Predicting Polarization Beyond Semantics for Wearable Robotics
2018 IEEE-RAS 18th International Conference on Humanoid Robots (Humanoids)(2018)
摘要
Semantic perception is a key enabler in robotics, which supposes a very resourceful and efficient manner of applying vision information for upper-level navigation and manipulation tasks. Given the challenges on specular semantics such as water hazards, transparent glasses and metallic surfaces, polarization imaging has been explored to complement the RGB-based pixel-wise semantic segmentation because it reflects surface characteristics and provides additional attributes. However, polarimetric measurements generally entail prohibitively expensive cameras and highly accurate calibrations. Inspired by the representation power of Convolutional Neural Networks (CNNs), we propose to predict polarization information from monocular RGB images, precisely per-pixel polarization difference. The core of our approach is a cluster of efficient deep architectures building on factorized convolutions, hierarchical dilations and pyramid representations, aimed to produce both semantic and polarimetric estimations in real time. Comprehensive experiments demonstrate the qualified accuracy on a wearable exoskeleton humanoid robot.
更多查看译文
关键词
wearable robotics,semantic perception,vision information,upper-level navigation,specular semantics,water hazards,transparent glasses,metallic surfaces,polarization imaging,RGB-based pixel-wise semantic segmentation,surface characteristics,polarimetric measurements,highly accurate calibrations,polarization information,monocular RGB images,per-pixel polarization difference,efficient deep architectures,factorized convolutions,pyramid representations,semantic estimations,polarimetric estimations,wearable exoskeleton humanoid robot,convolutional neural networks
AI 理解论文
溯源树
样例
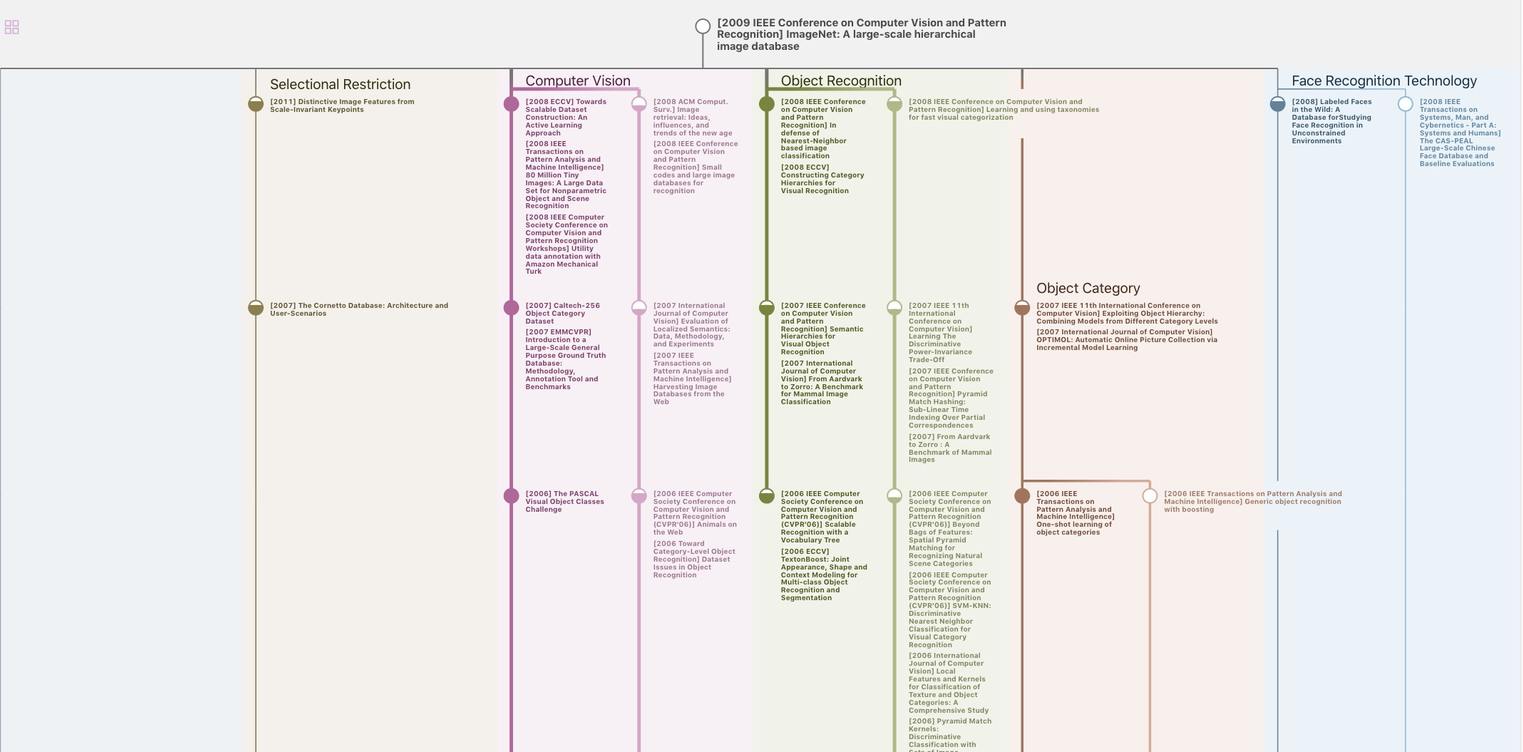
生成溯源树,研究论文发展脉络
Chat Paper
正在生成论文摘要