Listwise Click-Through Rate Prediction with Item-Item Interactions
2018 IEEE INTERNATIONAL CONFERENCE ON SYSTEMS, MAN, AND CYBERNETICS (SMC)(2018)
摘要
Predicting the click-through rates (CTR) of items for a specific user, is of great importance to recommender systems. In most cases, recommendation items are presented by an ordered list. Most previous studies, however, focus on the CTR prediction for a user-item pair, and ignore the interactions among the items in the list. Through our study, with different preceding items, the CTRs of the same active item become significantly different. It implies if this kind of dependency is not considered, the CTR prediction will not be accurate enough in practice. To solve this limitation, we investigate the listwise user-specific CTR prediction for items in the whole recommendation list, rather than for a single item. Specifically, a joint prediction framework based on matrix factorization (MF) and recurrent neural network (RNN) is proposed, which exploits the features of item-item interactions for a user, as well as the features of a single user-item pair. In the process of list generation, our model can improve the CTR prediction for the trailing items, with considering the determined preceding items. We evaluate the proposed approach in a real news recommendation scenario from industry. Compared with a competitive baseline, the performance has been improved by 0.64% in the AUC metric from the magnitude of 0.7357, and by 4.29% in the RIG metric from the magnitude of 0.1095. The improvement on specific position is up to 1.21% and 9.44% in the metric of AUC and RIG, respectively.
更多查看译文
关键词
listwise click-through rate prediction,item-item interactions,click-through rates,recommendation items,joint prediction framework,single user-item pair,user-specific CTR prediction,matrix factorization,recurrent neural network,RNN,AUC metric,recommender systems
AI 理解论文
溯源树
样例
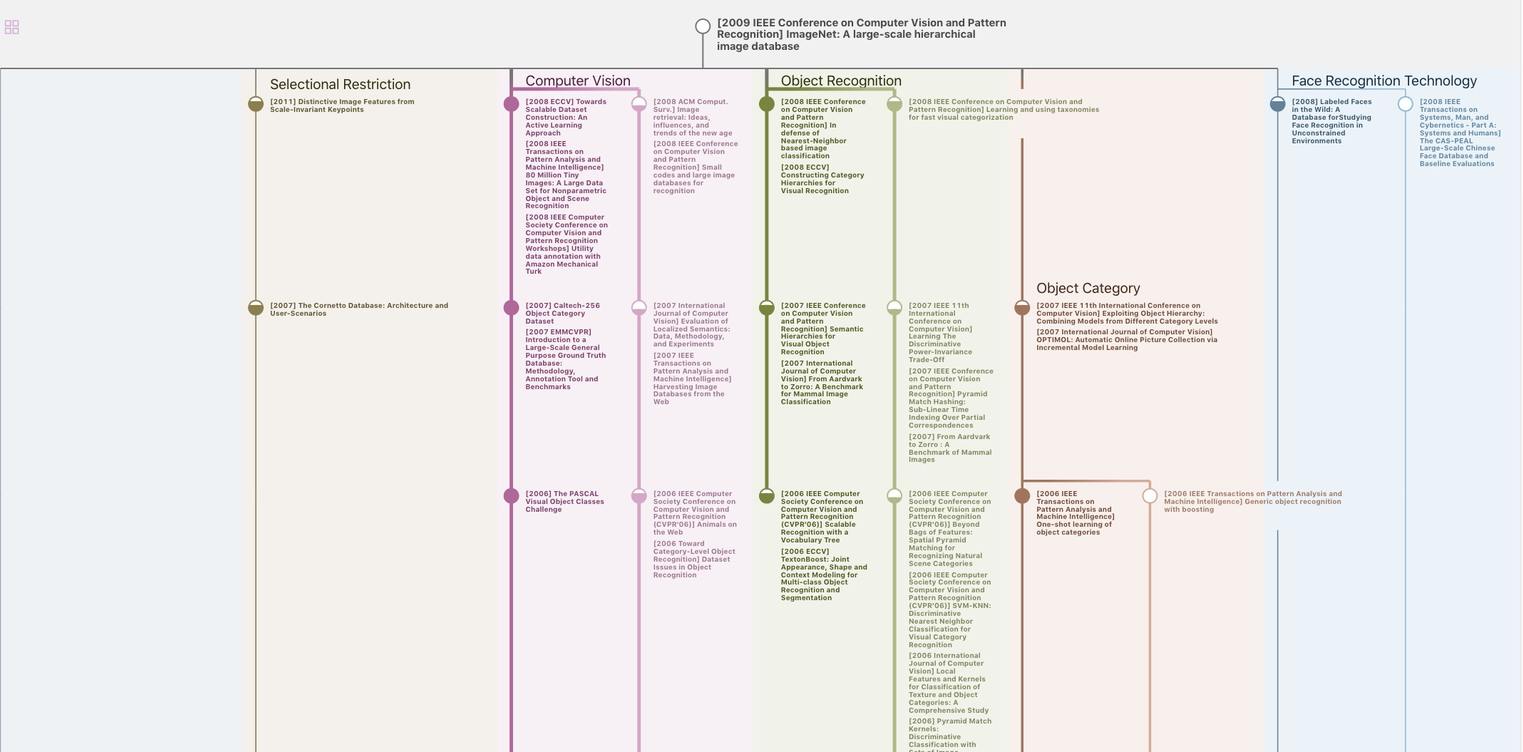
生成溯源树,研究论文发展脉络
Chat Paper
正在生成论文摘要