Gci: A Transfer Learning Approach For Detecting Cheats Of Computer Game
2018 IEEE INTERNATIONAL CONFERENCE ON BIG DATA (BIG DATA)(2018)
摘要
Cheating in massive multiple online games (MMOGs) adversely affect the game's popularity and reputation among its users. Therefore, game developers invest large amount of efforts to detect and prevent cheats that provide an unfair advantage to cheaters over other naive users during game play. Particularly, MMOG clients share data with the server during game play. Game developers leverage this data to detect cheating. However, detecting cheats is challenging mainly due to the limited client-side information, along with unknown and complex cheating techniques. In this paper, we aim to leverage machine learning based models to predict cheats over encrypted game traffic during game play. Concretely, network game traffic during game play from each player can be used to determine whether a cheat is employed. A major challenge in developing such a prediction model is the availability of sufficient training data, which is sparingly available in practice. Game traffic obtained from a few known players can be easily labeled. However, if such players are not a good representation of the population (i.e., other players), then a supervised model trained on labeled game traffic from these set of players may not generalize well for the population. Here, we propose a scalable transfer learning approach to overcome the constraints of limited labeled data. Our empirical evaluation on a popular MMOG demonstrates significant improvement in cheat prediction compared to other competing methods.
更多查看译文
关键词
Computer Games, Cheat Identification, Bias Correction, Relative Density Ratio
AI 理解论文
溯源树
样例
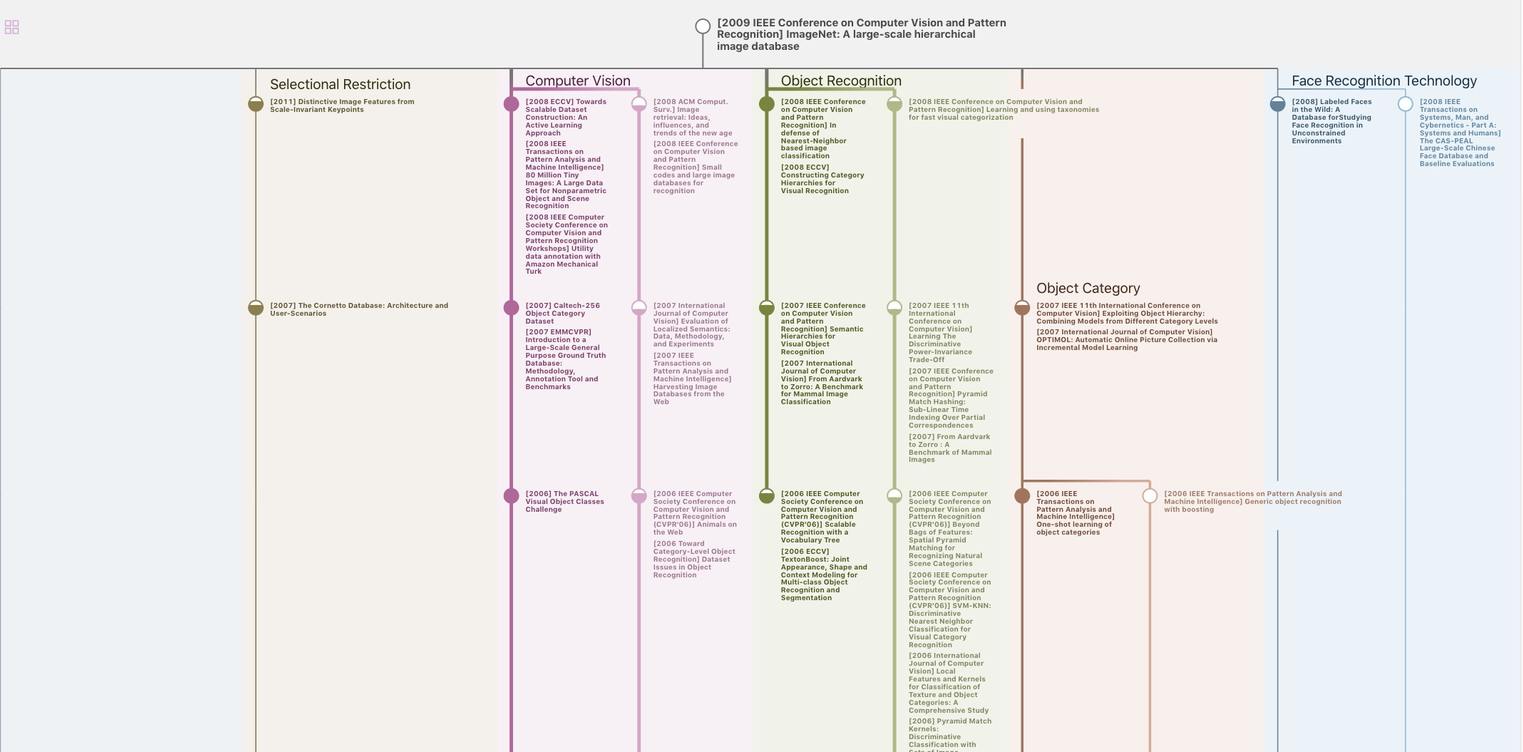
生成溯源树,研究论文发展脉络
Chat Paper
正在生成论文摘要