Harnessing the Nature of Spam in Scalable Online Social Spam Detection
2018 IEEE INTERNATIONAL CONFERENCE ON BIG DATA (BIG DATA)(2018)
摘要
Disinformation in social networks has been a worldwide problem. Social users are surrounded by a huge volume of malicious links, biased comments, fake reviews, or fraudulent advertisements, etc. Traditional spam detection approaches propose a variety of statistical feature-based models to filter out social spam from a historical dataset. However, they omit the real word situation of social data, that is, social spam is fast changing with new topics or events. Therefore, traditional approaches cannot effectively achieve online detection of the "drifting" social spam with a fixed statistic feature set. In this paper, we present Sifter, a system which can detect online social spam in a scalable manner without the labor-intensive feature engineering. The Sifter system is two-fold: (1) a decentralized DHT-based overlay deployment for harnessing the group characteristics of social spam activities within a specific topic/event; (2) a social spam processing with the support of Recurrent Neural Network (RNN) to get rid of the traditional manual feature engineering. Results show that Sifter achieves graceful spam detection performances with the minimal size of data and good balance in group management.
更多查看译文
关键词
online social networks, spam detection, RNN
AI 理解论文
溯源树
样例
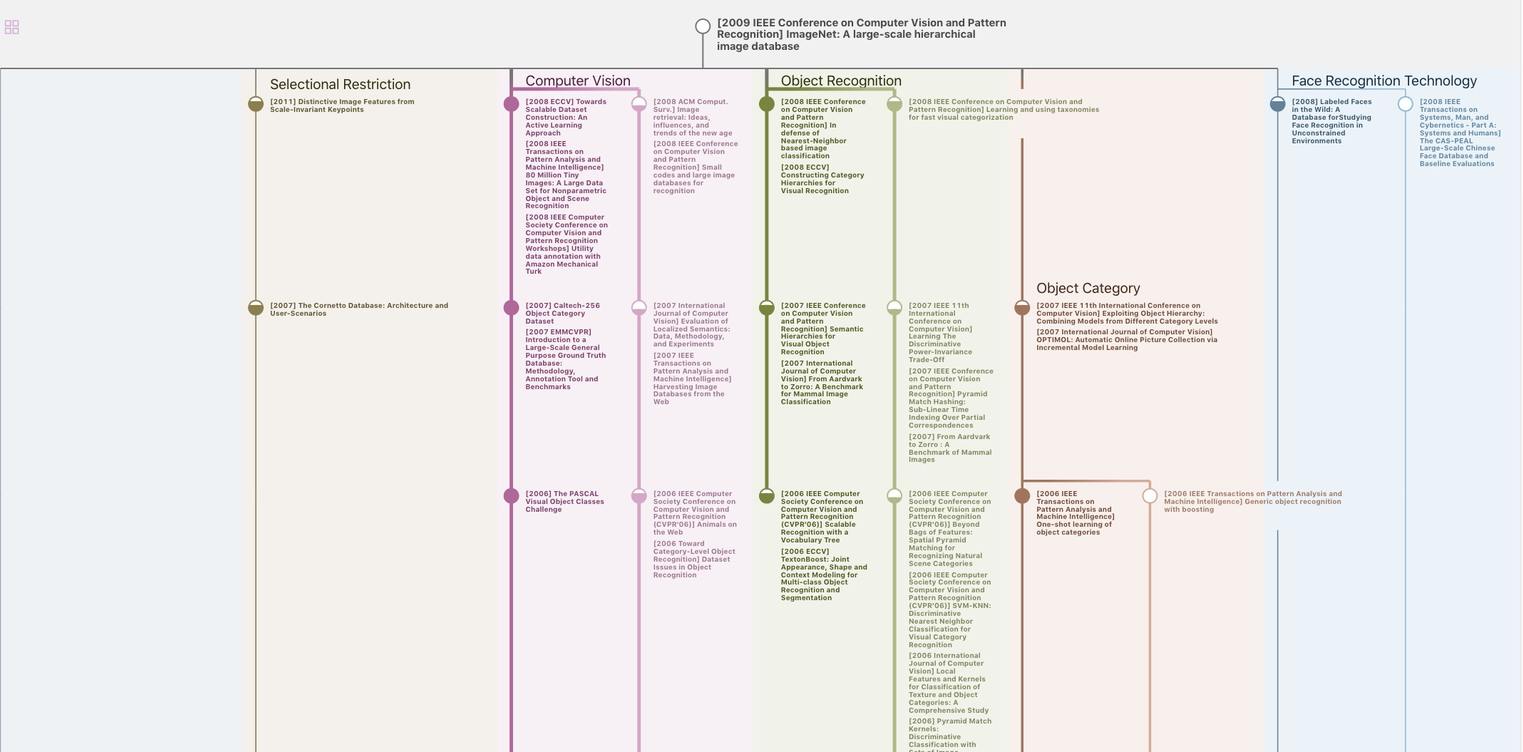
生成溯源树,研究论文发展脉络
Chat Paper
正在生成论文摘要