Tunable Floating-Point for Artificial Neural Networks
2018 25th IEEE International Conference on Electronics, Circuits and Systems (ICECS)(2018)
摘要
Approximate computing has emerged as a promising approach to energy-efficient design of digital systems in many domains such as digital signal processing, robotics, and machine learning. Numerous studies report that employing different data formats in Deep Neural Networks (DNNs), the dominant Machine Learning approach, could allow substantial improvements in power efficiency considering an acceptable quality for results. In this work, the application of Tunable Floating-Point (TFP) precision to DNN is presented. In TFP different precisions for different operations can be set by selecting a specific number of bits for significant and exponent in the floating-point representation. Flexibility in tuning the precision of given layers of the neural network may result in a more power efficient computation.
更多查看译文
关键词
Floating-point,power efficiency,neural networks
AI 理解论文
溯源树
样例
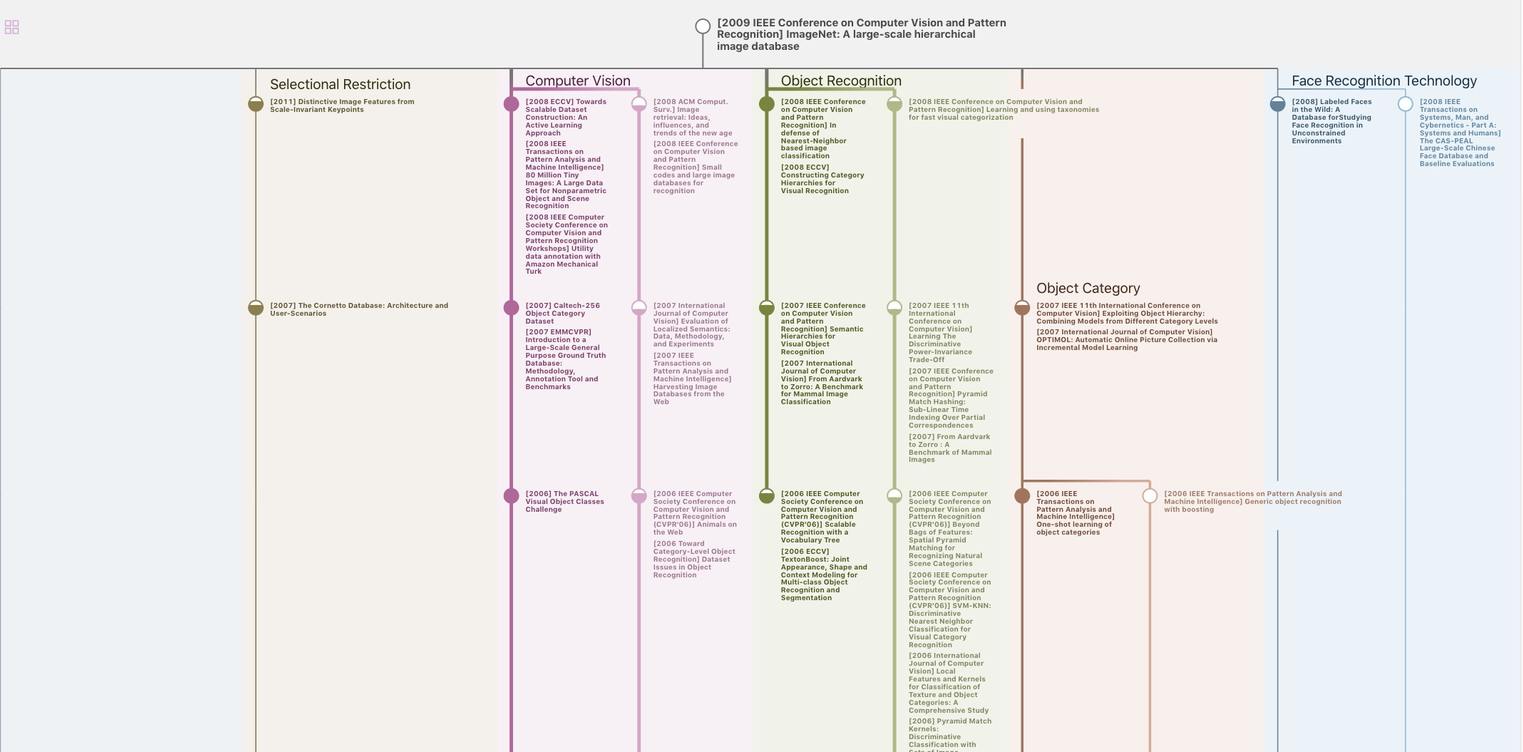
生成溯源树,研究论文发展脉络
Chat Paper
正在生成论文摘要