Privacy-Preserving User Profiling With Facebook Likes
2018 IEEE INTERNATIONAL CONFERENCE ON BIG DATA (BIG DATA)(2018)
摘要
The content generated by users on social media is rich in personal information that can be mined to construct accurate user profiles, and subsequently used for tailored advertising or other personalized services. Facebook has recently come under scrutiny after a third party gained access to the data of millions of users and mined it to construct psychographical profiles, which were allegedly used to influence voters in elections. As part of a possible solution to avoid data breaches while still being able to perform meaningful machine learning (ML) on social media data, we propose a privacy-preserving algorithm for k-nearest neighbor (kNN) [1] , one of the oldest ML methods, used traditionally in collaborative filtering recommender systems.
更多查看译文
关键词
privacy-preserving user profiling,personal information,accurate user profiles,tailored advertising,psychographical profiles,data breaches,social media data,privacy-preserving algorithm,machine learning,facebook likes,k-nearest neighbor,kNN,ML methods,collaborative filtering recommender systems
AI 理解论文
溯源树
样例
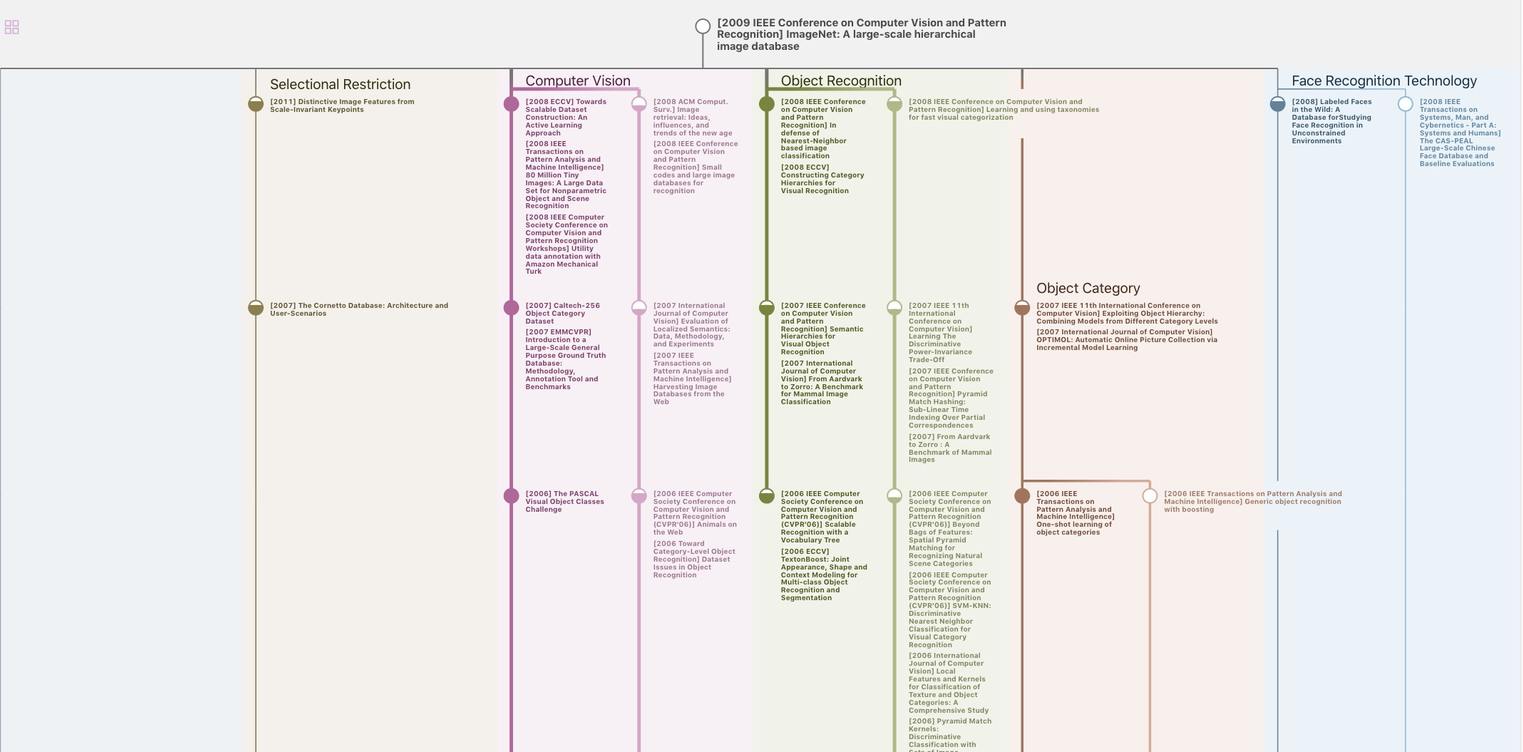
生成溯源树,研究论文发展脉络
Chat Paper
正在生成论文摘要