Cost-sensitive active learning with a label uniform distribution model.
International Journal of Approximate Reasoning(2019)
摘要
Active learning is a man-machine interaction scenario in which the machine acquires information actively from the expert. Cost-sensitive active learning balances the misclassification cost with the teacher cost paid for label queries. Inspired by granular computing (GrC) and three-way decision (3WD), this paper presents a new algorithm called cost-sensitive active learning through density clustering under a label uniform distribution model (CADU). CADU iteratively divides the universe, queries labels, and classifies instances until each label is queried or predicted. The density clustering technique is used to divide the universe into blocks. A label uniform distribution model is built to calculate the expected label distribution of each block. According to the teacher and misclassification cost settings, an optimization function is designed to determine the number of labels to be queried. Comparison study with 10 state-of-the-art algorithms are undertaken on 12 public datasets. Results show that CADU outperforms others in terms of average cost.
更多查看译文
关键词
Active learning,Density clustering,Granular computing,Label uniform distribution,Three-way decision
AI 理解论文
溯源树
样例
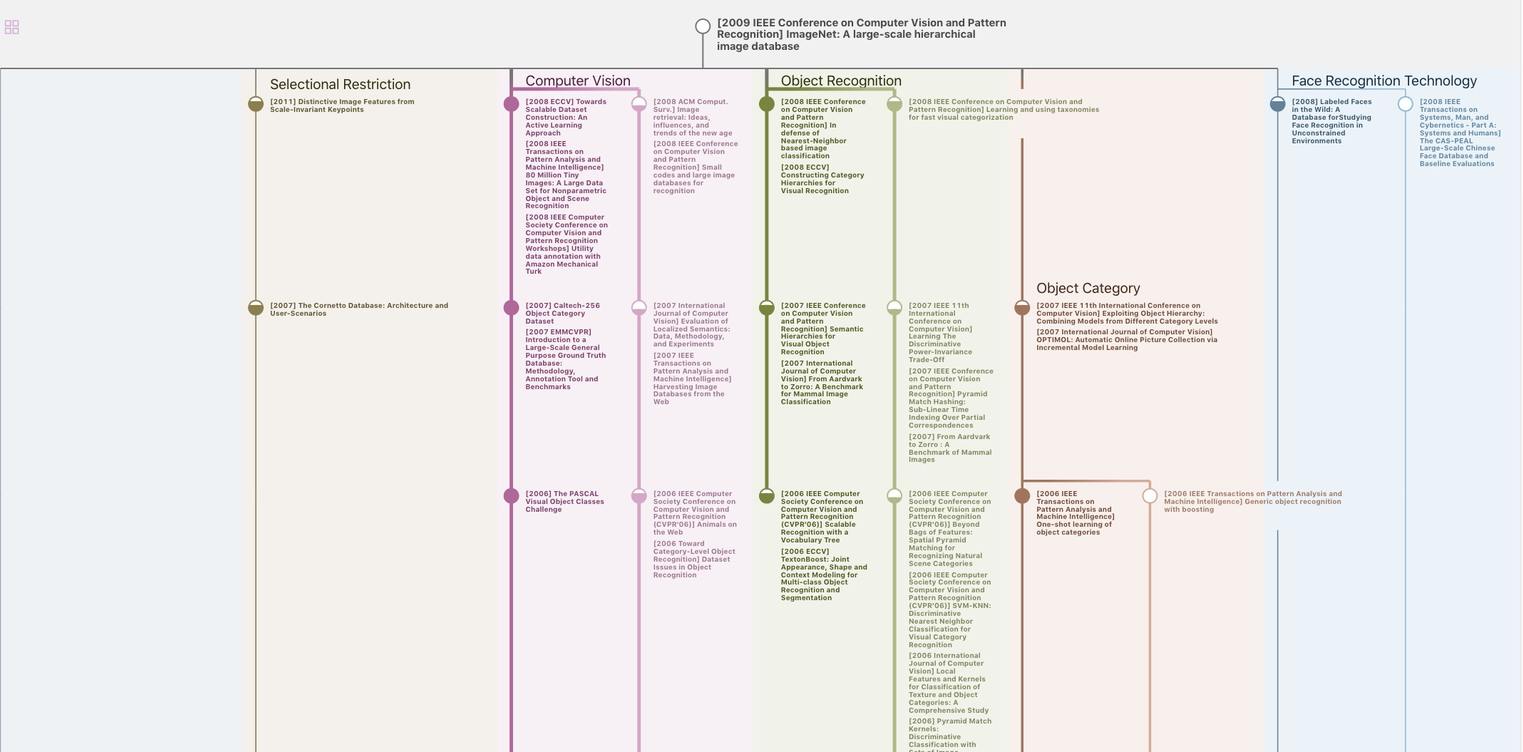
生成溯源树,研究论文发展脉络
Chat Paper
正在生成论文摘要