Learning Effective Embeddings for Machine Generated Emails with Applications to Email Category Prediction
2018 IEEE INTERNATIONAL CONFERENCE ON BIG DATA (BIG DATA)(2018)
摘要
Machine generated business-to-consumer (B2C) emails such as receipts, newsletters, and promotions constitute a large portion of users' inboxes today. These emails reflect the users' interests and often are sequentially correlated, e.g., users interested in relocating may receive a sequence of messages on housing, moving, job availability, etc. We aim to infer (and eventually serve) the users' future interests by predicting the categories of their future emails. There are many useful methods, such as recurrent neural networks, that can be applied for such predictions, but in all cases the key to better performance is an effective representation of emails and users. To this end, we propose a general framework for learning embeddings for emails and users, using as input only the sequence of B2C templates users receive and open. (A template is a B2C email stripped of all transient information related to specific users.) These learned embeddings allow us to identify both sequentially correlated emails and users with similar sequential interests. We can also use the learned embeddings either as input features or embedding initializers for email category prediction tasks. Extensive experiments with millions of fully anonymized B2C emails demonstrate that the learned embeddings can significantly improve the prediction accuracy for future email categories. We hope that this effective yet simple embedding learning framework will inspire new machine intelligence applications that will improve the users' email experience.
更多查看译文
关键词
email category prediction tasks,machine generated business-to-consumer,embedding learning,machine generated emails,users email experience,machine generated B2C
AI 理解论文
溯源树
样例
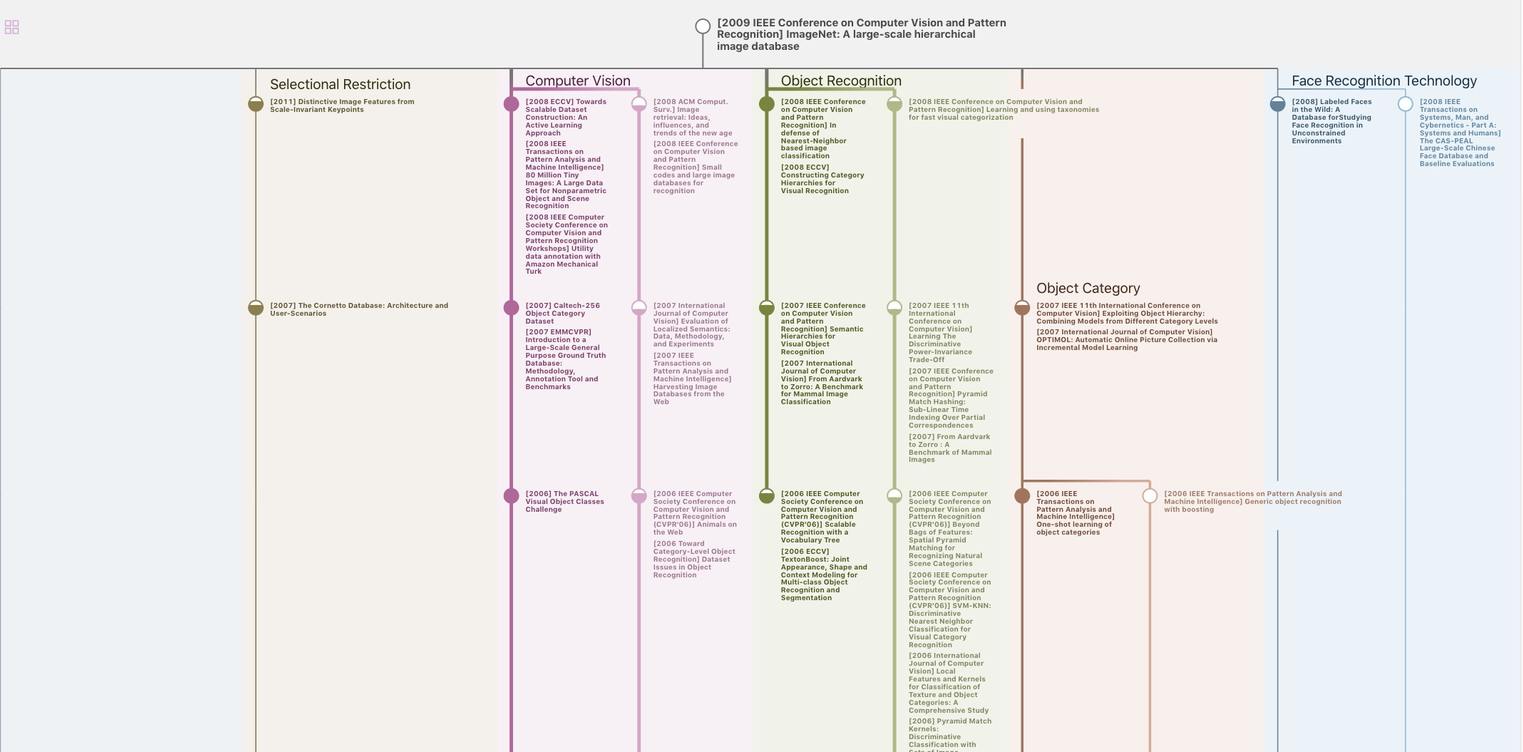
生成溯源树,研究论文发展脉络
Chat Paper
正在生成论文摘要