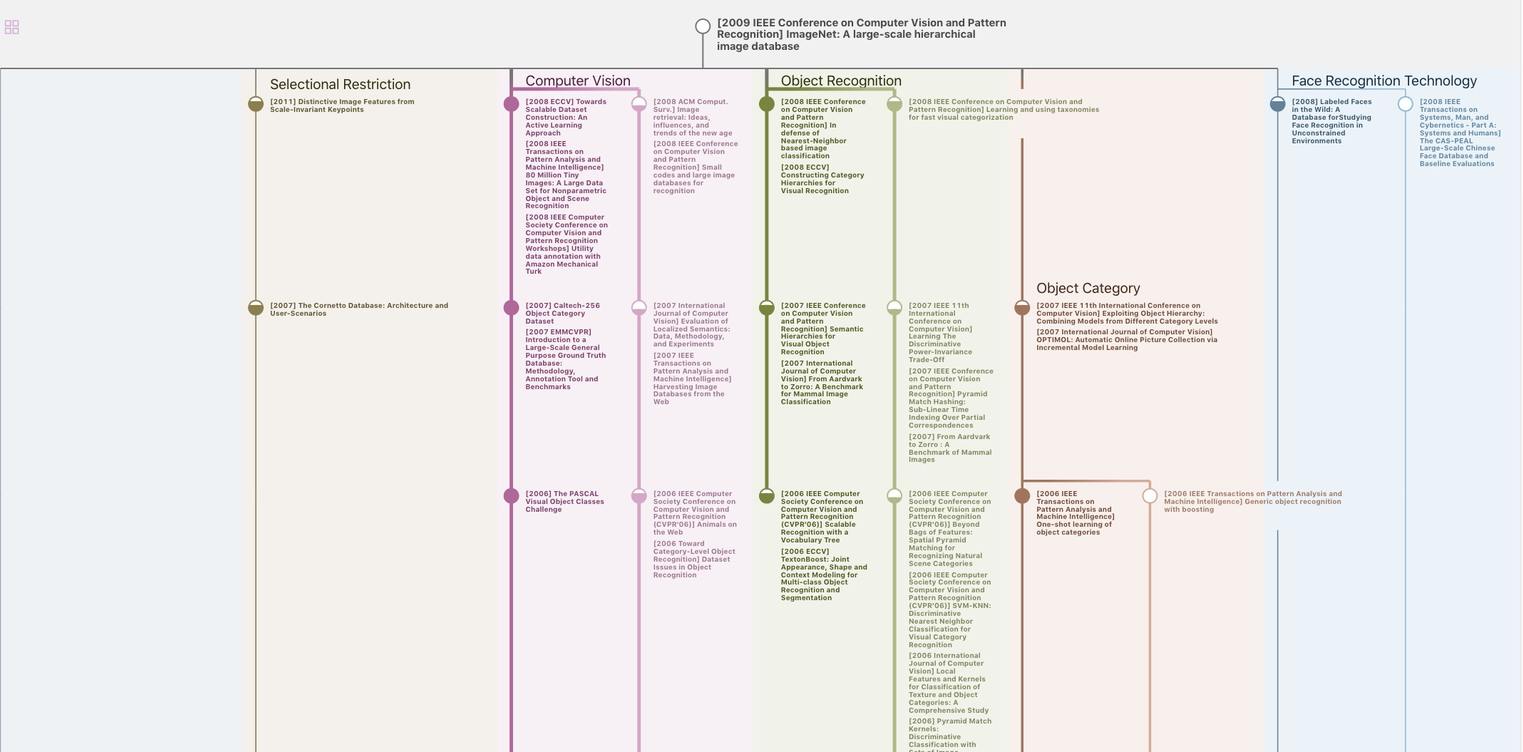
生成溯源树,研究论文发展脉络
Chat Paper
正在生成论文摘要