Situation-Based Interpretable Learning for Personality Prediction in Social Media
2018 IEEE INTERNATIONAL CONFERENCE ON BIG DATA (BIG DATA)(2018)
摘要
Predicting individuals personality traits with their social media profile has proved to be feasible, but researchers recently have run into bottlenecks on further improving the prediction accuracy. One major limitation is that existing studies failed to consider context information in predicting social media users' behaviors. In this paper, we adopted the DIAMONDS situation theory in psychology to capture the context information in Facebook posts. To solve this issue, we proposed a novel situation-based feature interaction learning model. In this study, we extracted situation features according to the DIAMONDS lexicon and computed the interaction values between these situation features and the commonly used n-gram features at the post level. Features at the post level were aggregated up to the user level using the averaging strategy. A group lasso penalty was employed to enforce strong heredity in the model, which addressed the overfitting challenge introduced by the interaction features. Empirical tests on a large-scale data set have demonstrated the effectiveness of the proposed method.
更多查看译文
关键词
personality, situation, psychology, social media, interaction learning
AI 理解论文
溯源树
样例
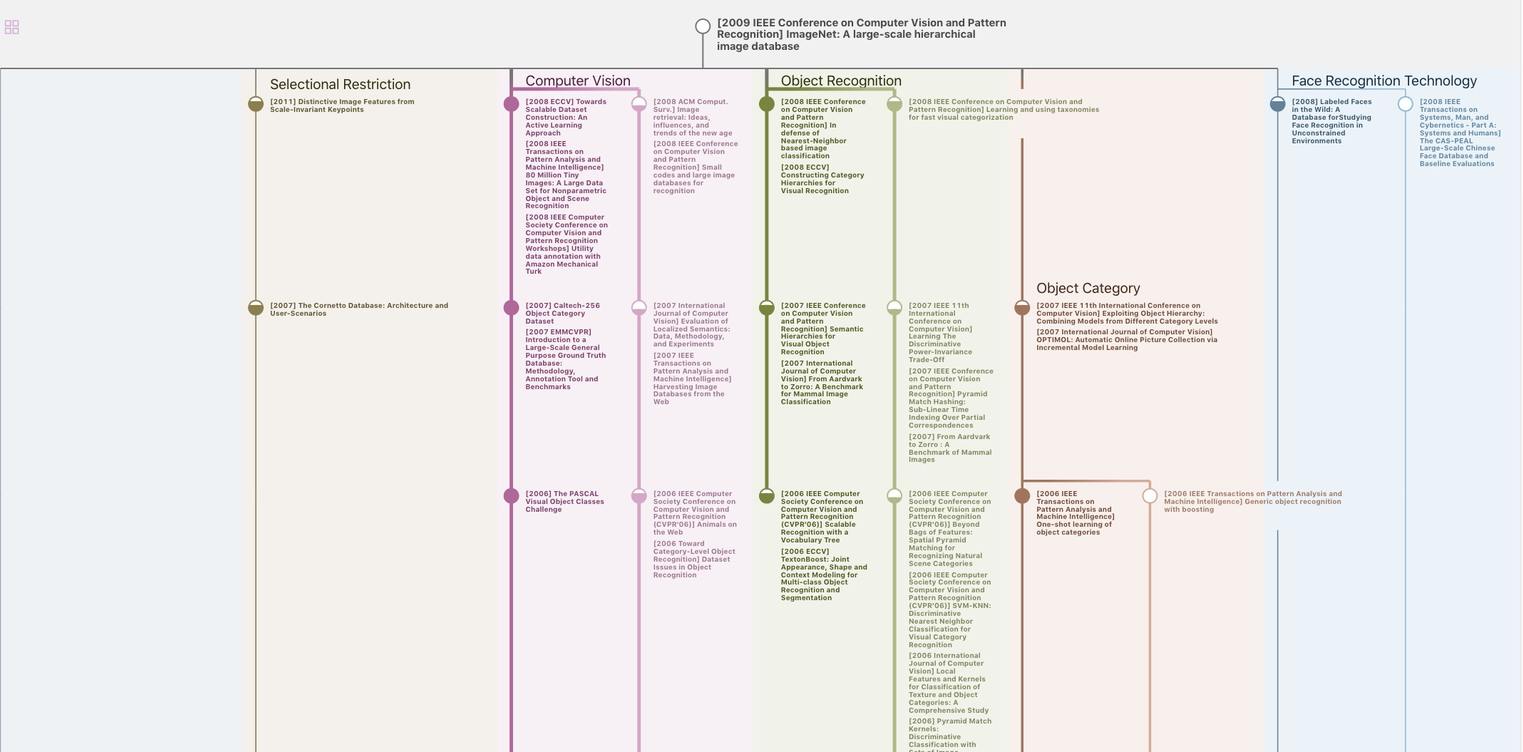
生成溯源树,研究论文发展脉络
Chat Paper
正在生成论文摘要