Multiorgan segmentation using distance-aware adversarial networks.
JOURNAL OF MEDICAL IMAGING(2019)
摘要
Segmentation of organs at risk (OAR) in computed tomography (CT) is of vital importance in radiotherapy treatment. This task is time consuming and for some organs, it is very challenging due to low-intensity contrast in CT. We propose a framework to perform the automatic segmentation of multiple OAR: esophagus, heart, trachea, and aorta. Different from previous works using deep learning techniques, we make use of global localization information, based on an original distance map that yields not only the localization of each organ, but also the spatial relationship between them. Instead of segmenting directly the organs, we first generate the localization map by minimizing a reconstruction error within an adversarial framework. This map that includes localization information of all organs is then used to guide the segmentation task in a fully convolutional setting. Experimental results show encouraging performance on CT scans of 60 patients totaling 11,084 slices in comparison with other state-of-the-art methods. (C) 2019 Society of Photo-Optical Instrumentation Engineers (SPIE)
更多查看译文
关键词
segmentation,deep learning,multiorgan,distance map,generative adversarial networks,convolutional neural networks,medical images
AI 理解论文
溯源树
样例
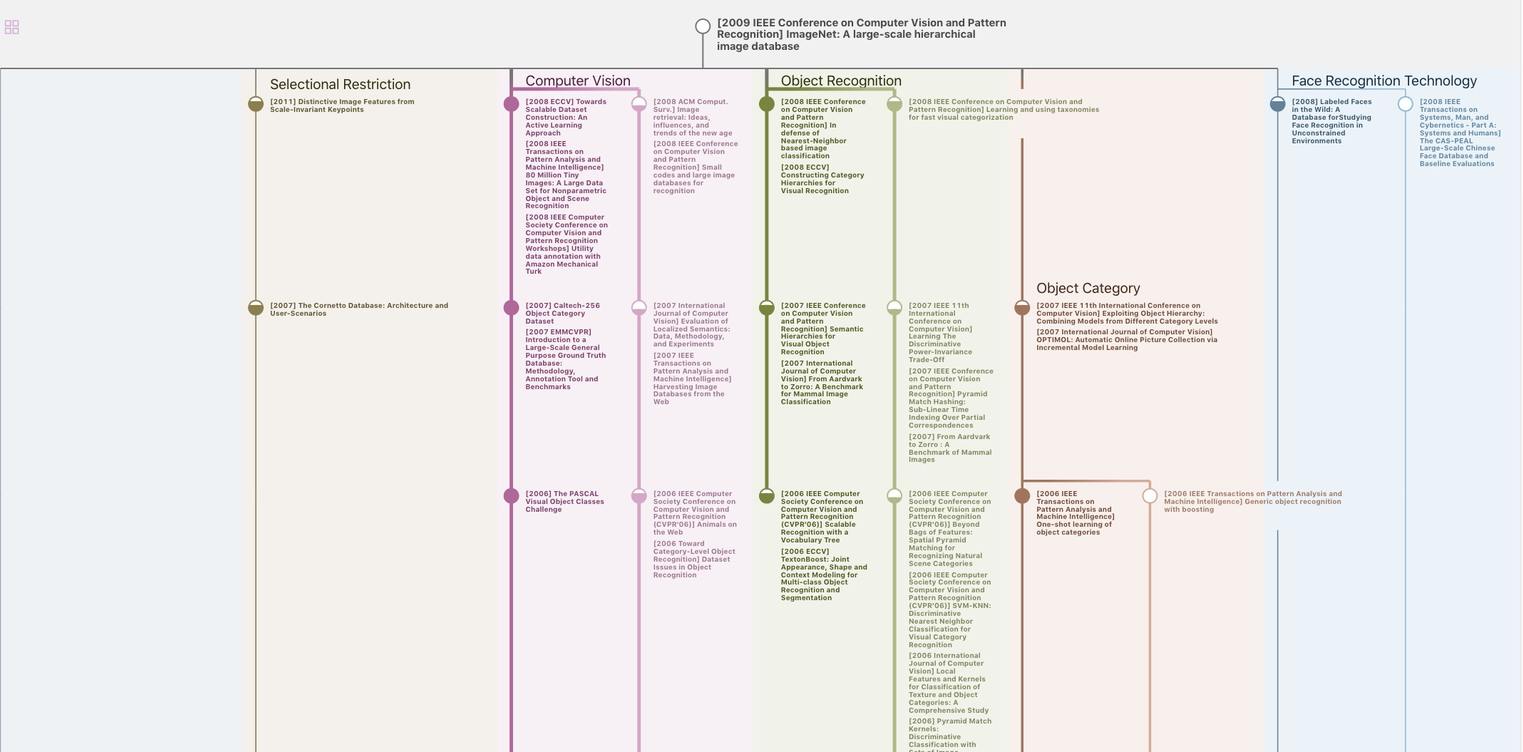
生成溯源树,研究论文发展脉络
Chat Paper
正在生成论文摘要