Conditioning Neural Networks: A Case Study Of Electricity Load Forecasting
2018 IEEE INTERNATIONAL CONFERENCE ON BIG DATA (BIG DATA)(2018)
摘要
Machine learning tasks typically involve minimizing a loss function that measures the distance of the model output and the ground-truth. In some applications, in addition to the usual loss function, the output must also satisfy certain requirements for further processing. We call such requirements model conditioning. We investigate cases where the conditioner is not differentiable or cannot be expressed in closed form and, hence, cannot be directly included in the loss function of the machine learning model. We propose to replace the conditioner with a learned dummy model which is applied on the output of the main model. The entire model, composed of the main and dummy models, is trained end-to-end. Throughout training, the dummy model learns to approximate the conditioner and, thus, forces the main model to generate outputs that satisfy the specified requirements. We demonstrate our approach on a use-case of demand charge-aware electricity load forecasting. We show that jointly minimizing the error in forecast load and its demand charge threshold results in significant improvement to existing load forecast methods.
更多查看译文
关键词
demand charge-aware electricity load forecasting,dummy models,main model,machine learning model,neural networks
AI 理解论文
溯源树
样例
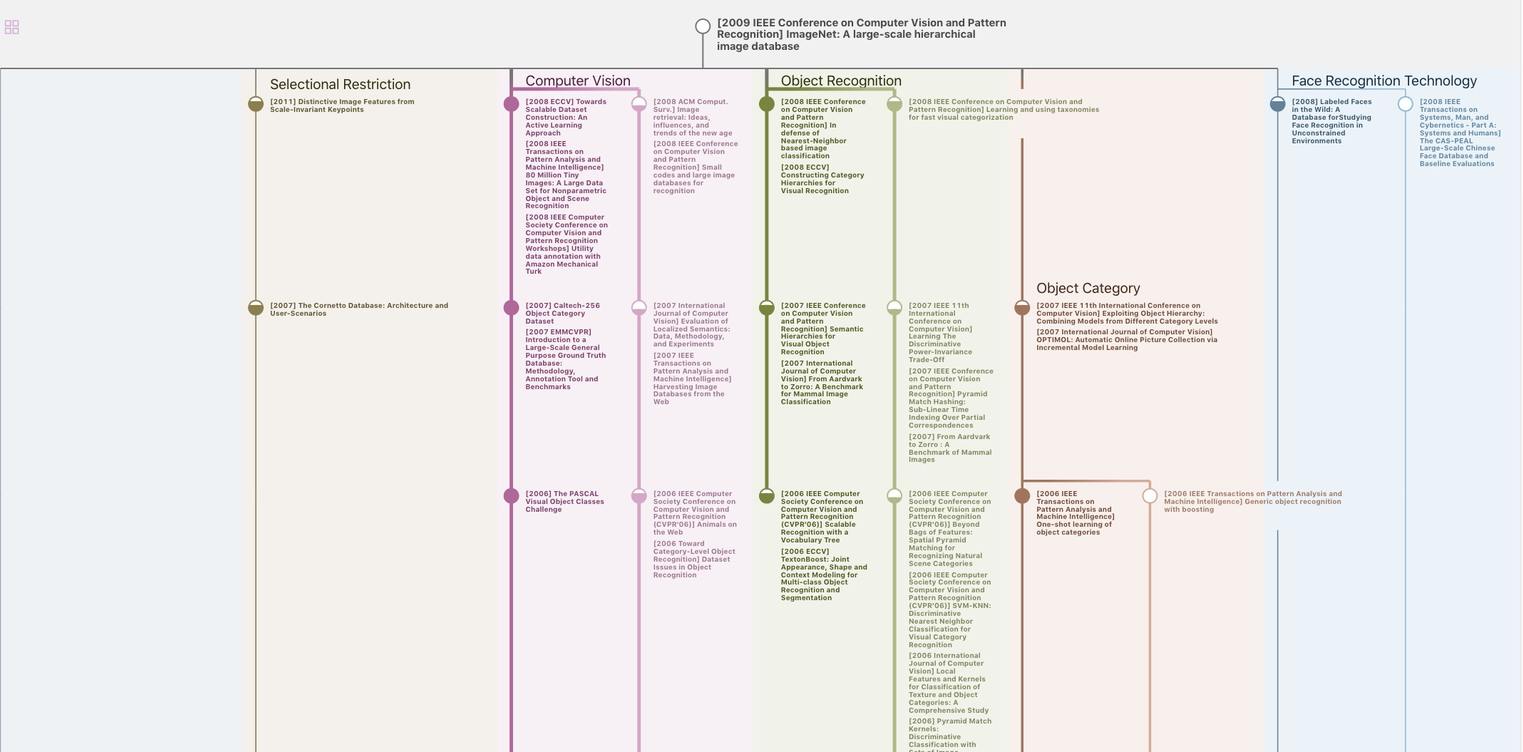
生成溯源树,研究论文发展脉络
Chat Paper
正在生成论文摘要