An In-Depth Comparison Of Group Betweenness Centrality Estimation Algorithms
2018 IEEE INTERNATIONAL CONFERENCE ON BIG DATA (BIG DATA)(2018)
摘要
One of the important indices defined for a set of vertices in a graph is group betweenness centrality. While in recent years a number of approximate algorithms have been proposed to estimate this index, there is no comprehensive and in-depth analysis and comparison of these algorithms in the literature. In this paper, we first present a generic algorithm that is used to express different approximate algorithms in terms of probability distributions. Using this generic algorithm, we show that interestingly existing methods have the same theoretical accuracy. Then, we present an extension of distance-based sampling to group betweenness centrality, which is based on a new notion of distance between a single vertex and a set of vertices. In the end, to empirically evaluate efficiency and accuracy of different algorithms, we perform experiments over several real-world networks. Our extensive experiments reveal that those approximate algorithms that are based on shortest path sampling are orders of magnitude faster than those algorithms that are based on pair sampling, while these two types of algorithms have almost comparable empirical accuracy.
更多查看译文
关键词
Social network analysis, group betweenness centrality, approximate algorithm, sampling, shortest path
AI 理解论文
溯源树
样例
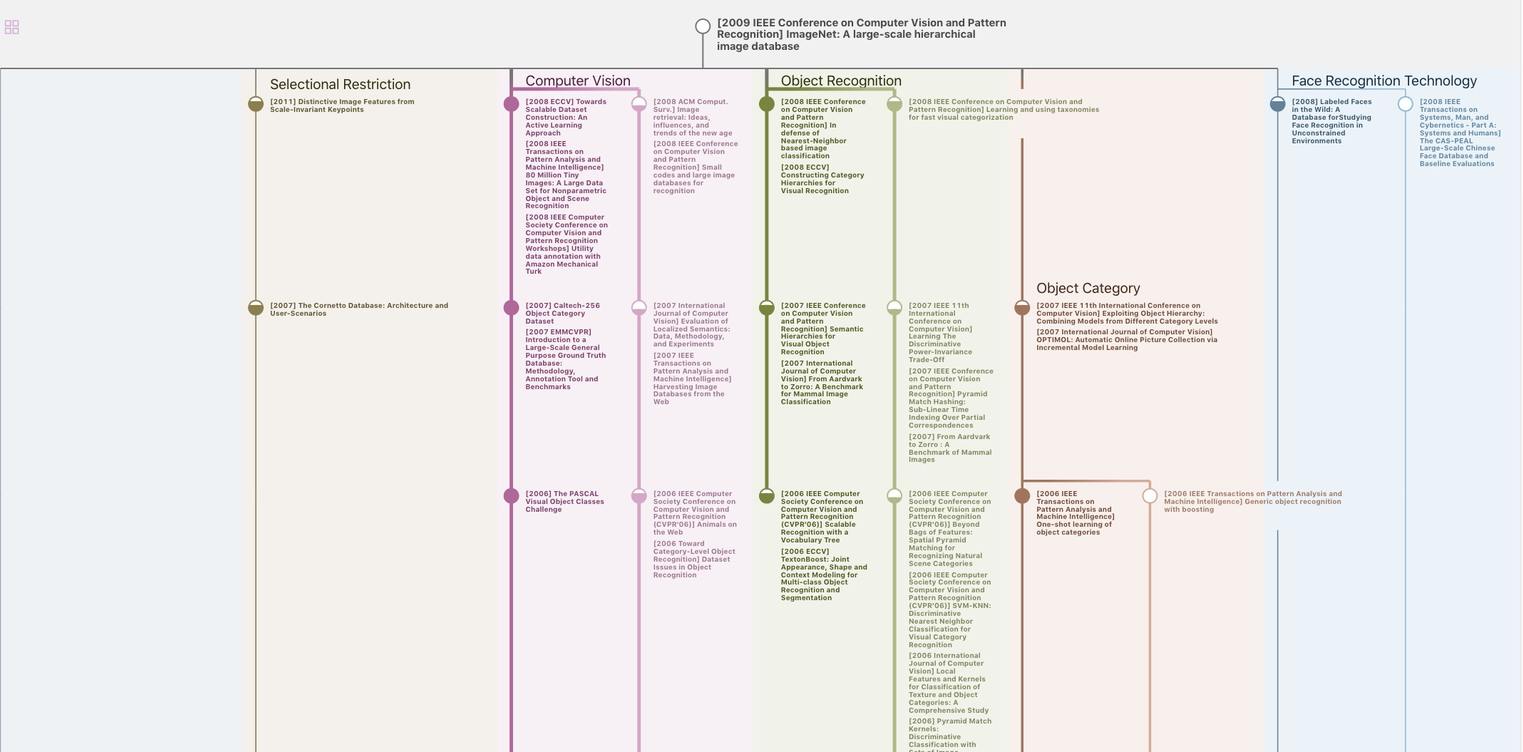
生成溯源树,研究论文发展脉络
Chat Paper
正在生成论文摘要